SKLEARN——Novelty and Outlier Detection
简介
很多方法都可以检测一个新的检测样本,是符合当前样本分布的成员还是不一样的利群点。通常,这些方法被用来对真实数据集进行清洗。这些检测方法可以分为两种:
novelty detection: | |
---|---|
The training data is not polluted by outliers, and we are interested in detecting anomalies in new observations. | |
outlier detection: | |
The training data contains outliers, and we need to fit the central mode of the training data, ignoring the deviant observations. |
- 奇异点检测:训练数据中没有离群点,我们是对检测新发现的样本点感兴趣;
- 异常点检测:训练数据中包含离群点,我们需要适配训练数据中的中心部分(密集的部分),忽视异常点;
Skleran的套路
也是sklearn成为机器学习神器的原因。提供了很多机器学习工具estimator,我们要做的就是:
- 根据数据特点选择合适的estimator;
- estimator.fit(X_train)训练;
- estimator.predict(X_test)预测;
最后输出1为正常,-1为异常,就是这么简单粗暴╮(╯▽╰)╭
奇异点检测(Novelty Detection)
样本数n;特征数p;待测样本一个
那么,这个待测样本到底是不是自己人(是不是符合原来数据的概率)。
概率学上认为,所有的数据都有它的隐藏的分布模式,这种分布模式可以由概率模型来具象化。一般来说,通过原始数据学习到的概率模型在p维空间上的表示,是一个边界凹凸的紧密收缩的边界形状。然后,如果新的样本点落在边界内,就是自己人;反之,就是奇异点。
sklearn工具:
svm.OneClassSVM 优化参数为核函数和边界的尺度参数。下面是官方的例子:
import numpy as np
import matplotlib.pyplot as plt
import matplotlib.font_manager
from sklearn import svm
xx, yy = np.meshgrid(np.linspace(-5, 5, 500), np.linspace(-5, 5, 500))
# Generate train data
# fit the model
y_pred_outliers = clf.predict(X_outliers) # 异常测试集的标签
n_error_train = y_pred_train[y_pred_train == -1].size
n_error_test = y_pred_test[y_pred_test == -1].size
n_error_outliers = y_pred_outliers[y_pred_outliers == 1].size
# plot the line, the points, and the nearest vectors to the plane
Z = clf.decision_function(np.c_[xx.ravel(), yy.ravel()])
Z = Z.reshape(xx.shape)
plt.title("Novelty Detection")
plt.contourf(xx, yy, Z, levels=np.linspace(Z.min(), 0, 7), cmap=plt.cm.PuBu)
a = plt.contour(xx, yy, Z, levels=[0], linewidths=2, colors='darkred')
plt.contourf(xx, yy, Z, levels=[0, Z.max()], colors='palevioletred')
s = 40
b1 = plt.scatter(X_train[:, 0], X_train[:, 1], c='white', s=s)
b2 = plt.scatter(X_test[:, 0], X_test[:, 1], c='blueviolet', s=s)
c = plt.scatter(X_outliers[:, 0], X_outliers[:, 1], c='gold', s=s)
plt.axis('tight')
plt.xlim((-5, 5))
plt.ylim((-5, 5))
plt.legend([a.collections[0], b1, b2, c],
["learned frontier", "training observations",
"new regular observations", "new abnormal observations"],
loc="upper left",
prop=matplotlib.font_manager.FontProperties(size=11))
plt.xlabel(
"error train: %d/200 ; errors novel regular: %d/40 ; "
"errors novel abnormal: %d/40"
% (n_error_train, n_error_test, n_error_outliers))
import matplotlib.pyplot as plt
import matplotlib.font_manager
from sklearn import svm
xx, yy = np.meshgrid(np.linspace(-5, 5, 500), np.linspace(-5, 5, 500))
# Generate train data
X = 0.3 * np.random.randn(100, 2)
X_train = np.r_[X + 2, X - 2] # 全是正常的,预测值应为1
# Generate some regular novel observations
X = 0.3 * np.random.randn(20, 2)
X_test = np.r_[X + 2, X - 2] # 全是正常的,
预测值应为1
# Generate some abnormal novel observations
X_outliers = np.random.uniform(low=-4, high=4, size=(20, 2)) # 全是异常的,
预测值应为-1
# fit the model
clf = svm.OneClassSVM(nu=0.1, kernel="rbf", gamma=0.1)
clf.fit(X_train)
y_pred_train = clf.predict(X_train) # 训练集的标签
y_pred_test = clf.predict(X_test) # 正常测试集的标签y_pred_outliers = clf.predict(X_outliers) # 异常测试集的标签
n_error_train = y_pred_train[y_pred_train == -1].size
n_error_test = y_pred_test[y_pred_test == -1].size
n_error_outliers = y_pred_outliers[y_pred_outliers == 1].size
# plot the line, the points, and the nearest vectors to the plane
Z = clf.decision_function(np.c_[xx.ravel(), yy.ravel()])
Z = Z.reshape(xx.shape)
plt.title("Novelty Detection")
plt.contourf(xx, yy, Z, levels=np.linspace(Z.min(), 0, 7), cmap=plt.cm.PuBu)
a = plt.contour(xx, yy, Z, levels=[0], linewidths=2, colors='darkred')
plt.contourf(xx, yy, Z, levels=[0, Z.max()], colors='palevioletred')
s = 40
b1 = plt.scatter(X_train[:, 0], X_train[:, 1], c='white', s=s)
b2 = plt.scatter(X_test[:, 0], X_test[:, 1], c='blueviolet', s=s)
c = plt.scatter(X_outliers[:, 0], X_outliers[:, 1], c='gold', s=s)
plt.axis('tight')
plt.xlim((-5, 5))
plt.ylim((-5, 5))
plt.legend([a.collections[0], b1, b2, c],
["learned frontier", "training observations",
"new regular observations", "new abnormal observations"],
loc="upper left",
prop=matplotlib.font_manager.FontProperties(size=11))
plt.xlabel(
"error train: %d/200 ; errors novel regular: %d/40 ; "
"errors novel abnormal: %d/40"
% (n_error_train, n_error_test, n_error_outliers))
plt.show()
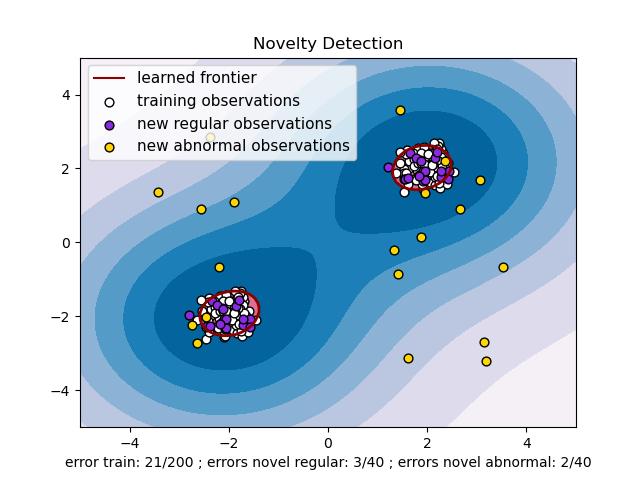
最后的结果并不令人满意,为什么正常的会被判定成异常,如上图所示,确实有几个正常样本点有点离群,但是还有更多分布正常的点被隔离了出去。下面我们来对oneclassSVM进行分析,来找原因。
One-class SVM
首先,SVM是用一个超平面来区分类别一和类别二;而One-class SVM是用无数个超平面区分类别一和类别二三四...。也就是说用于区分本类和异类的边界是有无数超平面组成的。既然这样,这个方法中就应该有参数来调节超平面的选取,使它通融一下,放几个人进来(扩大边界)。
Parameters:
- kernel 根据经验判断数据可能是那种分布。‘linear’:线性;‘rbf’:高斯分布,默认的;等等
- nu 似乎使我们要找的参数,表示训练误差分数(0, 1]。就不能是0吗 (`へ´)
- degree 当核函数是‘poly’多项式的时候有用,表示阶次。
- gamma 相关系数,默认1/n_features
- coef0 不懂
- tol 决定收敛的程度
- shrinking 不懂
- cache_size 没用,不管
- verbose 过程是否显示,默认不显示
- max_iter 最大迭代次数,默认-1,不限制
- random_state 加噪声
拨开云雾见天日,守得云开见月明!
原来上面代码中
clf = svm.OneClassSVM(nu=0.1, kernel="rbf", gamma=0.1)中nu=0.1表示训练集里有10%的叛徒,我们可以把nu尽量调小,但不能是0,这样error train就变小了,但是errors novel abnormal可能会变大。可以交叉验证找一个平衡点。
PS:这里说一下我写这篇文章的目的。最近有一个需求,有n个数据集,每个数据集中有m个数据,每个数据集中的数据都服从高斯分布。但是,有些数据集(k个,k<n)中有异常点,不知道有多少个,我想把这些异常点找出来。约束条件是,不能把正常的判定为异常,尽量能找出所有异常。找一下sklearn里的工具,One-class SVM受参数nu的限制,我不知道对每个数据集把nu设为多少合适,果然还是不行。
异常点检测(Outlier Detection)
异常点监测和奇异值检测有着相同的目的,都是为了把大部分数据聚集的簇与少量的噪声样本点分开。不同的是,对于异常点监测来说,我们没有一个干净的训练集(训练集中也有噪声样本)。
Fitting an elliptic envelope
一个一般的思路是,假设常规数据隐含这一个已知的概率分布。基于这个假设,我们尝试确定数据的形状(边界),也可以将远离边界的样本点定义为异常点。SKlearn提供了一个covariance.EllipticEnvelope类,它可以根据数据做一个鲁棒的协方差估计,然后学习到一个包围中心样本点并忽视离群点的椭圆。
鲁棒的意思是足够稳定,即每次通过EllipticEnvelope对相同数据做出的协方差估计基本相同。
举个例子,假设合群数据都是高斯分布的,那么EllipticEnvelope会鲁棒的估计合群数据的位置和协方差,而不会受到离群数据的影响。从该估计得到的马氏距离用于得出偏离度度量。例子如下:
import numpy as np
import matplotlib.pyplot as plt
from sklearn.covariance import EmpiricalCovariance, MinCovDet
# generate data
gen_cov = np.eye(n_features) # 创建一个2*2的单位矩阵
outliers_cov = np.eye(n_features)
# fit a Minimum Covariance Determinant (MCD) robust estimator to data
robust_cov = MinCovDet().fit(X) # 最小协方差确定
import matplotlib.pyplot as plt
from sklearn.covariance import EmpiricalCovariance, MinCovDet
n_samples = 125 # 125个训练集
n_outliers = 25 # 25个异常点
n_features = 2 # 2维的,为了方便在平面图上展示# generate data
gen_cov = np.eye(n_features) # 创建一个2*2的单位矩阵
gen_cov[0, 0] = 2. # 矩阵的第一个元素改成2
# 随机数矩阵与上面的2*2矩阵点乘,就是为了把分布搞得像个椭圆
X = np.dot(np.random.randn(n_samples, n_features), gen_cov)
# add some outliersoutliers_cov = np.eye(n_features)
X[-n_outliers:] = np.dot(np.random.randn(n_outliers, n_features), outliers_cov) # 125个训练集中有25个被诱反
# fit a Minimum Covariance Determinant (MCD) robust estimator to data
robust_cov = MinCovDet().fit(X) # 最小协方差确定
# compare estimators learnt from the full data set with true parameters
emp_cov = EmpiricalCovariance().fit(X) # 最大协方差
fig
= plt
.figure()
plt .subplots_adjust(hspace =-. 1, wspace =. 4, top =. 95, bottom =. 05) # 设置坐标系(子图)之间的间距
# Show data set
subfig1 = plt .subplot( 3, 1, 1)
inlier_plot = subfig1 .scatter(X[:, 0], X[:, 1],
color = 'black', label = 'inliers')
outlier_plot = subfig1 .scatter(X[:, 0][ -n_outliers:], X[:, 1][ -n_outliers:],
color = 'red', label = 'outliers')
subfig1 .set_xlim(subfig1 .get_xlim()[ 0], 11.) # [-5, 11]
subfig1 .set_title( "Mahalanobis distances of a contaminated data set:")
mahal_emp_cov = mahal_emp_cov .reshape(xx .shape)
emp_cov_contour = subfig1 .contour(xx, yy, np.sqrt(mahal_emp_cov),
cmap =plt .cm .PuBu_r,
linestyles = 'dashed')
mahal_robust_cov = robust_cov .mahalanobis(zz)
mahal_robust_cov = mahal_robust_cov .reshape(xx .shape)
robust_contour = subfig1 .contour(xx, yy, np.sqrt(mahal_robust_cov),
cmap =plt .cm .YlOrBr_r, linestyles = 'dotted')
subfig1 .legend([emp_cov_contour .collections[ 1], robust_contour .collections[ 1],
inlier_plot, outlier_plot],
[ 'MLE dist', 'robust dist', 'inliers', 'outliers'],
loc = "upper right", borderaxespad = 0)
plt .xticks(())
plt .subplots_adjust(hspace =-. 1, wspace =. 4, top =. 95, bottom =. 05) # 设置坐标系(子图)之间的间距
# Show data set
subfig1 = plt .subplot( 3, 1, 1)
inlier_plot = subfig1 .scatter(X[:, 0], X[:, 1],
color = 'black', label = 'inliers')
outlier_plot = subfig1 .scatter(X[:, 0][ -n_outliers:], X[:, 1][ -n_outliers:],
color = 'red', label = 'outliers')
subfig1 .set_xlim(subfig1 .get_xlim()[ 0], 11.) # [-5, 11]
subfig1 .set_title( "Mahalanobis distances of a contaminated data set:")
# Show contours of the distance functions
# np.linspace(-5, 11, 100)表示从-5到11的100个平均采样值
# np.meshgrid()详解见下
xx, yy
=
np.meshgrid(
np.linspace(plt
.xlim()[
0], plt
.xlim()[
1],
100),
# xx.ravel()把xx展开成一维
# np.c_使xx变成第一列,yy变成第二列
# 然后根据马氏距离的平方做同心的椭圆
mahal_emp_cov
= emp_cov
.mahalanobis(zz)
mahal_emp_cov = mahal_emp_cov .reshape(xx .shape)
emp_cov_contour = subfig1 .contour(xx, yy, np.sqrt(mahal_emp_cov),
cmap =plt .cm .PuBu_r,
linestyles = 'dashed')
mahal_robust_cov = robust_cov .mahalanobis(zz)
mahal_robust_cov = mahal_robust_cov .reshape(xx .shape)
robust_contour = subfig1 .contour(xx, yy, np.sqrt(mahal_robust_cov),
cmap =plt .cm .YlOrBr_r, linestyles = 'dotted')
subfig1 .legend([emp_cov_contour .collections[ 1], robust_contour .collections[ 1],
inlier_plot, outlier_plot],
[ 'MLE dist', 'robust dist', 'inliers', 'outliers'],
loc = "upper right", borderaxespad = 0)
plt .xticks(())
plt
.yticks(())
plt
.show()
讲真,这个官方的代码太长了,实在看不懂。奈何想看的电影还没下完,硬着头皮上了╮(╯▽╰)╭
自己的理解都用注释的形式写在了上面。
np.meshgrid(x, y)
返回两个相关向量(x,y)的相关矩阵。
- Parameters:x,y是两个1维的向量,代表一个网格
- Returns:返回矩阵X和Y,shape都是(len(y), len(x)),什么意思呢。
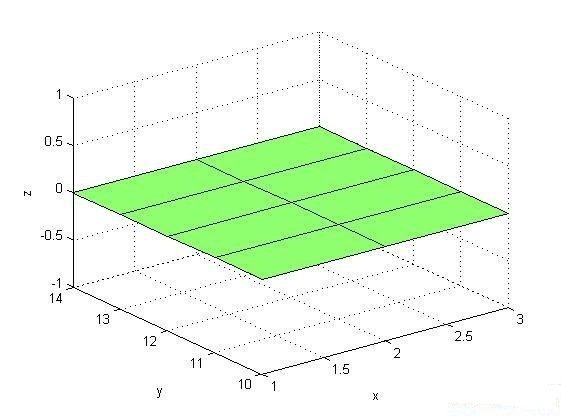
X表示所有交点的x坐标,Y表示所有交点的y坐标
mahalanobis(observations)
计算给定观测样本点的马氏距离的平方
- Parameters:观测点假定和之前训练用的数据集同分布
- Returns:观测点的马氏距离的平方
这个椭圆边界法能不能解决我刚刚的问题呢?首先,输入是带有异常点的数据,且不需要给定有多少异常点掺在里面。但是,我该如何找到输入数据中的异常点呢。有没有输出阈值??或者直接给出异常点的标签??然而,我晕,突然发现sklearn Userguide的2.7.2.1下面给的例子不是elliptic envelope的,怪不得不对劲。查看sklearn.covariance.EllipticEnvelope的方法,果然有predict()。它的例子分析放在后面。
Isolation Forest
孤立森林是一个高效的异常点监测算法。SKLEARN提供了ensemble.IsolationForest模块。该模块在进行检测时,会随机选取一个特征,然后在所选特征的最大值和最小值随机选择一个分切面。该算法下整个训练集的训练就像一颗树一样,递归的划分。划分的次数等于根节点到叶子节点的路径距离d。所有随机树(为了增强鲁棒性,会随机选取很多树形成森林)的d的平均值,就是我们检测函数的最终结果。
那些路径d比较小的,都是因为距离主要的样本点分布中心比较远的。也就是说可以通过寻找最短路径的叶子节点来寻找异常点。它的例子也放在后面。
One-class SVM
严格来说,一分类的SVM并不是一个异常点监测算法,而是一个奇异点检测算法:它的训练集不能包含异常样本,否则的话,可能在训练时影响边界的选取。但是,对于高维空间中的样本数据集,如果它们做不出有关分布特点的假设,One-class SVM将是一大利器。
下面是对三种异常检测算法进行比较,EllipticEnvelope随着训练集变得unimodal效果下降,OneClassSVM会好一点,不受unimodel的影响,而IsolationForest在各种情况下都比较理想。



import
numpy
as
np
from scipy import stats
import matplotlib.pyplot as plt
import matplotlib.font_manager
from sklearn import svm
from sklearn.covariance import EllipticEnvelope
from sklearn.ensemble import IsolationForest
rng = np .random .RandomState( 42)
# Example settings
n_samples = 200
outliers_fraction = 0.25
clusters_separation = [ 0, 1, 2]
# define two outlier detection tools to be compared
classifiers = {
"One-Class SVM": svm.OneClassSVM(nu = 0.95 * outliers_fraction + 0.05,
kernel = "rbf", gamma = 0.1),
"Robust covariance": EllipticEnvelope(contamination =outliers_fraction),
"Isolation Forest": IsolationForest(max_samples =n_samples,
contamination =outliers_fraction,
random_state =rng)}
# Compare given classifiers under given settings
xx, yy = np.meshgrid( np.linspace( - 7, 7, 500), np.linspace( - 7, 7, 500))
n_inliers = int(( 1. - outliers_fraction) * n_samples)
n_outliers = int(outliers_fraction * n_samples)
ground_truth = np.ones(n_samples, dtype = int)
ground_truth[ -n_outliers:] = - 1
# Fit the problem with varying cluster separation
for i, offset in enumerate(clusters_separation):
np.random.seed( 42)
# Data generation
X1 = 0.3 * np.random.randn(n_inliers // 2, 2) - offset
X2 = 0.3 * np.random.randn(n_inliers // 2, 2) + offset
X = np.r_[X1, X2]
# Add outliers
X = np.r_[X, np .random .uniform(low =- 6, high = 6, size =(n_outliers, 2))]
# Fit the model
plt .figure(figsize =( 10.8, 3.6))
for i, (clf_name, clf) in enumerate(classifiers .items()):
# fit the data and tag outliers
clf .fit(X)
scores_pred = clf .decision_function(X)
threshold = stats.scoreatpercentile(scores_pred,
100 * outliers_fraction)
y_pred = clf .predict(X)
n_errors = (y_pred != ground_truth) .sum()
# plot the levels lines and the points
Z = clf .decision_function( np.c_[xx .ravel(), yy .ravel()])
Z = Z .reshape(xx .shape)
subplot = plt .subplot( 1, 3, i + 1)
subplot .contourf(xx, yy, Z, levels = np.linspace(Z .min(), threshold, 7),
cmap =plt .cm .Blues_r)
a = subplot .contour(xx, yy, Z, levels =[threshold],
linewidths = 2, colors = 'red')
subplot .contourf(xx, yy, Z, levels =[threshold, Z .max()],
colors = 'orange')
b = subplot .scatter(X[: -n_outliers, 0], X[: -n_outliers, 1], c = 'white')
c = subplot .scatter(X[ -n_outliers:, 0], X[ -n_outliers:, 1], c = 'black')
subplot .axis( 'tight')
subplot .legend(
[a .collections[ 0], b, c],
[ 'learned decision function', 'true inliers', 'true outliers'],
prop =matplotlib .font_manager .FontProperties(size = 11),
loc = 'lower right')
subplot .set_title( " %d . %s (errors: %d )" % (i + 1, clf_name, n_errors))
subplot .set_xlim(( - 7, 7))
subplot .set_ylim(( - 7, 7))
plt .subplots_adjust( 0.04, 0.1, 0.96, 0.92, 0.1, 0.26)
from scipy import stats
import matplotlib.pyplot as plt
import matplotlib.font_manager
from sklearn import svm
from sklearn.covariance import EllipticEnvelope
from sklearn.ensemble import IsolationForest
rng = np .random .RandomState( 42)
# Example settings
n_samples = 200
outliers_fraction = 0.25
clusters_separation = [ 0, 1, 2]
# define two outlier detection tools to be compared
classifiers = {
"One-Class SVM": svm.OneClassSVM(nu = 0.95 * outliers_fraction + 0.05,
kernel = "rbf", gamma = 0.1),
"Robust covariance": EllipticEnvelope(contamination =outliers_fraction),
"Isolation Forest": IsolationForest(max_samples =n_samples,
contamination =outliers_fraction,
random_state =rng)}
# Compare given classifiers under given settings
xx, yy = np.meshgrid( np.linspace( - 7, 7, 500), np.linspace( - 7, 7, 500))
n_inliers = int(( 1. - outliers_fraction) * n_samples)
n_outliers = int(outliers_fraction * n_samples)
ground_truth = np.ones(n_samples, dtype = int)
ground_truth[ -n_outliers:] = - 1
# Fit the problem with varying cluster separation
for i, offset in enumerate(clusters_separation):
np.random.seed( 42)
# Data generation
X1 = 0.3 * np.random.randn(n_inliers // 2, 2) - offset
X2 = 0.3 * np.random.randn(n_inliers // 2, 2) + offset
X = np.r_[X1, X2]
# Add outliers
X = np.r_[X, np .random .uniform(low =- 6, high = 6, size =(n_outliers, 2))]
# Fit the model
plt .figure(figsize =( 10.8, 3.6))
for i, (clf_name, clf) in enumerate(classifiers .items()):
# fit the data and tag outliers
clf .fit(X)
scores_pred = clf .decision_function(X)
threshold = stats.scoreatpercentile(scores_pred,
100 * outliers_fraction)
y_pred = clf .predict(X)
n_errors = (y_pred != ground_truth) .sum()
# plot the levels lines and the points
Z = clf .decision_function( np.c_[xx .ravel(), yy .ravel()])
Z = Z .reshape(xx .shape)
subplot = plt .subplot( 1, 3, i + 1)
subplot .contourf(xx, yy, Z, levels = np.linspace(Z .min(), threshold, 7),
cmap =plt .cm .Blues_r)
a = subplot .contour(xx, yy, Z, levels =[threshold],
linewidths = 2, colors = 'red')
subplot .contourf(xx, yy, Z, levels =[threshold, Z .max()],
colors = 'orange')
b = subplot .scatter(X[: -n_outliers, 0], X[: -n_outliers, 1], c = 'white')
c = subplot .scatter(X[ -n_outliers:, 0], X[ -n_outliers:, 1], c = 'black')
subplot .axis( 'tight')
subplot .legend(
[a .collections[ 0], b, c],
[ 'learned decision function', 'true inliers', 'true outliers'],
prop =matplotlib .font_manager .FontProperties(size = 11),
loc = 'lower right')
subplot .set_title( " %d . %s (errors: %d )" % (i + 1, clf_name, n_errors))
subplot .set_xlim(( - 7, 7))
subplot .set_ylim(( - 7, 7))
plt .subplots_adjust( 0.04, 0.1, 0.96, 0.92, 0.1, 0.26)
plt
.show()
在上面三种分类器的定义中,都需要给定outliers_fraction,也就是说都需要知道原始训练集中有多少异常点,所以都不符合我当前问题的需要。