##读取图片并将图片中的像素点数据标准化
import os
import struct
import numpy as np
def load_mnist(path, kind='train'):
"""Load MNIST data from `path`"""
labels_path = os.path.join(path,
'%s-labels-idx1-ubyte' % kind)
images_path = os.path.join(path,'%s-images-idx3-ubyte' % kind)
with open(labels_path, 'rb') as lbpath:
#其中>表示big-endian,I表示无符号整数
magic, n = struct.unpack('>II',lbpath.read(8))
labels = np.fromfile(lbpath,
dtype=np.uint8)
with open(images_path, 'rb') as imgpath:
magic, num, rows, cols = struct.unpack(">IIII",
imgpath.read(16))
images = np.fromfile(imgpath,
dtype=np.uint8).reshape(
len(labels), 784)
#标准化像素值到-1到1
images = ((images / 255.) - .5) * 2
return images, labels
##展示数据的维数
X_train, y_train = load_mnist('', kind='train')
print('Rows: %d, columns: %d'% (X_train.shape[0], X_train.shape[1]))
X_test, y_test = load_mnist('', kind='t10k')
print('Rows: %d, columns: %d'% (X_test.shape[0], X_test.shape[1]))
##展示示例特征矩阵中的784像素向量重新整形后的数字0-9原始的28×28图像,不同的手写字体的不同
fig, ax = plt.subplots(nrows=5,
... ncols=5,
... sharex=True,
... sharey=True)
ax = ax.flatten()
for i in range(25):
... img = X_train[y_train == 7][i].reshape(28, 28)
... ax[i].imshow(img, cmap='Greys')
ax[0].set_xticks([])
ax[0].set_yticks([])
plt.tight_layout()
plt.show()
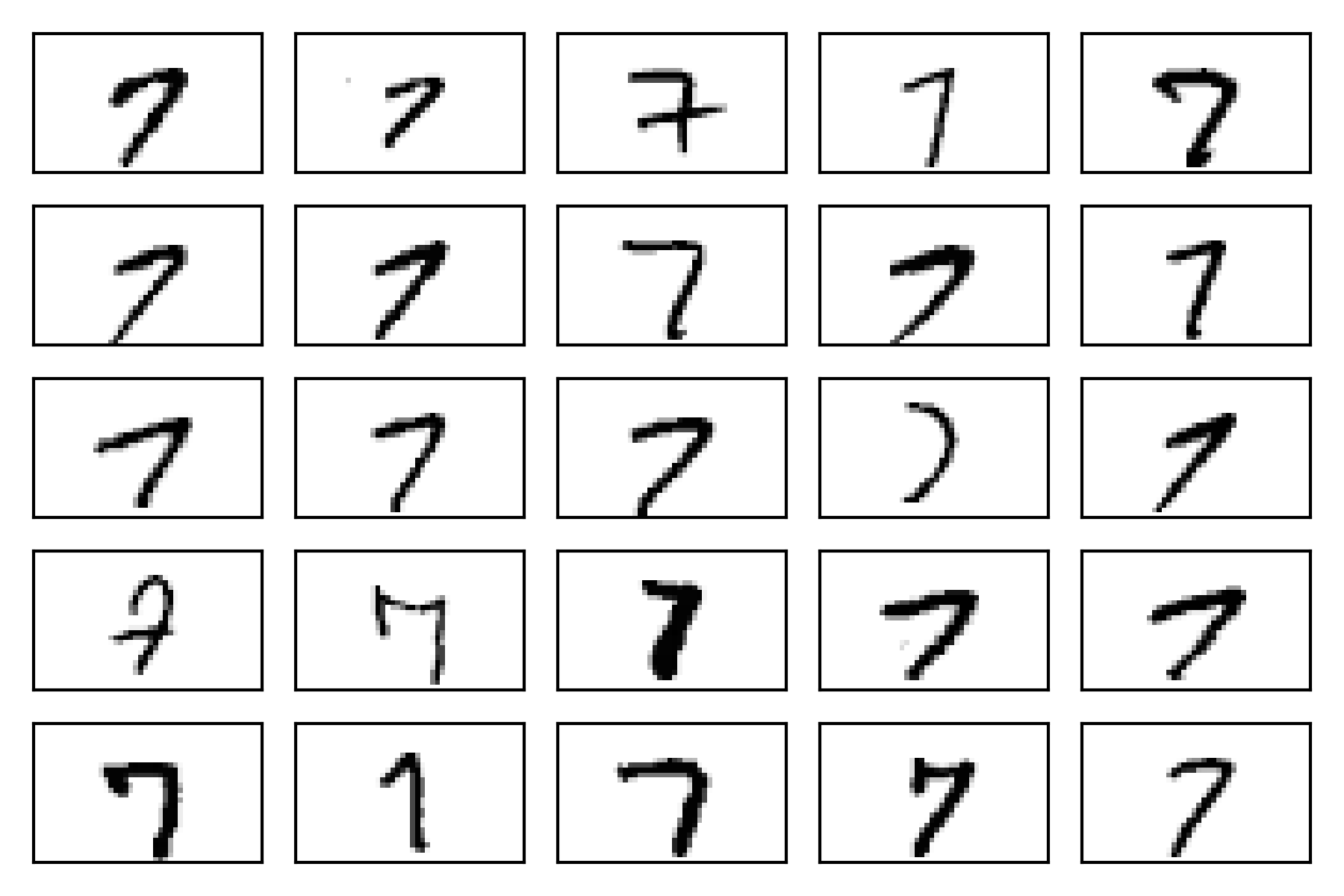
#train the MLP using 55,000 samples from the already shuffled MNIST training dataset
#and use the remaining 5,000 samples for validation during training
import numpy as np
import sys
class NeuralNetMLP(object):
""" Feedforward neural network / Multi-layer perceptron classifier.
Parameters
------------
n_hidden : int (default: 30)
Number of hidden units.
l2 : float (default: 0.)
Lambda value for L2-regularization.
No regularization if l2=0. (default)
epochs : int (default: 100)
Number of passes over the training set.
eta : float (default: 0.001)
Learning rate.
shuffle : bool (default: True)
Shuffles training data every epoch if True to prevent circles.
minibatch_size : int (default: 1)
Number of training samples per minibatch.
seed : int (default: None)
Random seed for initalizing weights and shuffling.
Attributes
-----------
eval_ : dict
Dictionary collecting the cost, training accuracy,
and validation accuracy for each epoch during training.
"""
def __init__(self, n_hidden=30,
l2=0., epochs=100, eta=0.001,
shuffle=True, minibatch_size=1, seed=None):
self.random = np.random.RandomState(seed)
self.n_hidden = n_hidden
self.l2 = l2
self.epochs = epochs
self.eta = eta
self.shuffle = shuffle
self.minibatch_size = minibatch_size
def _onehot(self, y, n_classes):
"""Encode labels into one-hot representation
Parameters
------------
y : array, shape = [n_samples]
Target values.
Returns
-----------
onehot : array, shape = (n_samples, n_labels)
"""
onehot = np.zeros((n_classes, y.shape[0]))
for idx, val in enumerate(y):
onehot[val, idx] = 1.
return onehot.T
def _sigmoid(self, z):
"""Compute logistic function (sigmoid)"""
return 1. / (1. + np.exp(-np.clip(z, -250, 250)))
def _forward(self, X):
"""Compute forward propagation step"""
# step 1: net input of hidden layer
# [n_samples, n_features] dot [n_features, n_hidden]
# -> [n_samples, n_hidden]
z_h = np.dot(X, self.w_h) + self.b_h
# step 2: activation of hidden layer
a_h = self._sigmoid(z_h)
# step 3: net input of output layer
# [n_samples, n_hidden] dot [n_hidden, n_classlabels]
# -> [n_samples, n_classlabels]
z_out = np.dot(a_h, self.w_out) + self.b_out
# step 4: activation output layer
a_out = self._sigmoid(z_out)
return z_h, a_h, z_out, a_out
def _compute_cost(self, y_enc, output):
"""Compute cost function.
Parameters
----------
y_enc : array, shape = (n_samples, n_labels)
one-hot encoded class labels.
output : array, shape = [n_samples, n_output_units]
Activation of the output layer (forward propagation)
Returns
---------
cost : float
Regularized cost
"""
L2_term = (self.l2 *
(np.sum(self.w_h ** 2.) +
np.sum(self.w_out ** 2.)))
term1 = -y_enc * (np.log(output))
term2 = (1. - y_enc) * np.log(1. - output)
cost = np.sum(term1 - term2) + L2_term
# If you are applying this cost function to other
# datasets where activation
# values maybe become more extreme (closer to zero or 1)
# you may encounter "ZeroDivisionError"s due to numerical
# instabilities in Python & NumPy for the current implementation.
# I.e., the code tries to evaluate log(0), which is undefined.
# To address this issue, you could add a small constant to the
# activation values that are passed to the log function.
#
# For example:
#
# term1 = -y_enc * (np.log(output + 1e-5))
# term2 = (1. - y_enc) * np.log(1. - output + 1e-5)
return cost
def predict(self, X):
"""Predict class labels
Parameters
-----------
X : array, shape = [n_samples, n_features]
Input layer with original features.
Returns:
----------
y_pred : array, shape = [n_samples]
Predicted class labels.
"""
z_h, a_h, z_out, a_out = self._forward(X)
y_pred = np.argmax(z_out, axis=1)
return y_pred
def fit(self, X_train, y_train, X_valid, y_valid):
""" Learn weights from training data.
Parameters
-----------
X_train : array, shape = [n_samples, n_features]
Input layer with original features.
y_train : array, shape = [n_samples]
Target class labels.
X_valid : array, shape = [n_samples, n_features]
Sample features for validation during training
y_valid : array, shape = [n_samples]
Sample labels for validation during training
Returns:
----------
self
"""
n_output = np.unique(y_train).shape[0] # number of class labels
n_features = X_train.shape[1]
########################
# Weight initialization
########################
# weights for input -> hidden
self.b_h = np.zeros(self.n_hidden)
self.w_h = self.random.normal(loc=0.0, scale=0.1,
size=(n_features, self.n_hidden))
# weights for hidden -> output
self.b_out = np.zeros(n_output)
self.w_out = self.random.normal(loc=0.0, scale=0.1,
size=(self.n_hidden, n_output))
epoch_strlen = len(str(self.epochs)) # for progress formatting
self.eval_ = {'cost': [], 'train_acc': [], 'valid_acc': []}
y_train_enc = self._onehot(y_train, n_output)
# iterate over training epochs
for i in range(self.epochs):
# iterate over minibatches
indices = np.arange(X_train.shape[0])
if self.shuffle:
self.random.shuffle(indices)
for start_idx in range(0, indices.shape[0] - self.minibatch_size +
1, self.minibatch_size):
batch_idx = indices[start_idx:start_idx + self.minibatch_size]
# forward propagation
z_h, a_h, z_out, a_out = self._forward(X_train[batch_idx])
##################
# Backpropagation
##################
# [n_samples, n_classlabels]
sigma_out = a_out - y_train_enc[batch_idx]
# [n_samples, n_hidden]
sigmoid_derivative_h = a_h * (1. - a_h)
# [n_samples, n_classlabels] dot [n_classlabels, n_hidden]
# -> [n_samples, n_hidden]
sigma_h = (np.dot(sigma_out, self.w_out.T) *
sigmoid_derivative_h)
# [n_features, n_samples] dot [n_samples, n_hidden]
# -> [n_features, n_hidden]
grad_w_h = np.dot(X_train[batch_idx].T, sigma_h)
grad_b_h = np.sum(sigma_h, axis=0)
# [n_hidden, n_samples] dot [n_samples, n_classlabels]
# -> [n_hidden, n_classlabels]
grad_w_out = np.dot(a_h.T, sigma_out)
grad_b_out = np.sum(sigma_out, axis=0)
# Regularization and weight updates
delta_w_h = (grad_w_h + self.l2*self.w_h)
delta_b_h = grad_b_h # bias is not regularized
self.w_h -= self.eta * delta_w_h
self.b_h -= self.eta * delta_b_h
delta_w_out = (grad_w_out + self.l2*self.w_out)
delta_b_out = grad_b_out # bias is not regularized
self.w_out -= self.eta * delta_w_out
self.b_out -= self.eta * delta_b_out
#############
# Evaluation
#############
# Evaluation after each epoch during training
z_h, a_h, z_out, a_out = self._forward(X_train)
cost = self._compute_cost(y_enc=y_train_enc,
output=a_out)
y_train_pred = self.predict(X_train)
y_valid_pred = self.predict(X_valid)
train_acc = ((np.sum(y_train == y_train_pred)).astype(np.float) /
X_train.shape[0])
valid_acc = ((np.sum(y_valid == y_valid_pred)).astype(np.float) /
X_valid.shape[0])
sys.stderr.write('\r%0*d/%d | Cost: %.2f '
'| Train/Valid Acc.: %.2f%%/%.2f%% ' %
(epoch_strlen, i+1, self.epochs, cost,
train_acc*100, valid_acc*100))
sys.stderr.flush()
self.eval_['cost'].append(cost)
self.eval_['train_acc'].append(train_acc)
self.eval_['valid_acc'].append(valid_acc)
return self
nn.fit(X_train=X_train[:55000],y_train=y_train[:55000],
X_valid=X_train[55000:],y_valid=y_train[55000:])

import matplotlib.pyplot as plt
plt.plot(range(nn.epochs), nn.eval_['cost'])
plt.ylabel('Cost')
plt.xlabel('Epochs')
plt.show()
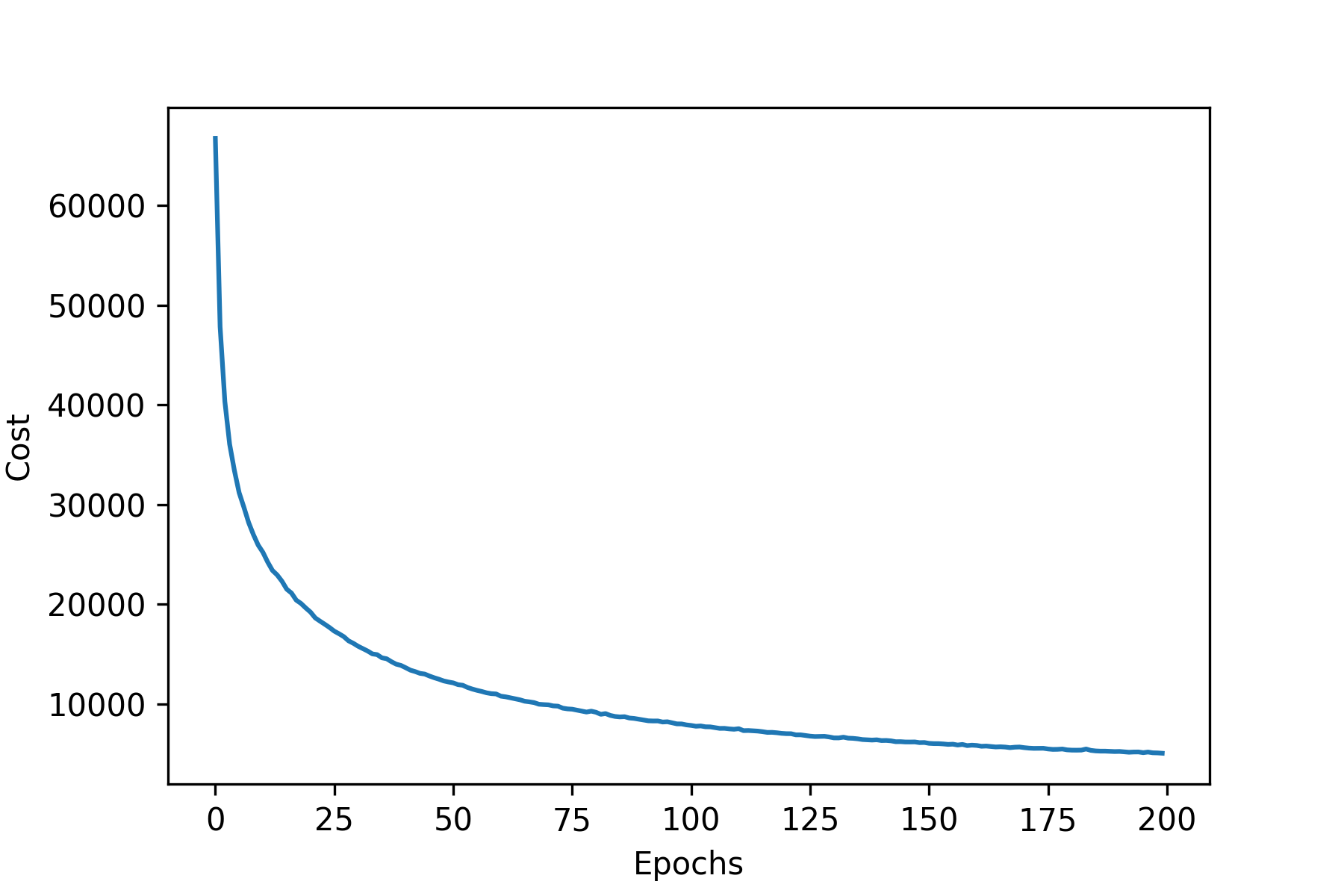
plt.plot(range(nn.epochs), nn.eval_['train_acc'],label='training')
plt.plot(range(nn.epochs), nn.eval_['valid_acc'],label='validation', linestyle='--')
plt.ylabel('Accuracy')
plt.xlabel('Epochs')
plt.legend()
plt.show()
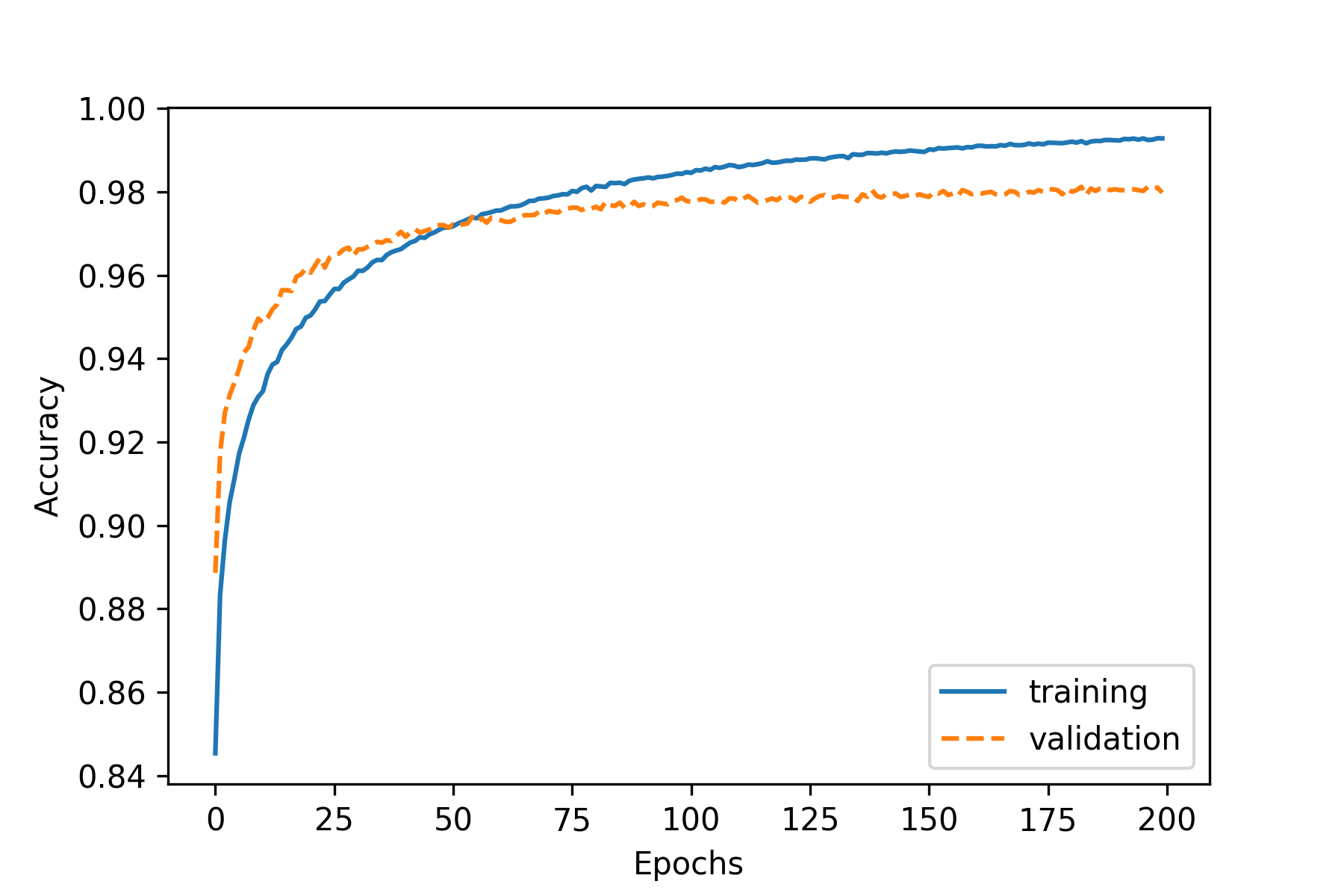
该图显示训练和验证准确度之间的差距增加我们训练网络的Epochs越来越多。
大约在第50个Epochs,训练和验证准确度值相等,然后网络开始过拟和训练数据。
y_test_pred = nn.predict(X_test)
acc = (np.sum(y_test == y_test_pred).astype(np.float) / X_test.shape[0])
print('Training accuracy: %.2f%%' % (acc * 100))
#查看25个被错误分类的例子
miscl_img = X_test[y_test != y_test_pred][:25]
correct_lab = y_test[y_test != y_test_pred][:25]
miscl_lab= y_test_pred[y_test != y_test_pred][:25]
fig, ax = plt.subplots(nrows=5,
ncols=5,sharex=True, sharey=True,)
ax = ax.flatten()
for i in range(25):
... img = miscl_img[i].reshape(28, 28)
... ax[i].imshow(img,
... cmap='Greys',
... interpolation='nearest')
... ax[i].set_title('%d) t: %d p: %d'
... % (i+1, correct_lab[i], miscl_lab[i]))
ax[0].set_xticks([])
ax[0].set_yticks([])
plt.tight_layout()
plt.show()
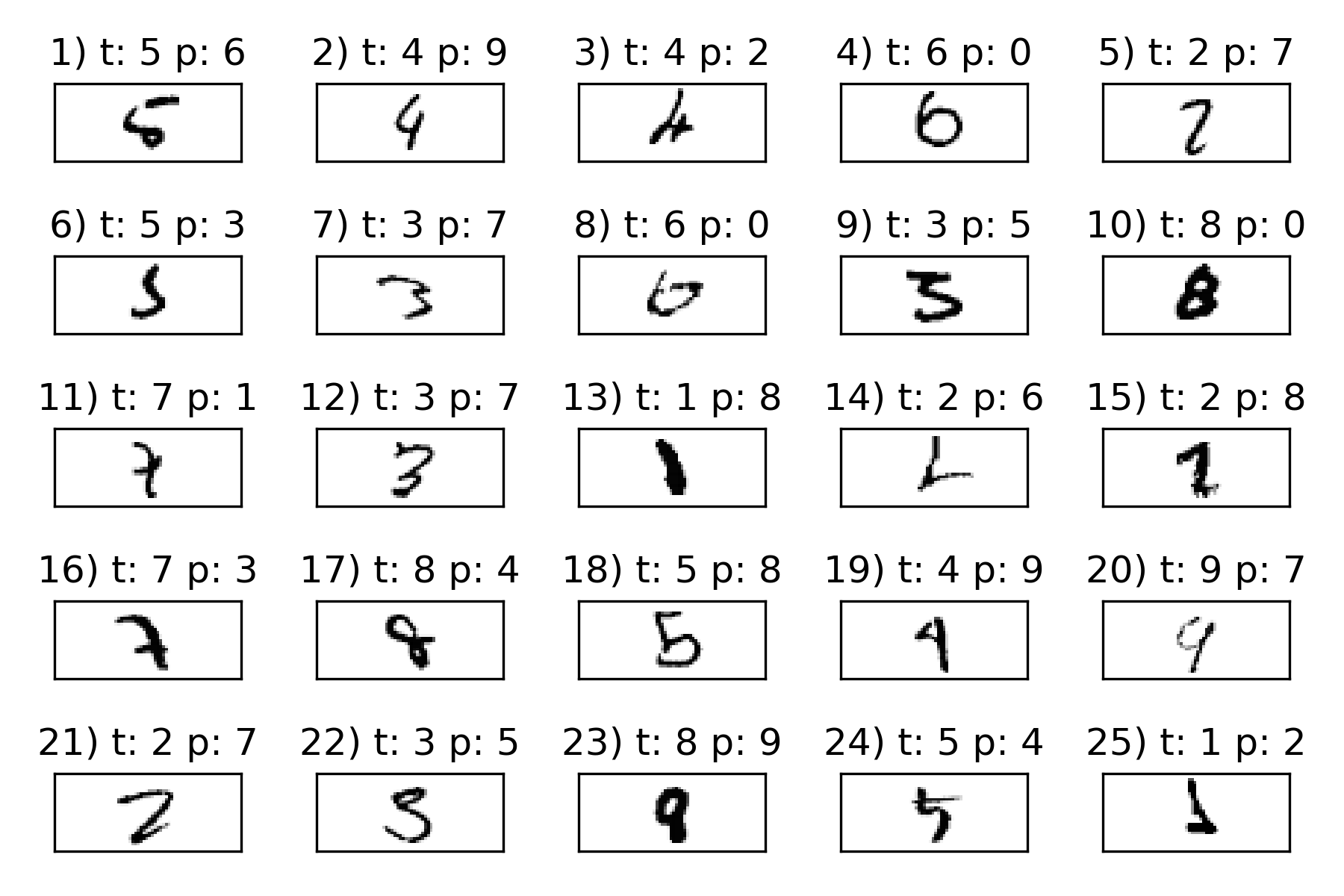
引入tensorflow,对上述的算法进行改进:在这里我们将用双曲线代替隐藏层中的逻辑单元切线激活函数(tanh),用softmax替换输出中的逻辑函数,并添加一个额外的隐藏层。
import tensorflow as tf
n_features = X_train_centered.shape[1]
n_classes = 10
random_seed = 123
np.random.seed(random_seed)
g = tf.Graph()
with g.as_default():
tf.set_random_seed(random_seed)
tf_x = tf.placeholder(dtype=tf.float32,
shape=(None, n_features),
name='tf_x')
tf_y = tf.placeholder(dtype=tf.int32,
shape=None, name='tf_y')
y_onehot = tf.one_hot(indices=tf_y, depth=n_classes)
h1 = tf.layers.dense(inputs=tf_x, units=50,
activation=tf.tanh,
name='layer1')
h2 = tf.layers.dense(inputs=h1, units=50,
activation=tf.tanh,
name='layer2')
logits = tf.layers.dense(inputs=h2,
units=10,
activation=None,
name='layer3')
predictions = {
'classes' : tf.argmax(logits, axis=1,
name='predicted_classes'),
'probabilities' : tf.nn.softmax(logits,
name='softmax_tensor')
}
## define cost function and optimizer:
with g.as_default():
cost = tf.losses.softmax_cross_entropy(
onehot_labels=y_onehot, logits=logits)
optimizer = tf.train.GradientDescentOptimizer(
learning_rate=0.001)
train_op = optimizer.minimize(
loss=cost)
init_op = tf.global_variables_initializer()
#return a generator batches of data
def create_batch_generator(X, y, batch_size=128, shuffle=False):
X_copy = np.array(X)
y_copy = np.array(y)
if shuffle:
data = np.column_stack((X_copy, y_copy))
np.random.shuffle(data)
X_copy = data[:, :-1]
y_copy = data[:, -1].astype(int)
for i in range(0, X.shape[0], batch_size):
yield (X_copy[i:i+batch_size, :], y_copy[i:i+batch_size])>>> ## create a session to launch the graph
>>> sess = tf.Session(graph=g)
>>> ## run the variable initialization operator
>>> sess.run(init_op)
>>>
>>> ## 50 epochs of training:
>>> for epoch in range(50):
... training_costs = []
... batch_generator = create_batch_generator(
... X_train_centered, y_train,
... batch_size=64, shuffle=True)
... for batch_X, batch_y in batch_generator:
... ## prepare a dict to feed data to our network:
... feed = {tf_x:batch_X, tf_y:batch_y}
... _, batch_cost = sess.run([train_op, cost],
feed_dict=feed)
... training_costs.append(batch_cost)
... print(' -- Epoch %2d '
... 'Avg. Training Loss: %.4f' % (
... epoch+1, np.mean(training_costs)
... ))
>>> ## do prediction on the test set:
>>> feed = {tf_x : X_test_centered}
>>> y_pred = sess.run(predictions['classes'],
... feed_dict=feed)
>>>
>>> print('Test Accuracy: %.2f%%' % (
... 100*np.sum(y_pred == y_test)/y_test.shape[0]))
使用karas生成神经网络
#set the random seed for NumPy and TensorFlow to get consistent results
>>> import tensorflow as tf
>>> import tensorflow.contrib.keras as keras
>>> np.random.seed(123)
>>> tf.set_random_seed(123)
#convert the class labels (integers 0-9) into the one-hot format
>>> y_train_onehot = keras.utils.to_categorical(y_train)
>>> print('First 3 labels: ', y_train[:3])
First 3 labels: [5 0 4]
>>> print('\nFirst 3 labels (one-hot):\n', y_train_onehot[:3])
#initialize a new model using
model = keras.models.Sequential()
#add there layers ,first two layers each have 50 hidden units with tanh activation function and last layer has 10 layers #for 10 class labels and use softmax to give the probability of each class.
model.add(
keras.layers.Dense(
units=50,
input_dim=X_train_centered.shape[1],
kernel_initializer='glorot_uniform',
bias_initializer='zeros',
activation='tanh'))
model.add(
keras.layers.Dense(
units=50,
input_dim=50,
kernel_initializer='glorot_uniform',
bias_initializer='zeros',
activation='tanh'))
model.add(
keras.layers.Dense(
units=y_train_onehot.shape[1],
input_dim=50,
kernel_initializer='glorot_uniform',
bias_initializer='zeros',
activation='softmax'))
sgd_optimizer = keras.optimizers.SGD(
lr=0.001, decay=1e-7, momentum=.9)
#设置随机梯度下降
model.compile(optimizer=sgd_optimizer,
loss='categorical_crossentropy')
#train the MLP over 50 epochs,by setting verbose=1,we can follow the
#optimization of the cost function during training
>>> history = model.fit(X_train_centered, y_train_onehot,
... batch_size=64, epochs=50,
... verbose=1,
... validation_split=0.1)
#print the model accuracy on training and test sets
>>> y_train_pred = model.predict_classes(X_train_centered,
... verbose=0)
>>> correct_preds = np.sum(y_train == y_train_pred, axis=0)
>>> train_acc = correct_preds / y_train.shape[0]
>>>
>>> print('First 3 predictions: ', y_train_pred[:3])
First 3 predictions: [5 0 4]
>>>
>>> print('Training accuracy: %.2f%%' % (train_acc * 100))
>>> y_test_pred = model.predict_classes(X_test_centered,
... verbose=0)
>>> correct_preds = np.sum(y_test == y_test_pred, axis=0)
>>> test_acc = correct_preds / y_test.shape[0]
>>> print('Test accuracy: %.2f%%' % (test_acc * 100))