前言
本文主要有两个目的:
- 推导卷积运算各个变量的梯度公式;
- 学习如何扩展Pytorch算子,自己实现了一个能够forward和backward的卷积算子;
首先介绍了计算图的自动求导方法,然后对卷积运算中Kernel和Input的梯度进行了推导,之后基于Pytorch实现了卷积算子并做了正确性检验。
本文的代码在这个GitHub仓库。
计算图
计算图(Computational Graphs)是torch.autograd
自动求导的理论基础,描述为一个有向无环图(DAG),箭头的方向是前向传播(forward)的方向,而逆向的反向传播(backward)的过程可以很方便地对任意变量求偏导。为了方便说明,这里举一个简单的例子:
a
=
x
2
,
b
=
3
a
,
c
=
b
y
其中
x
,
y
是输入,
c
是输出。
a=x^2,b=3a,c=by \\ 其中x,y是输入,c是输出。
a=x2,b=3a,c=by其中x,y是输入,c是输出。
根据链式求导法则我们可得:
∂
c
∂
x
=
∂
c
∂
b
∂
b
∂
a
∂
a
∂
x
=
6
x
y
∂
c
∂
y
=
b
=
3
x
2
\begin{aligned} \frac{\partial c}{\partial x}&=\frac{\partial c}{\partial b}\frac{\partial b}{\partial a}\frac{\partial a}{\partial x}=6xy \\ \frac{\partial c}{\partial y}&=b=3x^2 \end{aligned}
∂x∂c∂y∂c=∂b∂c∂a∂b∂x∂a=6xy=b=3x2
在Pytorch(Python)里定义上述三个函数:
def square(x):
return x ** 2
def mul3(x):
return x * 3
def mul_(x, y):
return x * y
然后用torchviz
可视化其复合函数的计算图:
x = torch.tensor(3., requires_grad=True, dtype=torch.float)
y = torch.tensor(2., requires_grad=True, dtype=torch.float)
a = square(x) # a=x^2
b = mul3(a) # b=3a
c = mul_(b, y) # c=by
torchviz.make_dot(c, {"x": x, "y": y, "c": c}).view()
得到如下结果:
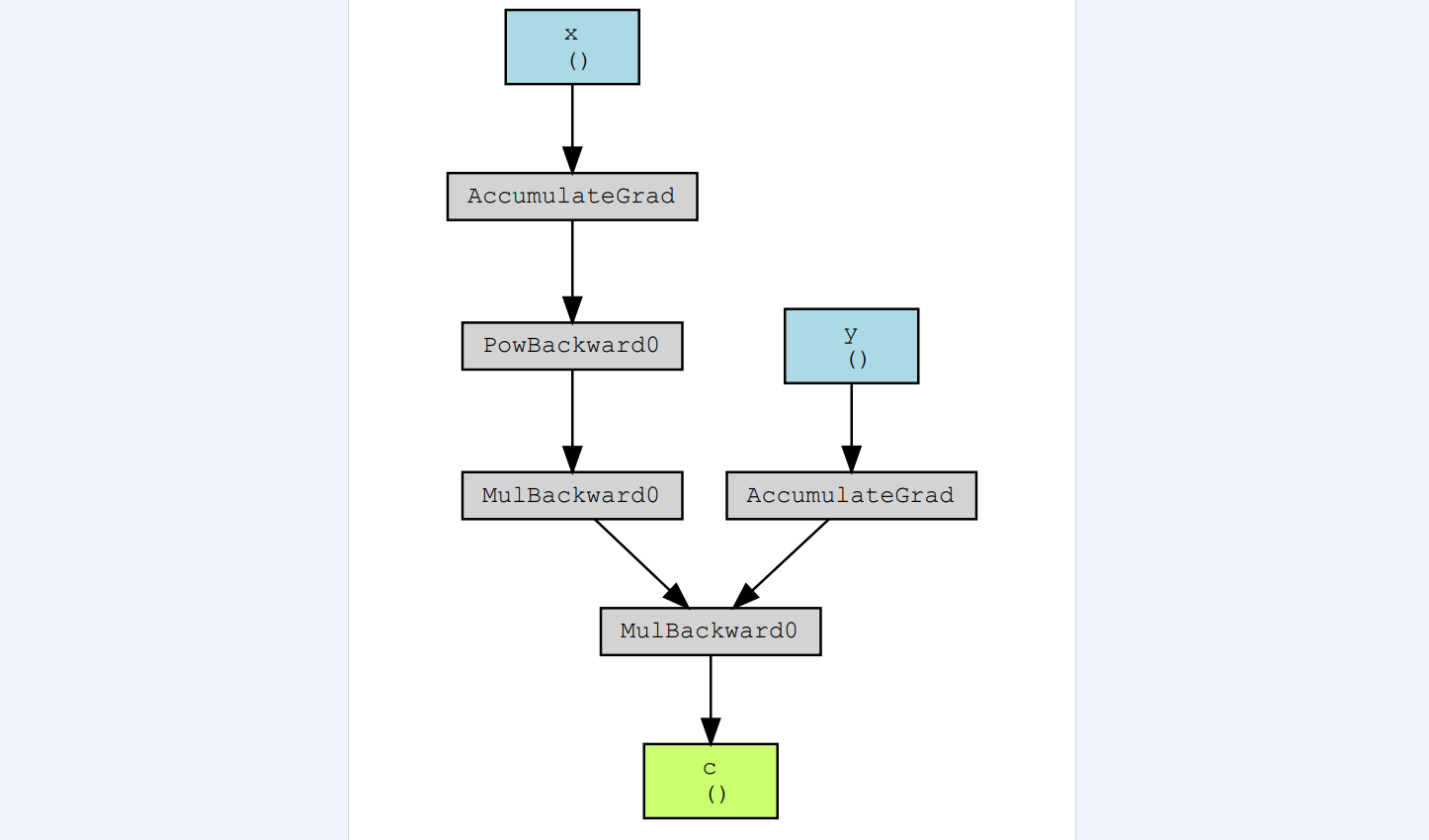
忽略“Accumulate”这个操作,在该计算图上的反向求导过程表示如下:
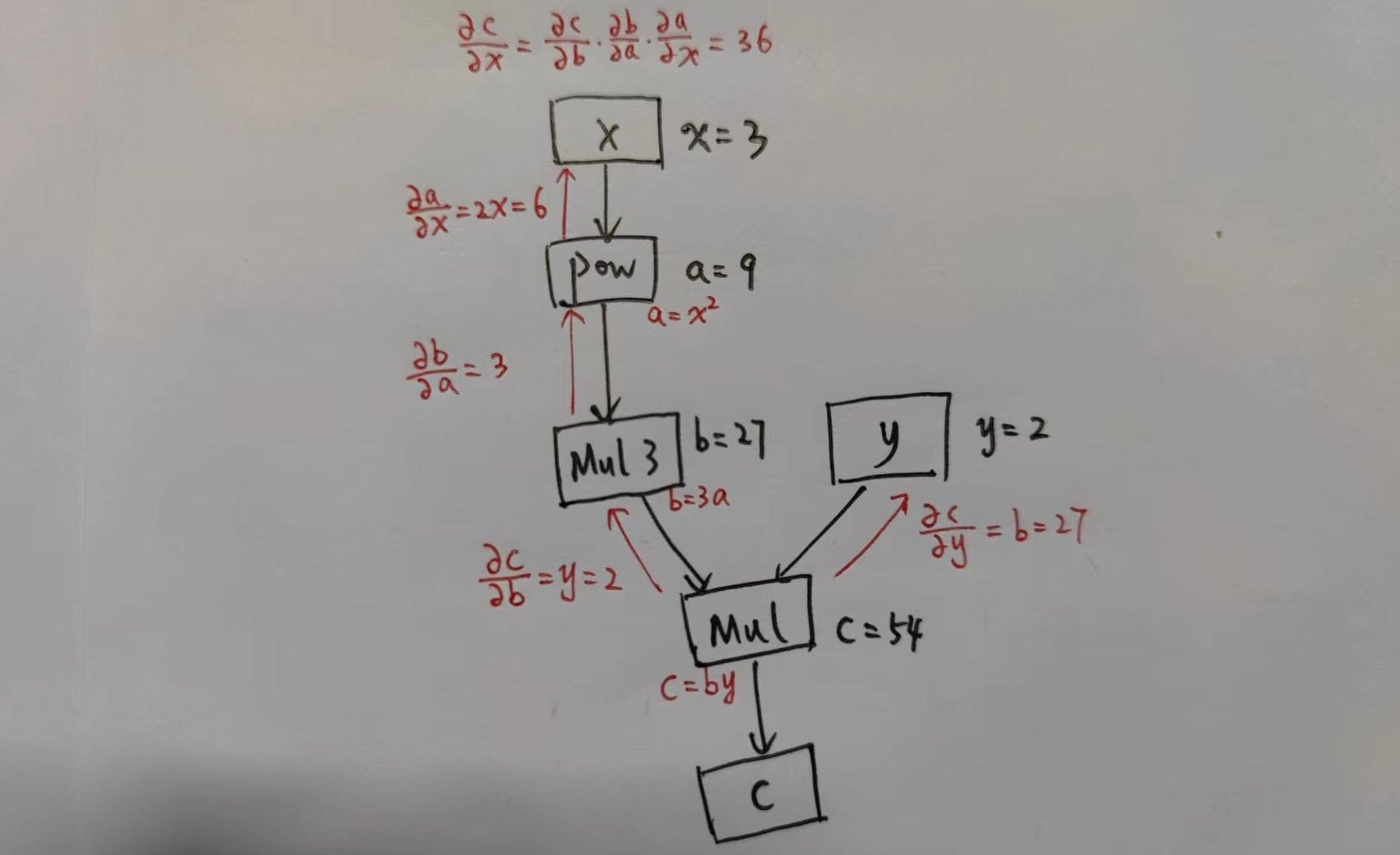
这很清晰地展示了计算图的功能,它记录了每一个变量(包括输出、中间变量)的计算函数(可以称之为一个算子,就是图中的方框,入边是输入,出边是输出),从而可以数值计算出相应的导数。实际上,任何变量 q q q对 p p p求导都可以对两者之间的反向链路进行累乘得到。
对输出
c
c
c调用.backward()
后,可以查看导数值:
c.backward()
print(y.grad)
print(x.grad)
输出结果和上图的计算结果一致。注意在backward过程中非叶子节点可以调用.retain_grad()
来记录grad。
以前我一直以为自动求导是一个很复杂的操作,没想到一个计算图就非常简洁地实现了,才发现“我以为”的复杂操作其实是形式化的求导……
卷积运算与梯度推导
本文所涉及的卷积运算是最平凡的卷积运算,不包含stride, padding, dilation, bias等。定义卷积运算
O
u
t
p
u
t
=
I
n
p
u
t
∗
K
e
r
n
e
l
,
Output=Input*Kernel,
Output=Input∗Kernel,
其中
I
n
p
u
t
=
T
e
n
s
o
r
[
B
,
C
i
n
,
N
,
N
]
Input=Tensor[B,C_{in},N,N]
Input=Tensor[B,Cin,N,N]为输入,
K
e
r
n
e
l
=
T
e
n
s
o
r
[
C
o
u
t
,
C
i
n
,
K
,
K
]
Kernel=Tensor[C_{out},C_{in},K,K]
Kernel=Tensor[Cout,Cin,K,K]为卷积核,
O
u
t
p
u
t
=
T
e
n
s
o
r
[
B
,
C
o
u
t
,
M
,
M
]
Output=Tensor[B,C_{out},M,M]
Output=Tensor[B,Cout,M,M]为输出,且有
M
=
N
−
K
+
1
M=N-K+1
M=N−K+1。
如何实现卷积?
可以先用nn.Unfold
将输入的tensor展开,注意Unfold()也是可以指定stride, dilation等参数的,但我们这里不考虑这些,因此只用传入kernel_size,就可以将Input展开为
T
e
n
s
o
r
[
B
,
C
i
n
×
(
K
×
K
)
,
M
×
M
]
Tensor[B,C_{in}\times(K\times K),M\times M]
Tensor[B,Cin×(K×K),M×M]的形式。
input_unf = nn.Unfold(kernel_size=K)(input)
然后通过view
将Input转变为
T
e
n
s
o
r
[
B
,
C
i
n
,
K
×
K
,
M
,
M
]
Tensor[B,C_{in},K\times K,M,M]
Tensor[B,Cin,K×K,M,M]的形式。
input_unf = input_unf.view((B, Cin, -1, M, M))
同样通过view
将Kernel转变为
T
e
n
s
o
r
[
C
o
u
t
,
C
i
n
,
K
×
K
]
Tensor[C_{out},C_{in},K\times K]
Tensor[Cout,Cin,K×K]的形式。
kernel_view = kernel.view((Cout, Cin, K * K))
而输出Output是 T e n s o r [ B , C o u t , M , M ] Tensor[B,C_{out},M,M] Tensor[B,Cout,M,M]的形式。
在这里就可以直接用Einstein求和标记将卷积运算写出来了:
代码为
output = torch.einsum("ijklm,njk->inlm", input_unf, kernel_view)
如何计算梯度?
这部分求导的推导是我自己在草稿纸上完成的,后面经过一些验证应该或许可以保证是正确的。
为了能够用Pytorch自带的gradcheck
来验证backward梯度计算的正确性,我们有必要对每个输入参数都进行求导,假设最终的Loss函数结果为
L
L
L(是一个标量),我们需要计算对输入Input的导数(
∂
L
/
∂
I
n
p
u
t
\partial{L}/\partial{Input}
∂L/∂Input)以及对卷积核Kernel的导数(
∂
L
/
∂
K
e
r
n
e
l
\partial{L}/\partial{Kernel}
∂L/∂Kernel)。
为了方便推导,先不考虑batch和channel,也就是Input, Kernel, Output都是二维的。
Kernel的梯度
根据链式求导法则我们可以将此导数(偏导)写作
∂
L
∂
K
e
r
n
e
l
=
∂
L
∂
O
u
t
p
u
t
∂
O
u
t
p
u
t
∂
K
e
r
n
e
l
,
\frac{\partial{L}}{\partial{Kernel}}=\frac{\partial{L}}{\partial{Output}}\frac{\partial{Output}}{\partial{Kernel}},
∂Kernel∂L=∂Output∂L∂Kernel∂Output,
式中
∂
L
∂
O
u
t
p
u
t
\frac{\partial{L}}{\partial{Output}}
∂Output∂L已知(backward过程中会作为参数一直传下去),也就是计算图中当前卷积算子后面的链路所有梯度的累乘,其size与Output一致。
那么问题就是求Output对Kernel的偏导,我们用一个简单的例子来推导:
可以发现,
∂
L
∂
k
11
\frac{\partial{L}}{\partial{k_{11}}}
∂k11∂L竟然就是
∂
L
∂
O
u
t
p
u
t
\frac{\partial{L}}{\partial{Output}}
∂Output∂L和Input矩阵的左上子矩阵的点积,对于其它的
∂
L
∂
k
i
j
\frac{\partial{L}}{\partial{k_{ij}}}
∂kij∂L也是同理,因此我们可以得到结论:
∂
L
∂
K
e
r
n
e
l
=
I
n
p
u
t
∗
∂
L
∂
O
u
t
p
u
t
\frac{\partial{L}}{\partial{Kernel}}=Input * \frac{\partial{L}}{\partial{Output}}
∂Kernel∂L=Input∗∂Output∂L
也就是说,Kernel的梯度,就是以Output的梯度作为卷积核,对Input卷积的结果。
Input的梯度
同样,Input的梯度可以写作
∂
L
∂
I
n
p
u
t
=
∂
L
∂
O
u
t
p
u
t
∂
O
u
t
p
u
t
∂
I
n
p
u
t
,
\frac{\partial{L}}{\partial{Input}}=\frac{\partial{L}}{\partial{Output}}\frac{\partial{Output}}{\partial{Input}},
∂Input∂L=∂Output∂L∂Input∂Output,
式中
∂
L
∂
O
u
t
p
u
t
\frac{\partial{L}}{\partial{Output}}
∂Output∂L已知,同样沿用上面的例子来推导:
我们可以发现,把
∂
L
∂
O
u
t
p
u
t
\frac{\partial{L}}{\partial{Output}}
∂Output∂L适当的0填充后,以旋转180°的Kernel做卷积运算,就得到了
∂
L
∂
I
n
p
u
t
\frac{\partial{L}}{\partial{Input}}
∂Input∂L。公式可以写作(可能不太规范):
∂
L
∂
I
n
p
u
t
=
∂
L
∂
O
u
t
p
u
t
∗
^
(
K
e
r
n
e
l
)
旋转
180
°
\frac{\partial{L}}{\partial{Input}}=\frac{\partial{L}}{\partial{Output}} \hat{*} (Kernel)^{旋转180°}
∂Input∂L=∂Output∂L∗^(Kernel)旋转180°
其中
∗
^
\hat{*}
∗^表示反卷积。
因此Input的梯度计算方式可以表述为:Input的梯度,就是以旋转180°的Kernel作为卷积核,对 ∂ L ∂ O u t p u t \frac{\partial{L}}{\partial{Output}} ∂Output∂L反卷积的结果。
自定义卷积算子
本文的一个很大目的,就是让我自己学会怎么扩展Pytorch的算子,从官方文档了解到,需要实现一个继承torch.autograd.Function
的函数,并且实现forward
和backward
静态函数,才能适应Pytorch的自动求导框架,有一些需要注意的细节:
forward
和backward
函数的第一个参数都是ctx
,就是context的意思,与self
类似,一般如果在backward过程中要用到forward的参数,在forward时就要调用ctx.save_for_backward()
保存起来;forward
有多少个输入,backward
就要有多少个输出,这个看计算图就能明白了,如果不需要求梯度的入边,可以返回None
;
梯度求解
前面在定义卷积运算时,都是考虑了Batch和Channel的,而在推导对Input和Kernel的梯度时,却为了方便没有考虑这两个参数。实际上在实现时,要特别注意每个数据的view
的每个维度之间的关系。
例如我这里定义的:
I
n
p
u
t
:
T
e
n
s
o
r
[
B
,
C
i
n
,
N
,
N
]
K
e
r
n
e
l
:
T
e
n
s
o
r
[
C
o
u
t
,
C
i
n
,
K
,
K
]
O
u
t
p
u
t
:
T
e
n
s
o
r
[
B
,
C
o
u
t
,
M
,
M
]
\begin{aligned} Input&: Tensor[B,C_{in},N,N] \\ Kernel&: Tensor[C_{out},C_{in},K,K] \\ Output&: Tensor[B,C_{out},M,M] \end{aligned}
InputKernelOutput:Tensor[B,Cin,N,N]:Tensor[Cout,Cin,K,K]:Tensor[B,Cout,M,M]
在求Kernel的梯度时,根据公式
∂
L
∂
K
e
r
n
e
l
=
I
n
p
u
t
∗
∂
L
∂
O
u
t
p
u
t
\frac{\partial{L}}{\partial{Kernel}}=Input * \frac{\partial{L}}{\partial{Output}}
∂Kernel∂L=Input∗∂Output∂L ,这里的维度是
I
n
p
u
t
:
T
e
n
s
o
r
[
B
,
C
i
n
,
N
,
N
]
∂
L
∂
O
u
t
p
u
t
:
T
e
n
s
o
r
[
B
,
C
o
u
t
,
M
,
M
]
∂
L
∂
K
e
r
n
e
l
:
T
e
n
s
o
r
[
C
o
u
t
,
C
i
n
,
K
,
K
]
\begin{aligned} Input&: Tensor[B,C_{in},N,N] \\ \frac{\partial{L}}{\partial{Output}}&: Tensor[B,C_{out},M,M] \\ \frac{\partial{L}}{\partial{Kernel}}&: Tensor[C_{out},C_{in},K,K] \end{aligned}
Input∂Output∂L∂Kernel∂L:Tensor[B,Cin,N,N]:Tensor[B,Cout,M,M]:Tensor[Cout,Cin,K,K]
因此我们需要先把
I
n
p
u
t
Input
Input的01维交换(transpose),再把
∂
L
∂
O
u
t
p
u
t
\frac{\partial{L}}{\partial{Output}}
∂Output∂L的01维交换,然后再做卷积,得到的结果还要把01维交换,才能得到
∂
L
∂
K
e
r
n
e
l
\frac{\partial{L}}{\partial{Kernel}}
∂Kernel∂L。代码写作:
input_ = torch.transpose(input, 0, 1)
grad_output_ = torch.transpose(grad_output, 0, 1)
grad_weight = MyConv2dFunc.conv2d(input_, grad_output_).transpose(0, 1)
求Input的梯度也是类似。
代码
class MyConv2dFunc(torch.autograd.Function):
@staticmethod
def conv2d(input: Tensor, kernel: Tensor) -> Tensor:
"""
卷积运算
Output = Input * Kernel
:param input: Tensor[B, Cin, N, N]
:param kernel: Tensor[Cout, Cin, K, K]
:return: Tensor[B, Cout, M, M], M=N-K+1
"""
B = input.shape[0]
Cin = input.shape[1]
N = input.shape[2]
Cout = kernel.shape[0]
K = kernel.shape[2]
M = N - K + 1
input_unf = nn.Unfold(kernel_size=K)(input)
input_unf = input_unf.view((B, Cin, -1, M, M))
kernel_view = kernel.view((Cout, Cin, K * K))
output = torch.einsum("ijklm,njk->inlm", input_unf, kernel_view)
return output
@staticmethod
def forward(ctx, input, weight):
ctx.save_for_backward(input, weight)
output = MyConv2dFunc.conv2d(input, weight)
return output
@staticmethod
def backward(ctx, grad_output):
input, weight = ctx.saved_tensors
grad_input = grad_weight = None
if grad_output is None:
return None, None
if ctx.needs_input_grad[0]:
# 反卷积
gop = nn.ZeroPad2d(weight.shape[2] - 1)(grad_output)
kk = torch.rot90(weight, 2, (2, 3)) # 旋转180度
kk = torch.transpose(kk, 0, 1)
grad_input = MyConv2dFunc.conv2d(gop, kk)
if ctx.needs_input_grad[1]:
input_ = torch.transpose(input, 0, 1)
grad_output_ = torch.transpose(grad_output, 0, 1)
grad_weight = MyConv2dFunc.conv2d(input_, grad_output_).transpose(0, 1)
return grad_input, grad_weight
正确性验证
torch.autograd.gradcheck
提供了检验梯度运算正确性的工具,它的原理是,给定输入,用你写的算子的backward计算一个output和input的雅各比矩阵,然后再用有限差分的方法计算一个数值解,然后对比这两个结果是否一致。
验证上面的MyConv2dFunc
算子的正确性:
input = (torch.rand((2, 4, 10, 10), requires_grad=True, dtype=torch.double),
torch.rand((6, 4, 5, 5), requires_grad=True, dtype=torch.double))
test = torch.autograd.gradcheck(MyConv2dFunc.apply, input)
print(test)
输出为True
。
自定义卷积层模型
需要继承nn.Module
,并且用nn.Parameter
保存权重,也就是卷积核。还要实现forward
方法。
class MyConv2d(nn.Module):
def __init__(self, in_channels, out_channels, kernel_size: tuple):
super(MyConv2d, self).__init__()
self.in_channels = in_channels
self.out_channels = out_channels
self.kernel_size = kernel_size
# Parameters
self.weight = nn.Parameter(torch.empty(out_channels, in_channels, kernel_size[0], kernel_size[1]))
nn.init.uniform_(self.weight, -0.1, 0.1)
def forward(self, x):
return MyConv2dFunc.apply(x, self.weight)
def extra_repr(self):
return 'MyConv2d: in_channels={}, out_channels={}, kernel_size={}'.format(
self.in_channels, self.out_channels, self.kernel_size
)
基于MNIST的测试
使用的卷积神经网络模型为LeNet:
CNN(
(layer1): Sequential(
(0): Conv2d(1, 32, kernel_size=(3, 3), stride=(1, 1))
(1): ReLU()
(2): Conv2d(32, 64, kernel_size=(3, 3), stride=(1, 1))
(3): BatchNorm2d(64, eps=1e-05, momentum=0.1, affine=True, track_running_stats=True)
(4): ReLU()
(5): MaxPool2d(kernel_size=2, stride=2, padding=0, dilation=1, ceil_mode=False)
)
(flatten): Flatten(start_dim=1, end_dim=-1)
(fc): Sequential(
(0): Linear(in_features=9216, out_features=256, bias=True)
(1): ReLU()
(2): Linear(in_features=256, out_features=10, bias=True)
)
)
任务是对MNIST手写体数字进行分类。
首先用Pytorch自带的Conv、Linear这些网络层搭建然后训练,然后把网络中的Conv2d
替换为我写的MyConv2d
做同样的训练,得到的结果如下(5个epoch, CUDA):
Accuracy | time cost(s) | |
---|---|---|
nn.Conv2d | 99.2% | 33.72 |
MyConv2d | 99.1% | 76.49 |