既有适合小白学习的零基础资料,也有适合3年以上经验的小伙伴深入学习提升的进阶课程,涵盖了95%以上大数据知识点,真正体系化!
由于文件比较多,这里只是将部分目录截图出来,全套包含大厂面经、学习笔记、源码讲义、实战项目、大纲路线、讲解视频,并且后续会持续更新
然后加入‘bird’对象,布隆过滤器的内容并没有改变,因为‘bird’和‘fish’恰好拥有相同的哈希。
最后我们检查一堆对象(‘dog’, ‘fish’, ‘cat’, ‘bird’, ‘duck’, ‘emu’)是不是已经被索引了。结果发现‘duck’返回True,2而‘emu’返回False。因为‘duck’的哈希恰好和‘dog’是一样的。
分词
下面一步我们要实现分词。 分词的目的是要把我们的文本数据分割成可搜索的最小单元,也就是词。这里我们主要针对英语,因为中文的分词涉及到自然语言处理,比较复杂,而英文基本只要用标点符号就好了。
下面我们看看分词的代码:
def major_segments(s):
"""
Perform major segmenting on a string. Split the string by all of the major
breaks, and return the set of everything found. The breaks in this implementation
are single characters, but in Splunk proper they can be multiple characters.
A set is used because ordering doesn't matter, and duplicates are bad.
"""
major_breaks = ' '
last = -1
results = set()
# enumerate() will give us (0, s[0]), (1, s[1]), ...
for idx, ch in enumerate(s):
if ch in major_breaks:
segment = s[last+1:idx]
results.add(segment)
last = idx
# The last character may not be a break so always capture
# the last segment (which may end up being "", but yolo)
segment = s[last+1:]
results.add(segment)
return results
主要分割
主要分割使用空格来分词,实际的分词逻辑中,还会有其它的分隔符。例如Splunk的缺省分割符包括以下这些,用户也可以定义自己的分割符。
] < > ( ) { } | ! ; , ’ " * \n \r \s \t & ? + %21 %26 %2526 %3B %7C %20 %2B %3D – %2520 %5D %5B %3A %0A %2C %28 %29
def minor_segments(s):
"""
Perform minor segmenting on a string. This is like major
segmenting, except it also captures from the start of the
input to each break.
"""
minor_breaks = '_.'
last = -1
results = set()
for idx, ch in enumerate(s):
if ch in minor_breaks:
segment = s[last+1:idx]
results.add(segment)
segment = s[:idx]
results.add(segment)
last = idx
segment = s[last+1:]
results.add(segment)
results.add(s)
return results
次要分割
次要分割和主要分割的逻辑类似,只是还会把从开始部分到当前分割的结果加入。例如“1.2.3.4”的次要分割会有1,2,3,4,1.2,1.2.3
def segments(event):
"""Simple wrapper around major_segments / minor_segments"""
results = set()
for major in major_segments(event):
for minor in minor_segments(major):
results.add(minor)
return results
分词的逻辑就是对文本先进行主要分割,对每一个主要分割在进行次要分割。然后把所有分出来的词返回。
我们看看这段 code是如何运行的:
for term in segments('src_ip = 1.2.3.4'):
print term
src
1.2
1.2.3.4
src_ip
3
1
1.2.3
ip
2
=
4
搜索
好了,有个分词和布隆过滤器这两个利器的支撑后,我们就可以来实现搜索的功能了。
上代码:
class Splunk(object):
def __init__(self):
self.bf = Bloomfilter(64)
self.terms = {} # Dictionary of term to set of events
self.events = []
def add_event(self, event):
"""Adds an event to this object"""
# Generate a unique ID for the event, and save it
event_id = len(self.events)
self.events.append(event)
# Add each term to the bloomfilter, and track the event by each term
for term in segments(event):
self.bf.add_value(term)
if term not in self.terms:
self.terms[term] = set()
self.terms[term].add(event_id)
def search(self, term):
"""Search for a single term, and yield all the events that contain it"""
# In Splunk this runs in O(1), and is likely to be in filesystem cache (memory)
if not self.bf.might_contain(term):
return
# In Splunk this probably runs in O(log N) where N is the number of terms in the tsidx
if term not in self.terms:
return
for event_id in sorted(self.terms[term]):
yield self.events[event_id]
- Splunk代表一个拥有搜索功能的索引集合
- 每一个集合中包含一个布隆过滤器,一个倒排词表(字典),和一个存储所有事件的数组
- 当一个事件被加入到索引的时候,会做以下的逻辑
- 为每一个事件生成一个unqie id,这里就是序号
- 对事件进行分词,把每一个词加入到倒排词表,也就是每一个词对应的事件的id的映射结构,注意,一个词可能对应多个事件,所以倒排表的的值是一个Set。倒排表是绝大部分搜索引擎的核心功能。
- 当一个词被搜索的时候,会做以下的逻辑
- 检查布隆过滤器,如果为假,直接返回
- 检查词表,如果被搜索单词不在词表中,直接返回
- 在倒排表中找到所有对应的事件id,然后返回事件的内容
我们运行下看看把:
s = Splunk()
s.add_event('src_ip = 1.2.3.4')
s.add_event('src_ip = 5.6.7.8')
s.add_event('dst_ip = 1.2.3.4')
for event in s.search('1.2.3.4'):
print event
print '-'
for event in s.search('src_ip'):
print event
print '-'
for event in s.search('ip'):
print event
src_ip = 1.2.3.4
dst_ip = 1.2.3.4
-
src_ip = 1.2.3.4
src_ip = 5.6.7.8
-
src_ip = 1.2.3.4
src_ip = 5.6.7.8
dst_ip = 1.2.3.4
是不是很赞!
更复杂的搜索
更进一步,在搜索过程中,我们想用And和Or来实现更复杂的搜索逻辑。
上代码:
class SplunkM(object):
def __init__(self):
self.bf = Bloomfilter(64)
self.terms = {} # Dictionary of term to set of events
self.events = []
def add_event(self, event):
"""Adds an event to this object"""
# Generate a unique ID for the event, and save it
event_id = len(self.events)
self.events.append(event)
# Add each term to the bloomfilter, and track the event by each term
for term in segments(event):
self.bf.add_value(term)
if term not in self.terms:
self.terms[term] = set()
self.terms[term].add(event_id)
def search_all(self, terms):
"""Search for an AND of all terms"""
# Start with the universe of all events...
results = set(range(len(self.events)))
for term in terms:
# If a term isn't present at all then we can stop looking
if not self.bf.might_contain(term):
return
if term not in self.terms:
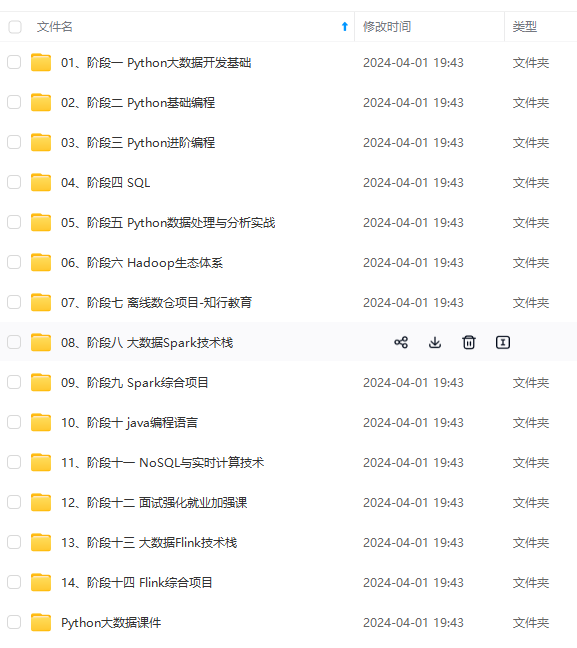
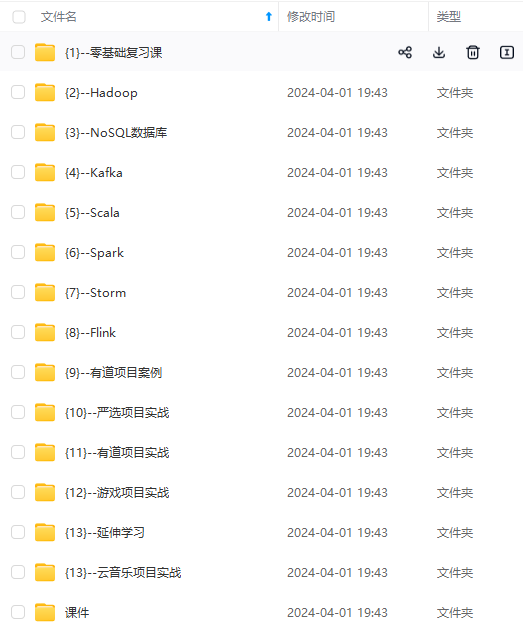
**网上学习资料一大堆,但如果学到的知识不成体系,遇到问题时只是浅尝辄止,不再深入研究,那么很难做到真正的技术提升。**
**[需要这份系统化资料的朋友,可以戳这里获取](https://bbs.youkuaiyun.com/forums/4f45ff00ff254613a03fab5e56a57acb)**
**一个人可以走的很快,但一群人才能走的更远!不论你是正从事IT行业的老鸟或是对IT行业感兴趣的新人,都欢迎加入我们的的圈子(技术交流、学习资源、职场吐槽、大厂内推、面试辅导),让我们一起学习成长!**
..(img-FlxZRLTS-1715459943224)]
**网上学习资料一大堆,但如果学到的知识不成体系,遇到问题时只是浅尝辄止,不再深入研究,那么很难做到真正的技术提升。**
**[需要这份系统化资料的朋友,可以戳这里获取](https://bbs.youkuaiyun.com/forums/4f45ff00ff254613a03fab5e56a57acb)**
**一个人可以走的很快,但一群人才能走的更远!不论你是正从事IT行业的老鸟或是对IT行业感兴趣的新人,都欢迎加入我们的的圈子(技术交流、学习资源、职场吐槽、大厂内推、面试辅导),让我们一起学习成长!**