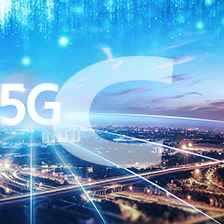
自然语言处理
文章平均质量分 86
NLP
ShadyPi
写写博客当笔记
展开
-
[Microsoft] A Comparative Study of DSL Code Generation
Natural Language to Domain Specific Language原创 2025-04-08 04:57:44 · 688 阅读 · 0 评论 -
[幻方 DeepSeek-R1] DeepSeek-R1: Incentivizing Reasoning Capability in LLMs via Reinforcement Learning
DeepSeek-R1原创 2025-01-30 05:08:40 · 896 阅读 · 0 评论 -
[GRPO] DeepSeekMath: Pushing the Limits of Mathematical Reasoning in Open Language Models
DeepSeekMath: GRPO原创 2025-01-28 15:20:43 · 925 阅读 · 1 评论 -
Proximal Policy Optimization (PPO) in LLM Training
PPO in LLM Training原创 2025-01-28 15:16:22 · 1021 阅读 · 0 评论 -
[DeepMind AlphaCode] Competition-Level Code Generation with AlphaCode
DeepMind AlphaCode原创 2025-01-22 14:23:44 · 591 阅读 · 0 评论 -
[OpenAI Codex] Evaluating Large Language Models Trained on Code
Codex from OpenAI原创 2025-01-21 12:17:38 · 1072 阅读 · 0 评论 -
NLP interview sheet
NLP interview questions原创 2025-01-01 12:27:32 · 1051 阅读 · 0 评论 -
Implementation of Multi-Head Self-Attention and Transformer Block
【Code】Implementation of Multi-Head Self-Attention and Transformer Block。原创 2024-12-05 15:22:50 · 614 阅读 · 0 评论 -
[Megagon Labs] Annotating Columns with Pre-trained Language Models
使用预训练语言模型标注表格数据类型以及数据关系原创 2024-08-13 17:21:04 · 675 阅读 · 0 评论 -
多模态小记:CLIP、BLIP与BLIP2
CLIP、BLIP以及BLIP2原创 2024-04-08 09:55:29 · 3796 阅读 · 0 评论 -
Interpretable Multimodal Misinformation Detection with Logic Reasoning
核心:多模态、可解释。原创 2023-12-05 10:04:37 · 1311 阅读 · 1 评论 -
Polish Questions to Catch the Inclination of Large Language Model: A Technical Report
Polish Questions to Catch the Inclination of Large Language Model: A Technical Report。原创 2023-10-16 22:36:23 · 244 阅读 · 0 评论 -
[Google DeepMind] LARGE LANGUAGE MODELS AS OPTIMIZERS
总体架构Optimization by PROmpting (OPRO):最开始输入meta-prompt,这个初始的meta-prompt基本上只是对优化任务进行了描述(也会有few-shot example)。输入后LLM便会生成一个solution,这个solution由objective function评估并打分。(solution, score)组合成一对添加到meta-prompt中,如此完成一个循环。多次循环后取分数最高的solution作为优化结果。原创 2023-09-13 19:58:47 · 1589 阅读 · 0 评论 -
Self-Polish: Enhance Reasoning in Large Language Models via Problem Refinement
核心思想是通过instruction让LLM来优化问题本身,从而达到更好的效果,且这种针对问题的优化可以跟其他的prompt技术,如CoT或者Least-to-Most相结合。原创 2023-09-13 14:14:24 · 477 阅读 · 0 评论 -
ACL2023 Prompt 相关文章速通 Part 1
ACL2023 prompt相关文章速览原创 2023-08-25 12:13:31 · 1744 阅读 · 0 评论 -
[ACL2023] Symbolic Chain-of-Thought Distillation: Small Models Can Also “Think” Step-by-Step
虽然CoT作为一种prompt形式在提高LLM的表现上有很好的效果,但此前有研究表明CoT并不能在小规模的LM(参数原创 2023-08-25 12:11:03 · 449 阅读 · 1 评论 -
[ACL2023] Exploring Lottery Prompts for Pre-trained Language Models
基于此,作者提出了一种集成prompt方法,在少量的训练集上选出优秀的prompt以后,根据他们的表现赋予不同的权重,表现越好的prompt权重越高,之后将这些prompt的分类预测加权在一起,得到最后的分类。而模型的能力也对搜索次数有影响,越大的模型需要的搜索次数越少。除了多个prompt对一个input,那自然也有一个prompt对多个input,作者统计了prompt在整个数据集上的表现,除了有66个类的最难的Few-NERD,其他数据集都能找到一个表现不错的prompt。原创 2023-08-24 19:39:36 · 809 阅读 · 0 评论 -
The CoT Collection: Improving Zero-shot and Few-shot Learning of Language Models via Chain-of-Though
主要的收集方法依然是基于LLM生成,首先人工手写并筛选出精品CoT样例,之后以这些样例为demo,让LLM输出某个问题的推理过程(rationale),从而获得大量的带rationale的问题作为数据集。最后,作者对生成的这些rationale进行了评估,质量评估使用了ROSCOE的13个指标,又评估了rationale的多样性,显示他们的CoT Collection是更多样化的(动词更多样)。第四步,过滤生成的rationale,作者删掉了rationale中没出现答案的、太长的以及相同的。原创 2023-08-24 15:37:27 · 429 阅读 · 0 评论 -
Automatic Prompt Augmentation and Selection with Chain-of-Thought from Labeled Data
港科的工作,主要着眼于CoT样例的生成和选取,数据增强的部分就是用已有的CoT样例做few-shot推理,让LLM生成新的CoT样例(或者直接用Zero-shot-CoT),将生成正确的样例加入到备选池子里。至于如何选择,则是将抽取样例的过程看成从一个随机分布里采样的过程。用一些参数构建一个随机分布,该分布的采样值对应不同的CoT样例。为了学习这个分布,作者采用了强化学习的方法。实验显示在各数据集上达到了SOTA。原创 2023-08-22 16:33:30 · 278 阅读 · 0 评论 -
Toward Human Readable Prompt Tuning: Kubrick’s The Shining is a good movie, and a good prompt too?
基于soft prompt,但生成较可读的prompt,探寻优质prompt的特征原创 2023-08-07 12:19:06 · 156 阅读 · 0 评论 -
Demystifying Prompts in Language Models via Perplexity Estimation
低困惑度的prompt更能提升LLM的性能原创 2023-08-04 13:04:04 · 1250 阅读 · 0 评论 -
INSTRUCTZERO: EFFICIENT INSTRUCTION OPTIMIZATION FOR BLACK-BOX LARGE LANGUAGE MODELS
用soft prompt调教Vicuna,生成更好的instruction提高chatGPT的性能原创 2023-07-31 17:29:46 · 308 阅读 · 0 评论 -
[ACL2023] Prompter: Zero-shot Adaptive Prefixes for Dialogue State Tracking Domain Adaptation
Soft prompt 助力 Dialogue State Tracking 任务原创 2023-07-28 20:26:30 · 299 阅读 · 0 评论 -
[ICML2023] PromptBoosting: Black-Box Text Classification with Ten Forward Passes
弱Prompt在分类问题上的集成原创 2023-07-28 15:40:50 · 195 阅读 · 0 评论 -
[ICML2023] Synthetic Prompting: Generating Chain-of-Thought Demonstrations for Large Language Models
NLP推理任务的Program-aimed样例生成原创 2023-07-28 15:39:52 · 360 阅读 · 0 评论 -
Prompt Engineering论文梳理(主要为2022年)
主要是2022年Prompt Engineering相关工作的梳理原创 2023-07-27 11:36:56 · 1723 阅读 · 0 评论 -
Automatic Prompt Optimization with “Gradient Descent” and Beam Search
整篇文章比较精髓的思想在于yyppggpggp。原创 2023-06-01 20:57:55 · 2040 阅读 · 3 评论