1.Vector的使用和清除里面所有的内容:
vector<CvPoint2D64d>edgepoint;
edgepoint.clear();//清除所有的东西。
C++ vector删除特定元素的方法如下:
for(it = v.begin();it!=v.end();){
if(*it == 3){
#define _aaa_
class aaa
{
};
#endif
#ifndef _STDIO_H_ //这给_STDIO_H_ 可以任意取,表示出现过的这个东西则不再进行声明,没出现过的则进行#define _STDIO_H_
#define _STDIO_H_
......
#endif
2.cvDrawContours函数如何控制只画一条轮廓和画全部轮廓
其函数原型为:
函数原型:void cvDrawContours( CvArr *img, CvSeq* contour,
CvScalar external_color, CvScalar hole_color,
int max_level, int thickness=1,
int line_type=8, CvPoint offset=cvPoint(0,0) );
例子:cvDrawContours(drawimg,contour,CV_RGB(255,0,0),CV_RGB(250,0,0,),0,1,8);//这里的cvDrawContours
第五个参数为负数或0,其是表示只画当前的轮廓,而不是把contour里的所以轮廓都画出来
hSobel(pGrayImage,pEdgeImage,pGradMat,pDirection,10,120);//pGradMat为梯度图,288ms
/*切记,其中的pGrayImage是灰度图,而不是二值图,pEdgeImage才是二值图。如果边缘内是白色,外围是黑色时,cvFindContours使用CV_RETR_LIST
与CV_RETR_EXTERNAL参数检测到的边缘是一个,如果是这里的边缘大概位置才是白色的,则其CV_RETR_LIST会检测到两条边缘,一个是内边缘,另一个是
外边缘,如果不想其平均来计算边缘点,就要使用CV_RETR_EXTERNAL来只检测外边缘。
*/
int mun= cvFindContours(pEdgeImage, storage, &contour, sizeof(CvContour), CV_RETR_EXTERNAL, CV_CHAIN_APPROX_SIMPLE); //8mms
for (; contour != 0;contour=contour->h_next)
{
double contours_len = cvArcLength(contour, CV_WHOLE_SEQ, -1);
if (contours_len>360) //这里的面积太大则不能进入循环
{
hSubPixelEdge(pGradMat, pEdgeImge_copy, pDirection, pSubEdgeMatH, pSubEdgeMatW, &edgepoint, contour);
for (size_t i = 0; i < edgepoint.size(); i++)
{
x[i] = edgepoint[i].x;
y[i] = edgepoint[i].y;
if (i>1)
{
cvLine(drawimg1, cvPoint(cvRound(x[i]), cvRound(y[i])), cvPoint(cvRound(x[i-1]), cvRound(y[i- 1])), cvScalar(0,255,0),2);
}
}
N = edgepoint.size();
CircleSim1(x, y, N);
poit.x = a;
poit.y = b;
poit.r = r;
circle.push_back(poit);
edgepoint.clear();
}
}
5.cout时,会输出1.#INF或者-1.#INF,代表什么?
case 1:
nG0=(pGradImage+h*nGradWidthStep)[w-1];//这些提取的是梯度值
nG1=(pGradImage+h*nGradWidthStep)[w];
nG2=(pGradImage+h*nGradWidthStep)[w+1];
valuex = nG0 + nG2 - 2 * nG1;
if (valuex >= -EPSILON && valuex <= EPSILON)
{
fEdgeH = h;
fEdgeW = w;
}
else
{
fEdgeH = h;
fEdgeW = w + ((double)(nG0 - nG2) / (nG0 + nG2 - 2 * nG1))*0.5;
//cout<<"1h:"<<h<<" w:"<<w<<" fw:"<<fEdgeW<<" "<<nG0<<" "<<nG2<<" "<<nG1<<endl;
//cout << "1= " << valuex << endl;
}
break;
6. Mat图像类型的初始化,例子:
7、如何通过RGB来划分颜色:
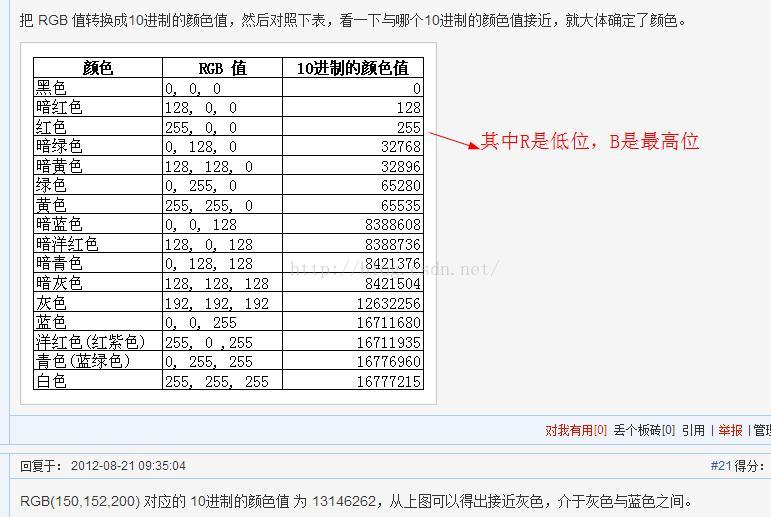
import cv2
from skimage.color import rgb2hsv
import numpy as np
if __name__ == '__main__':
#把原始图像的RGB转换为0-1的值
img_rgb=(np.array([[[130,66,241]]])/255.).astype(dtype='float32')
# img_rgb=cv2.imread("temp/timg.jpg")
#这样子得出的结果才符合理论值:
cv_HSV = cv2.cvtColor(img_rgb, cv2.COLOR_RGB2HSV)
print(cv_HSV)
print(rgb2hsv(img_rgb))
skimage_HSV = np.array(rgb2hsv(img_rgb) * 255).astype(np.uint8)
print(skimage_HSV)
输出结果为:
[[[261.94284 0.726141 0.94509804]]]
[[[0.727619 0.7261411 0.94509804]]]
[[[185 185 241]]]
然后,对于HSV:
On output 0≤V≤1, 0≤S≤1, 0≤H≤360.
The values are then converted to the destination data type:
8-bit images:
V <- V*255, S <- S*255, H <- H/2 (to fit to 0..255)
16-bit images (currently not supported):
V <- V*65535, S <- S*65535, H <- H
32-bit images:
H, S, V are left as is
对于CIE(Lab):
On output 0≤L≤100, -127≤a≤127, -127≤b≤127
The values are then converted to the destination data type:
8-bit images:
L <- L*255/100, a <- a + 128, b <- b + 128
16-bit images are currently not supported
32-bit images:
L, a, b are left as is