import numpy as np
import pandas as pd
import matplotlib.pyplot as plt
df=pd.read_csv('ex1data1-Copy1.txt',header=None,names=['population','profit'])
df.head()
| population | profit |
---|
0 | 6.1101 | 17.5920 |
---|
1 | 5.5277 | 9.1302 |
---|
2 | 8.5186 | 13.6620 |
---|
3 | 7.0032 | 11.8540 |
---|
4 | 5.8598 | 6.8233 |
---|
df_np=df.values
df_np=np.insert(df_np,0,1.0,axis=1)
df_np.shape
(97, 3)
X=df_np[:,0:2]
X=np.matrix(X)
X.shape
(97, 2)
x1=df.iloc[:,0].values
y=df.iloc[:,1].values
plt.scatter(x1,y,color='r')
plt.xlabel('population')
plt.ylabel('profit')
plt.show()
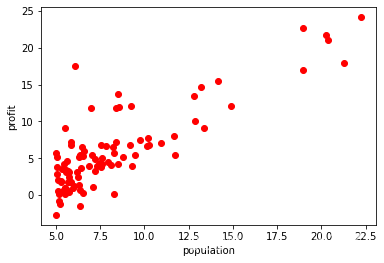
y=y.reshape(97,1)
y=np.matrix(y)
y.shape
(97, 1)
梯度下降实现
'X:97x2'
'theta:列向量,2x1 '
def cost(X,y,theta):
inn=np.sum(np.power((X*theta-y),2))
return inn/(2*len(y))
theta=[0.1,0.1]
theta=np.matrix(theta).reshape(2,1)
theta.shape
(2, 1)
loss_0=cost(X,y,theta)
loss_0
25.449553111855668
def GredientDec(X,y,theta,iters,alpha):
parameters=X.shape[1]
loss=np.zeros((iters,1))
theta_fig=theta
for a in range(iters):
error=(X*theta-y)
for j in range(parameters):
term=np.sum(np.multiply(error,X[:,j]))
theta[j]=theta[j]-(alpha*term)/len(y)
loss[a]=cost(X,y,theta)
return theta,loss
np.seterr(invalid='ignore')
theta,loss=GredientDec(X,y,theta=theta,iters=1000,alpha=0.01)
theta
matrix([[-3.78565572],
[ 1.18197038]])
loss_new=cost(X,y,theta)
loss_new
4.478075461131649
x=np.linspace(X[:,1].min(),X[:,1].max(),100)
y_fig=theta[0]+theta[1]*x
y_fig=y_fig.reshape(100,1)
x1=df.iloc[:,0].values
y=df.iloc[:,1].values
plt.scatter(x1,y,color='r')
plt.plot(x,y_fig,color='k')
plt.xlabel('population')
plt.ylabel('profit')
plt.show()
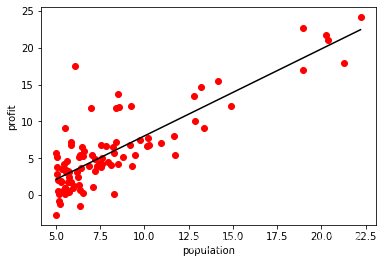
绘制损失函数
iters=1000
plt.plot(np.arange(iters),loss,color='r')
plt.xlabel('numbers of iter')
plt.ylabel('loss of J(θ)')
plt.show()
绘制等高线图
J=[]
for i in np.arange(-10,10,0.1):
for j in np.arange(-10,10,0.1):
theta=np.matrix([i,j]).reshape(2,1)
J.append(cost(X,y,theta=theta))
J=np.array(J).reshape(200,200)
plt.contour(np.arange(-10,10,0.1),np.arange(-10,10,0.1),J,levels=20)
<matplotlib.contour.QuadContourSet at 0x173d9b6a8b0>
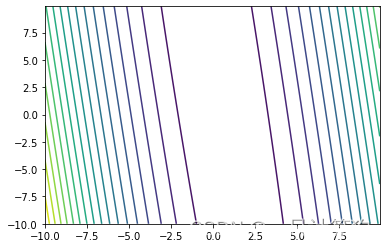