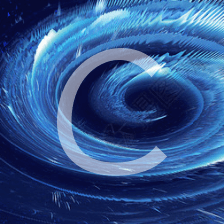
深度学习
文章平均质量分 57
令狐傻笑
图像算法工程师,主要研究方向为图像分类,目标检测
展开
-
基于pytorch和torchvision实现DeformableConv
相关代码如下:import torchimport torchvision.opsfrom torch import nnclass DeformableConv2d(nn.Module): def __init__(self, in_channels, out_channels, kernel_size=3, stride=1,原创 2021-10-13 17:20:49 · 2706 阅读 · 2 评论 -
基于PyTorch的卷积神经网络图像分类——猫狗大战(二):使用Pytorch定义网络模型
文章目录1. 需要用到的库2. 模型定义3. 测试基于上一篇文章https://blog.youkuaiyun.com/linghu8812/article/details/100044971,这次介绍一下网络模型的定义。1. 需要用到的库import torchimport torch.nn as nnfrom torchvision.models import resnet50基于ResNet50定义网络模型,在此基础上进行微调。2. 模型定义class CatVSDogNet(nn.Module原创 2021-07-27 17:08:19 · 865 阅读 · 0 评论 -
Ubuntu18.04配置yolov5目标检测
Ubuntu18.04配置yolov5目标检测原创 2021-06-02 09:18:52 · 1512 阅读 · 0 评论 -
Ubuntu18.04 配置mmdetection实现Faster RCNN目标检测
1.安装mmcv1.1 安装方法方法1pip install mmcv-full -f ttps://download.openmmlab.com/mmcv/dist/{cu_version}/{torch_version}/index.html方法2pip install mmcv-full -f https://download.openmmlab.com/mmcv/dist/cu110/torch1.7.0/index.html方法3git clone https://g原创 2021-05-22 10:26:47 · 406 阅读 · 1 评论 -
训练fast-reid模型
1. 下载项目git clone git@github.com:JDAI-CV/fast-reid.git2. 安装依赖安装requirements.txtconda create -n fastreid python=3.7conda activate fastreidconda install pytorch==1.6.0 torchvision tensorboard -c pytorchpip install -r docs/requirements.txt编译rank_c原创 2021-04-20 10:11:23 · 882 阅读 · 0 评论 -
mmpose PyTorch模型转TensorRT
文章目录mmpose PyTorch模型转TensorRT1. github开源代码2. PyTorch模型转ONNX模型3. ONNX模型转TensorRT模型3.1 概述3.2 编译3.3 运行4. 推理结果mmpose PyTorch模型转TensorRT1. github开源代码yolov5 TensorRT推理的开源代码位置在https://github.com/linghu8812/tensorrt_inference/tree/master/mmpose,PyTorch转onnx的代码是原创 2021-03-06 09:10:25 · 1459 阅读 · 4 评论 -
yolov5 custom数据集查看anchors
1. 查看anchorsyolov5模型在训练自己的数据集之前会进行autoanchor check,autoanchor check会自动对数据集中的ground truth boxes进行key means聚类,产生新的anchors以提高模型检测的mAP,可以通过以下代码查看新的生成的anchors:import torchfrom models.experimental import attempt_loadmodel = attempt_load('./weights/yolov5s.原创 2020-12-03 15:17:30 · 3055 阅读 · 0 评论 -
深度学习模型剪枝、量化和TensorRT推理
深度学习模型剪枝、量化和TensorRT推理模型剪枝算法参考文献:Rethinking the Value of Network Pruning (ICLR 2019)github:https://github.com/Eric-mingjie/rethinking-network-pruningRethinking the Value of Network Pruning这篇文献主要介绍了以下几种剪枝算法,并在github上开源了代码,在ImageNet和cifar两个数据集上进行了测试,论文原创 2020-11-10 20:24:36 · 4647 阅读 · 1 评论 -
MXNet Symbol Batch Normalization fix_gamma=True转ONNX方法
1. 问题当MXNet模型的Batch Normalization的fix_gamma参数为True时,会导致转ONNX模型失败,此时输出的ONNX参数如下图所示,导致ONNX的推理结果和MXNet不一致。2. 解决方法出现MXNet Batch Normalization的fix_gamma参数等于True时,可以手动修改batchnorm_gamma参数值,使ONNX模型输出正常,相关代码如下:sym, arg_params, aux_params = mx.model.load_check原创 2020-11-03 11:13:55 · 554 阅读 · 0 评论 -
深度学习模型PyTorch训练并转ONNX与TensorRT部署
深度学习模型PyTorch训练与TensorRT部署原创 2020-10-06 15:25:19 · 2296 阅读 · 1 评论 -
Ubuntu18.04 基于detectron2实现Faster RCNN目标检测(三)——Faster RCNN检测单张图片
1. demo.py在图片上实现单张图片的检测可以通过以下命令实现python3 demo.py --config-file ../configs/PascalVOC-Detection/faster_rcnn_R_50_FPN.yaml \ --input cat.jpg \ --output result_cat_voc.jpg \ --opts MODEL.WEIGHTS ....原创 2020-05-26 17:25:36 · 1408 阅读 · 0 评论 -
Ubuntu18.04配置darknet环境实现YOLOv4目标检测(二)——基于YOLOv4训练自己数据
目录1. 数据准备1.1 下载VOC数据1.2 生成数据标签1.3 下载预训练模型2. 训练数据2.1 准备cfg文件2.2 准备训练脚本文件sh完成darknet环境配置后,可以在darknet框架下进行目标检测数据训练。1. 数据准备1.1 下载VOC数据参考https://pjreddie.com/darknet/yolo/网站介绍,下载VOC数据。wget https://pjr...原创 2020-04-27 14:46:04 · 7561 阅读 · 34 评论 -
Ubuntu18.04 基于detectron2实现Faster RCNN训练VOC数据(二)
1.准备VOC数据下载VOC数据,将VOC数据放在datasets文件夹下,VOC2007和VOC2012两个文件夹分开放,文件夹下结构不用改变。datasets|----VOC2007|----VOC20122. 数据训练通过以下命令开始训练。CUDA_VISIBLE_DEVICES=0 python3 train_net.py \ --config-file ../confi...原创 2020-04-23 15:48:34 · 3325 阅读 · 2 评论 -
Ubuntu18.04 配置detectron2实现Faster RCNN目标检测和Mask RCNN实例分割(一)
1. 背景介绍detectron2项目地址:https://github.com/facebookresearch/detectron2FasterRCNN论文:https://arxiv.org/abs/1506.01497COCO数据集:http://cocodataset.org/#homeVOC数据集:http://host.robots.ox.ac.uk/pascal/VOC/...原创 2020-04-23 10:53:27 · 4278 阅读 · 11 评论 -
基于PyTorch实现cosine learning rate
1.需要用到的库设置学习率和模型import mathimport matplotlib.pyplot as pltimport torch.optim as optimfrom torchvision.models import resnet18lr_rate = 0.1model = resnet18(num_classes=10)2.LambdaLR实现cosine...原创 2020-03-26 16:49:55 · 6597 阅读 · 0 评论 -
InsightFace算法学习
目录InsightFace算法学习开源仓库识别算法配置相关算法算法测试参考文献InsightFace算法学习开源仓库InsightFace: https://github.com/deepinsight/insightface识别算法配置安装mxnetpip install mxnet-cu80 #or mxnet-cu90 or mxnet-cu100下载insightfac...原创 2019-12-05 16:01:23 · 4228 阅读 · 4 评论 -
Ubuntu18.04配置darknet环境实现YOLOv4目标检测(一)——配置YOLOv4环境darknet
目录1. 概述2.darknet配置2.1 下载darknet2.2 编译darknet3.测试3.1 测试图片3.2 测试视频1. 概述YOLO官方网址:https://pjreddie.com/darknet/yolo/作者开源项目地址:https://github.com/pjreddie/darknetYOLOv1论文地址:https://...原创 2019-09-16 00:18:15 · 10227 阅读 · 17 评论 -
Ubuntu18.04 配置maskrcnn-benchmark实现Faster RCNN目标检测和Mask RCNN实例分割
目录1. 环境要求2. 安装步骤2.1 安装cocoapi2.2 安装apex2.3 配置maskrcnn-benchmark maskrcnn-benchmark是facebook research开源的目标检测和实例分割的算法仓库,可以实现的模型有Faster RCNN,Mask RCNN,RetinaNet等。1. 环境要求PyTorch...原创 2019-09-06 23:18:21 · 1070 阅读 · 0 评论 -
基于PyTorch的卷积神经网络图像分类——猫狗大战(一):使用Pytorch定义DataLoader
目录1. 需要用到的库2. 数据扩充定义3. 自定义Dataset4. 测试 开始一个新的系列,基于Kaggle比赛的猫狗大战数据集,基于PyTorch实现猫狗图像分类。 数据集地址在:https://www.kaggle.com/c/dogs-vs-cats-redux-kernels-edition/overview。 ...原创 2019-08-23 23:01:38 · 4611 阅读 · 0 评论 -
深度学习经典论文及开源代码
目录综述Deep Learning图像分类LeNetAlexNetZFNetInception系列VGGResNetDenseNetResNeXtSENetEfficientNet目标检测RCNNOverFeatSPPFast RCNNFaster RCNNR-FCNFPNSSD系列YOLO系列Reti...原创 2019-03-14 23:57:08 · 6551 阅读 · 1 评论