#导入库
import pandas
import seaborn as sns
import numpy as np
import os
import matplotlib.pyplot as plt
#设置需要分析相关性的参数,我这里共有16个参数需要作相关性分析
names = ['h-ave','h-max','h-min','h-std','h-area','h-time','h-var','h-entropy','a-ave','a-max','a-min','a-std','a-area','a-time','a-var','a-entropy'] #设置变量名
#设置路径
os.chdir(os.getcwd()) #os.getcwd()获取当前路径,os.chdir(...)改变路径为...
#输入数据
data = pandas.read_table('lal3.txt',sep='\s+') #点击打开链接,参考此文对pandas读取文件的用法
#求解相关系数
correlations = data.corr()
correction=abs(correlations)# 取绝对值,只看相关程度 ,不关心正相关还是负相关
# plot correlation matrix
fig = plt.figure()
ax = fig.add_subplot(figsize=(20,20)) #图片大小为20*20
ax = sns.heatmap(correction,cmap=plt.cm.Greys, linewidths=0.05,vmax=1, vmin=0 ,annot=True,annot_kws={'size':6,'weight':'bold'})
#热力图参数设置(相关系数矩阵,颜色,每个值间隔等)
#ticks = numpy.arange(0,16,1) #生成0-16,步长为1
plt.xticks(np.arange(16)+0.5,names) #横坐标标注点
plt.yticks(np.arange(16)+0.5,names) #纵坐标标注点
#ax.set_xticks(ticks) #生成刻度
#ax.set_yticks(ticks)
#ax.set_xticklabels(names) #生成x轴标签
#ax.set_yticklabels(names)
ax.set_title('Characteristic correlation')#标题设置
plt.savefig('cluster.tif',dpi=300)
plt.show()
结果展示:
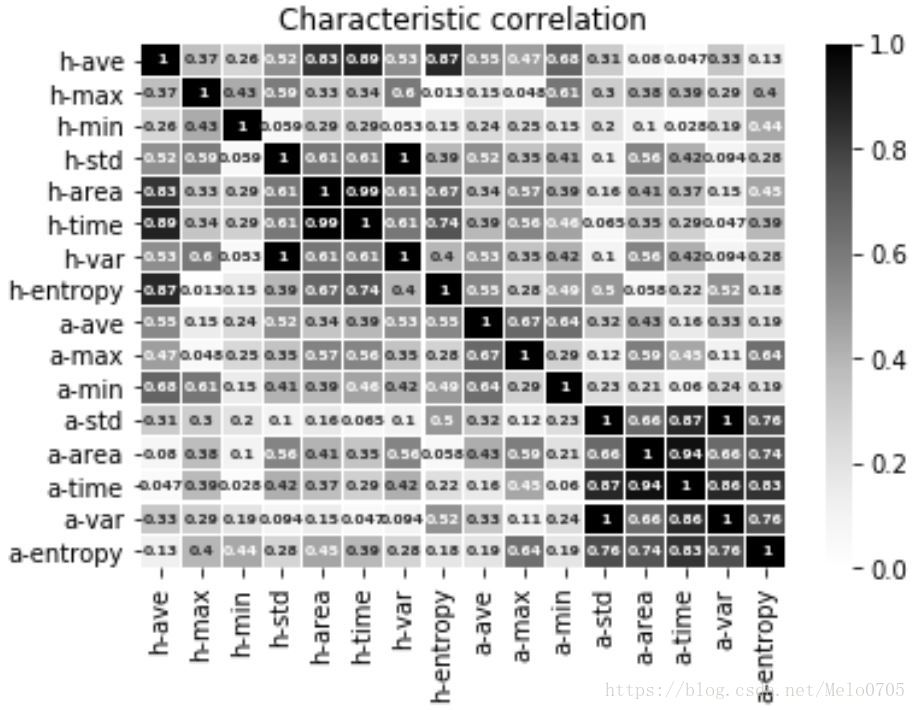
程序注解:
1:seaborn 是一个精简的python库,可以创建具有统计意义的图表,能理解pandas的DataFrame类型。详见官网
点击打开链接。
2:pandas.DataFrame.corr#Pandas库中的求解相关系数(计算列的两两相关,不包括NA/null值)函数,用法如下,来自官网解释
DataFrame.corr(method='pearson', min_periods=1) 数据名.corr(方法参数1,参数2),
method : {‘pearson’, ‘kendall’, ‘spearman’}
pearson : standard correlation coefficient 标准的相关系数(默认缺省值),卡尔·皮尔逊设计的统计指标,是研究变量之间线性相关程度的量
kendall : Kendall Tau correlation coefficient 肯德尔τ相关系数
spearman : Spearman rank correlation 斯皮尔曼等级相关
#连续数据,正态分布,线性关系,用pearson相关系数是最恰当
DataFrame.corr(method='pearson', min_periods=1) 数据名.corr(方法参数1,参数2),
method : {‘pearson’, ‘kendall’, ‘spearman’}
pearson : standard correlation coefficient 标准的相关系数(默认缺省值),卡尔·皮尔逊设计的统计指标,是研究变量之间线性相关程度的量
kendall : Kendall Tau correlation coefficient 肯德尔τ相关系数
spearman : Spearman rank correlation 斯皮尔曼等级相关
#连续数据,正态分布,线性关系,用pearson相关系数是最恰当
min_periods : int, optional(缺省为1)