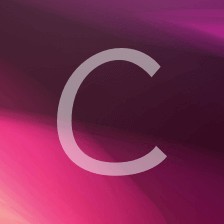
paper
seamanj
这个作者很懒,什么都没留下…
展开
-
End-to-end Recovery of Human Shape and Pose
公式(1)LreprojL_{reproj}Lreprojjoint投影到2D的误差L3DL_{3D}L3D joint和smpl的regressionLadvL_{adv}Ladv adversary train原创 2019-11-06 03:29:32 · 578 阅读 · 3 评论 -
DaNet: Decompose-and-aggregate Network for 3D Human Shape and Pose Estimation
UVI 当中间变量global UVI regresses camera and shapepartial UVI regresses rotationpredict rotation时, 切换到postion然后用LSTM , 最后再refine rotation.原创 2019-11-06 02:19:55 · 457 阅读 · 0 评论 -
hand keypoint detection in single images using multiview bootstrapping
这篇文章主要detect手关节的位置对于labelled 的数据直接用公式(3)然后对于unlabelled的数据用algorithm 1去生成labelled数据 用于下一次的训练section 5, 主要说明 detector和camera view之间的需求关系给定detector可以算出PCK, 对于不同的TP或者 FP的要求根据图6可以选择不同的V, 即view的个数...原创 2019-11-04 21:29:16 · 1008 阅读 · 0 评论 -
convolutional pose machines
该篇文章主要用来估计Joint的位置贡献在于用了ψt>1\psi_{t>1}ψt>1 capture spatial context across all stages值得注意的句子the magnitude of back-propagated gradients decreases in strength with the number of intermediat...原创 2019-11-04 21:15:53 · 303 阅读 · 0 评论 -
Learning to Reconstruct 3D Human Pose and Shape via Model-fitting in the Loop
这篇文章把optimization and regression做了一个结合, 让他们相互支持和提高公式(1), 回归SMPL模型的参数公式(2), 即simipify 里面的优化过程公式(3),(4), 用优化后的结果回归新的SMPL模型参数...原创 2019-10-14 19:43:47 · 600 阅读 · 0 评论 -
keep it SMPL: Automatic estimation of 3d human pose and shape from a single image
公式(2), 即SMPL 3D模型上面投影后的joint和2d image上 detected joint points之间的差异公式(3), 惩罚elbows and knees 向外弯, 即θ\thetaθ大于0公式(4), pose prior公式(6), 相交关节的penalty, 如果关节i,j相交 , 则他们所在的球的球心之间的距离由公式(6)来约束, 这里我不是太明白???...原创 2019-10-14 18:51:06 · 722 阅读 · 0 评论 -
realtime multi-person 2D pose estimation using part affinity fields
2.Method JJJ个joint, 对应JJJ张confidence mapSJS_JSJ, CCC个limb, 对应CCC张向量图(PAF)LCL_CLCfigure3 以及公式(1)(2), F是feature map, StS^tSt第t阶段的confidence map, LtL^tLt第t阶段的PAF公式(7), 对于多人的confidence map, 在某一点...原创 2019-10-13 17:48:44 · 123 阅读 · 0 评论 -
Monocular Total capture: posing face, body, and hands in the wild
(2)公式算POF, 即关节到关节的单位向量, 跟PAF中2维向量不同, 这里是3维向量公式(4)中, jmBj_m^BjmB是2d image detected keypoints, Π(J~mB(θ,ϕ,t))\mathbf \Pi(\widetilde{\mathbf J}^B_m(\theta, \phi, t))Π(JmB(θ,ϕ,t))是根据参数建立的3D模型中keypoint...原创 2019-10-11 22:57:01 · 626 阅读 · 0 评论 -
Energetically Consistent Invertible Elasticity
文章来源: Energetically Consistent Invertible Elasticity2 Isotropic hyperelasticity 证明 P(F)=UP^(σ)VTP(F)=U\hat{P}(\sigma)V^{T}其实有更简单的证明方法:1.Invertible Finite Elements For Robust Simulation of Large De原创 2015-12-08 03:18:28 · 731 阅读 · 0 评论 -
Projective Dynamics: Fusing Constraint Projections for Fast Simulation
3.1 Implicit Euler Solverqn+1=qn+hvn+1(1)q_{n+1}=q_{n}+hv_{n+1}\hspace{200px}(1) vn+1=vn+hM−1(fint(qn+1+fext)(2)v_{n+1} =v_{n}+hM^{-1}(f_{int}(q_{n+1}+f_{ext})\hspace{55px}(2) 将(2)代入(1)得 qn+1=qn+hvn原创 2015-11-21 08:32:57 · 931 阅读 · 0 评论 -
Robust Quasistatic Finite Elements and Flesh Simulation
文章来源: Robust Quasistatic Finite Elements and Flesh Simulation3.Quasistatic Formulation{ 公式xt→=v⃗ \vec{x_t}=\vec{v}以及vt→=M−1f⃗ (t,x⃗ ,t⃗ )\vec{v_t}=M^{-1}\vec{f}(t,\vec{x},\vec{t})中下标tt表示对tt求导 }4.Str原创 2015-12-25 00:42:03 · 941 阅读 · 0 评论 -
how to calculate the QR decomposition of a matrix
In many papers(such as [1], [2]), we need to solve the polar decomposition of a matrix which decompose a matrix A into a rotation matrix R and a symmetric matrix S, s.t. A = RSHere, we will be u原创 2016-02-05 05:18:13 · 1037 阅读 · 0 评论 -
mean value coordinates
paper source:http://128.148.32.110/courses/cs224/papers/mean_value.pdfexpanding process2.4 -> 2.3原创 2016-03-18 06:45:51 · 2005 阅读 · 1 评论 -
As-Rigid-As-Possible Surface Modeling
paper source2.1if M is a psd(positive semi definite) matrix, then for any orthogonal R, Tr(M)≥Tr(RM)Tr(M) \ge Tr(RM){ note: In this monograph positive(semi) define matrices are necessarily symmetric.原创 2016-01-28 00:21:12 · 3402 阅读 · 0 评论 -
Mixed Finite Elements for Variational Surface Modeling
paper source { formula 5 LB=12<v,v>Ω0+<λ,Δu−v>Ω0=12<v,v>Ω0−<λ,v>Ω0+<λ,Δu>Ω0L_B=\frac{1}{2}<v,v>_{\Omega_0}+<\lambda,\Delta u-v>_{\Omega_0}=\frac{1}{2}<v,v>_{\Omega_0}-<\lambda,v>_{\Omega_0}+<\lambda,原创 2016-06-20 23:20:21 · 683 阅读 · 0 评论 -
Bounded Biharmonic Weigths for Real-Time Deformation
3.1 Formulation { First of all, let me explain the physical meaning of the objective function. The function tries to make the weight of each anchor point on each point have least changed. The ideal原创 2016-06-03 03:31:23 · 1739 阅读 · 2 评论 -
position based dynamics
pbd-position based dynamics原创 2014-08-14 09:08:33 · 6783 阅读 · 27 评论 -
As-Conformal-As-Possible Surface Registration
{3.2. Conformal stiffness energyLConformal(T)=∥T11−T22∥2+∥T22−T33∥2+∥T33−T11∥2+∥T12+T21∥2+∥T23+T32∥2+∥T31+T13∥2LConformal(T) =\lVert T_{11} - T_{22}\rVert^2+\lVert T_{22} - T_{33}\rVert^2+\lVert T_{33}原创 2016-07-07 00:43:09 · 1080 阅读 · 1 评论 -
Alec jacobson thesis analysis
chapter 2Q1: what is an Euler-Lagrange equation? Here why the Euler-Lagrange equation is the Laplace equation?A1: refer to http://blog.youkuaiyun.com/seamanj/article/details/50898781Q2: How ca原创 2016-03-15 19:36:04 · 648 阅读 · 0 评论 -
Factorized Graph Matching
{ 推导:7式 (3) vec(X)TKvec(X)vec(X)^TKvec(X) (6) K=diag(vec(Kp))+(G2⊗G1)diag(vec(Kq))(H2⊗H1)TK = diag(vec(K_p))+(G_2\otimes G_1)diag(vec(K_q))(H_2\otimes H_1)^T 将(6)式代入(3)式得到: \begin{align} &vec(X)^原创 2016-08-26 20:38:28 · 2340 阅读 · 0 评论 -
non-rigid shape registration using similarity-invariant differential coordinates
这篇其实是as rigid as possible的变种, 只不过把R换成了sR(2)->(3) 同时除个标量说得过去, 因为这里取的是向量变后的方向需要一致, 同时除个标量不影响方向(5)->(6) 公式(6)原文写错了应该为∑j∈Ni4wij(e′ij−12(siRi+sjRj)eij)\sum_{j \in N_i}4w_{ij}(e'_{ij}-\frac{1}{2}(s_iR_i+s_原创 2016-11-28 19:48:38 · 913 阅读 · 0 评论 -
Non-Rigid Registration Under Isometric Deformations
2. OverviewCorrespondence Computation.这部分讲matching过程不仅考虑最近点, 而且还要考虑feature最相似的点3. Downsampled RepresentationFeatures算principle curvatures作为它的feature discriptor5.1 算cluster, 注意s原创 2016-11-28 22:47:58 · 998 阅读 · 0 评论 -
Scale-Dependent 3D Geometric Features
这篇文章主要讲如何提取3D模型中的corner和edge. 但是是scale-dependent的我理解的scale就是文中平滑的时候影响的范围大小原创 2016-11-30 23:01:54 · 466 阅读 · 0 评论 -
Hierarchical deformation of Locally Rigid Meshes
这篇文章的主要思想是, 先通过 edge collapse 将 mesh 简化, 然后呢通过变形简化的模型 , 重建出复杂的模型, 行为有点类似于embedding deformation那篇2.1 找每个点的EsE_s, 用来决定哪条边被退化, 我估计边退化这里取的是中点2.2 EcE_c呢是指密集点ckc_k在constrain的约束下(实际上就是handle的控制下)移动到dkd_k后,它们原创 2016-12-05 20:45:17 · 424 阅读 · 0 评论 -
Meshless Deformations Based on Shape Matching
论文来源公式(7)的推导:原创 2016-01-16 06:20:40 · 1040 阅读 · 1 评论 -
as-conformal-as-possible discrete volumetric mapping
cauchy-riemann equation 3.1中的cauchy-riemann equation中的fxf_x和fyf_y就相当于这里的uu和vv 这个方程呢是相对于每个点来讲的, f的三个分量可以看作在每个点处的偏移量3.2 公式(1)是tetrahedron的插值公式对公式(1)求导,得到JJ, 结果3.1中的D\mathscr D从而可以推出公式(2)中的DD公式(3)的推导:从上原创 2016-12-06 22:57:27 · 478 阅读 · 0 评论 -
Projective Dynamics: Fusing Constraint Projections for Fast Simulation
1.(3)->(4)qn+1−qn−hvn−h2Mfext=h2Mfint(qn+1)q_{n+1}- q_n-hv_n-\frac{h^2}{M}f_{ext}= \frac{h^2}{M}f_{int}(q_{n+1}) 1h2M(qn+1−sn)=fint(qn+1)\frac{1}{h^2}M(q_{n+1}- s_n)= f_{int}(q_{n+1})右边项左移然后对qn+1q_{n+原创 2016-11-23 18:55:31 · 1383 阅读 · 3 评论 -
Deformation Transfer for Triangle Meshes
1.where S refers to the set of triangle indices for the source mesh.这里S是指source三角形的个数2.M集合里面的pair个数是指, source里的s1三角形的形变应与target里t1三角形的形变接近3.对于公式7, |T|代表target里面三角形的个数. 公式7的几何意义, source中的原创 2016-11-28 05:48:02 · 3669 阅读 · 5 评论 -
Optimal Step Nonrigid ICP Algorithms for surface registration
这篇paper的约束有三个:距离, stiffness, landmark原创 2016-11-28 06:30:33 · 1843 阅读 · 0 评论 -
Stable Region Correspondences Between Non-Isometric Shapes
to be read...原创 2017-01-11 00:23:45 · 604 阅读 · 0 评论 -
Deformable 3D shape registration based on local similarity transforms
本质上是shape matching的as similar as possible版本它的主要贡献在于, 找到一种方法使displacement field尽量平滑, 具体的做法就是公式7, 使每个点的偏移量, 尽量接近它周围邻居偏移量的平均值.注意tkt_k公式在paper中是错误的 应该为 tk=ck(t)−skRkC0kt_k = c_k(t) - s_kR_kC^0_k因为rk=skRk原创 2017-02-16 07:21:08 · 815 阅读 · 0 评论 -
Least-Squares Fitting of Two 3-D Point Sets
http://ieeexplore.ieee.org/stamp/stamp.jsp?arnumber=4767965原创 2017-08-23 22:23:44 · 2081 阅读 · 0 评论 -
Laplacian surface editing
先讲下各个公式公式(2)是laplacian coordinates的定义公式(5)第一部分是保证laplacian coordinate坐标的一致性, 后面是handle点的约束公式(8)是T的构造公式(12)是求T中的系数2D MATLAB 版在它的project里面自带, 这里将其拓展成3D版本, 源代码如下:func原创 2017-02-15 22:27:35 · 3474 阅读 · 2 评论 -
The Wave kernel Signature: A Quantum Mechanical Approach to shape Analysis
相对于HKS, WKS是模拟粒子在某一时刻tt,出现在地点为xx的概率,表示为ψ(x,t)\psi(x,t) HKS的缺点是它强调了低频部分, 而忽略了高频的影响. 为了解决这个问题WKS在方程里面加了一个ii, 变成复数. {引用[1],66页 A mathematical solution is to multiply the Laplace-Beltrami operator by the原创 2017-09-06 21:12:47 · 1455 阅读 · 3 评论 -
Modeling Deformable Objects from a Single Depth Camera
1 partial surface →(point correspondence)→ warp partial surface →(assemble)→complete watertight surface2 section 3In the initialization step, … into 3D meshes.颜色部分用SIFT提取salient features 深度部分用来进行3D原创 2017-09-19 04:08:40 · 422 阅读 · 0 评论 -
map-based exploration of intrinsic shape differences and variability
这篇主要用矩阵来测量两个shape之间的area distortion和comformal distortion2) 图二中V(f)V(f)说明how much ff is distorted by FF, 3)Theorem 2中, f,gf,g都作用于MM上 4)公式(3)的推导 根据Theorem 2, fTHMDg=fTFTHNFgf^TH^MDg = f^TF^TH^NFg HM原创 2017-09-09 17:49:04 · 516 阅读 · 0 评论 -
KinectFusion: Real-time 3D Reconstruction and Interaction Using a Moving Depth Camera
1) Depth map conversion vi(u)=Di(u)K−1[u,1]v_i(u) = D_i(u)K^{-1}[u,1]可以找出深度图中每 个像素对应的camera view中的坐标2) Camera tracking 这里论文中的算法写得不太清楚 直接对照着源码来看吧 pcl/gpu/kinfu/src/cuda/estimate_combined.cu__device_原创 2017-10-05 04:34:31 · 1466 阅读 · 0 评论 -
KinectFusion: Real-Time Dense Surface Mapping and Tracking
1) 3.1 u以像素单位, u∈[0,640]×[0,480]u \in [0,640] \times [0,480]2) 3.2 注意这里Rk(u)R_k(u)和Dk(u)D_k(u)的单位, Kinnect采回来的数据是以millimeter (mm), 在论文中没有考虑单位问题, 实际编程的时候,是要考虑的, kinnect采集的时候存成的是mm, http://wiki.ros原创 2017-11-06 00:45:31 · 1113 阅读 · 0 评论 -
unique Signatures of Histograms for local surface description
这篇paper就是模仿这SIFT来做的, 不过SIFT是2D的,而它是3D的用在MESH的。对于MESH上的一点,首先要确认该点的local reference frame, 怎么确认, 第3章有讲确认完reference frame之后, 空间分区是按照reference frame来分的, 而且每个区的bin的分块也是通过邻居点的法向量于reference frame Z轴方向的夹角来原创 2018-01-05 04:33:48 · 1031 阅读 · 1 评论 -
A Concise and Provably Informative Multi-Scale Signature Based on Heat Diffusion
这篇文章有很多理论基础我没有看懂, 感兴趣的同学请参考HSU02这本书1) 先说figure 1, 曲线图是描述不同时间段, scaled HKS的值, 最开始1,2,3,4这四点相同, 因为这时还没有扩散, 到最后1,2 变成一类, 3,4 变成一类, 这是因为,1,2扩散到了头, 而3,4,扩散到了尾, 而头和尾有很大区别, 所以出现情况描述的情况2) 第3页 Thus bo原创 2017-09-02 04:04:19 · 923 阅读 · 0 评论