一、 基础知识
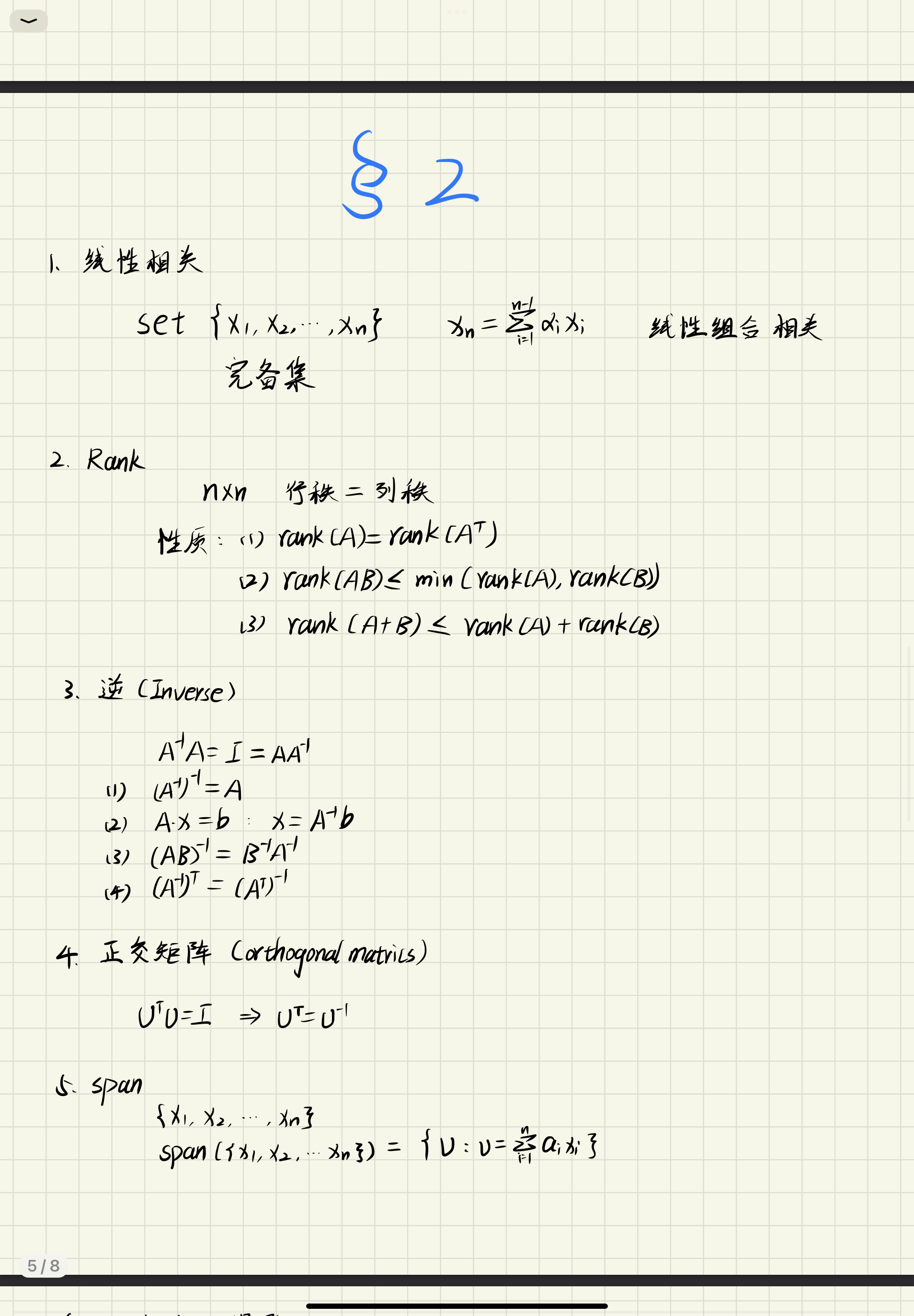
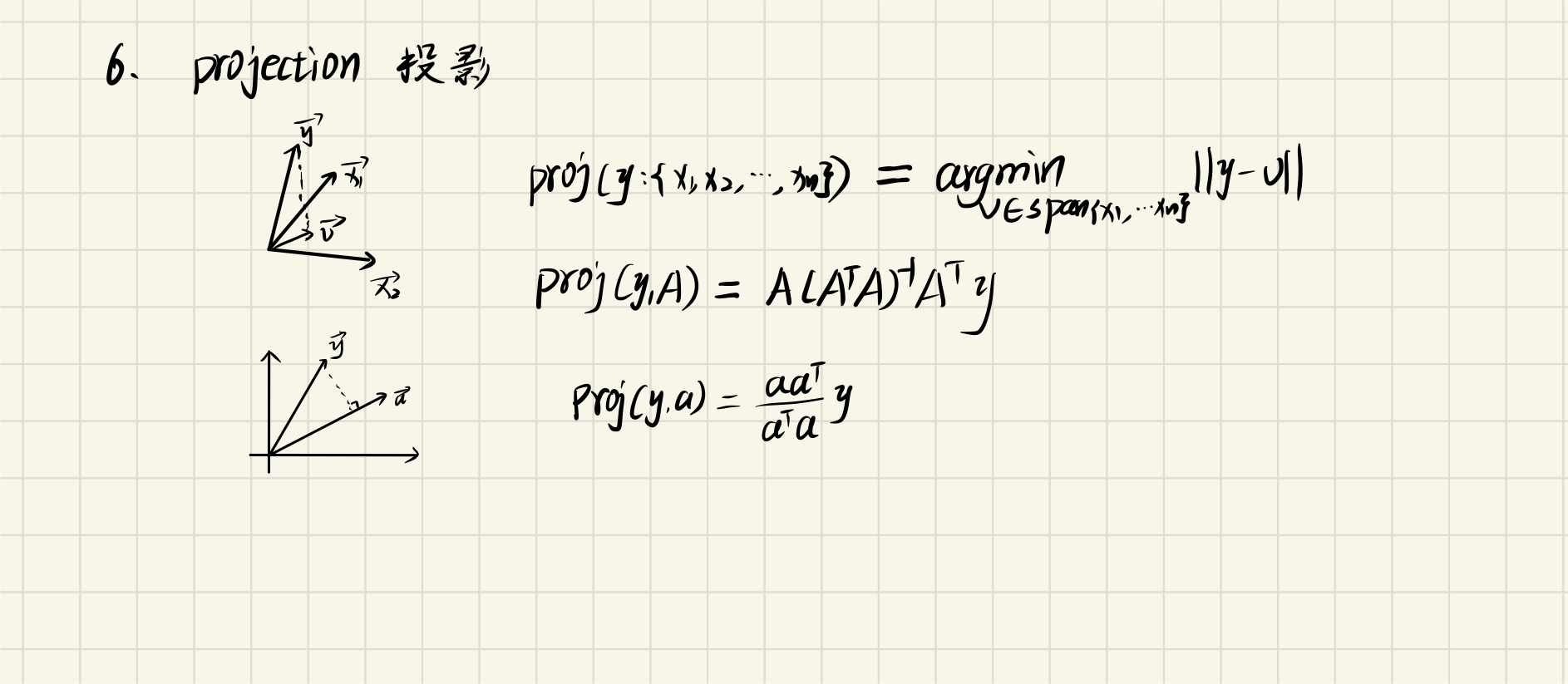
二、 线性回归和梯度下降算法
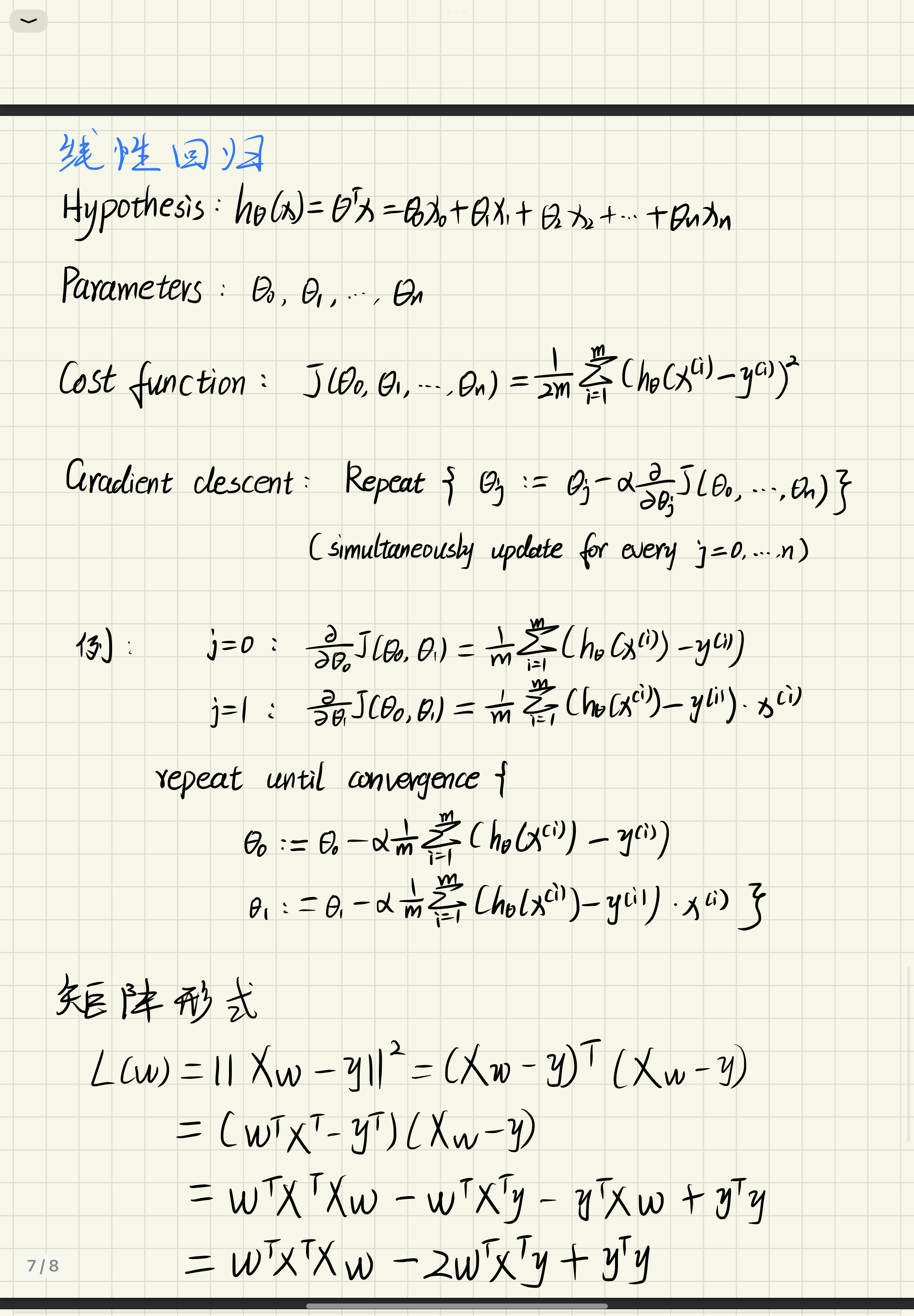
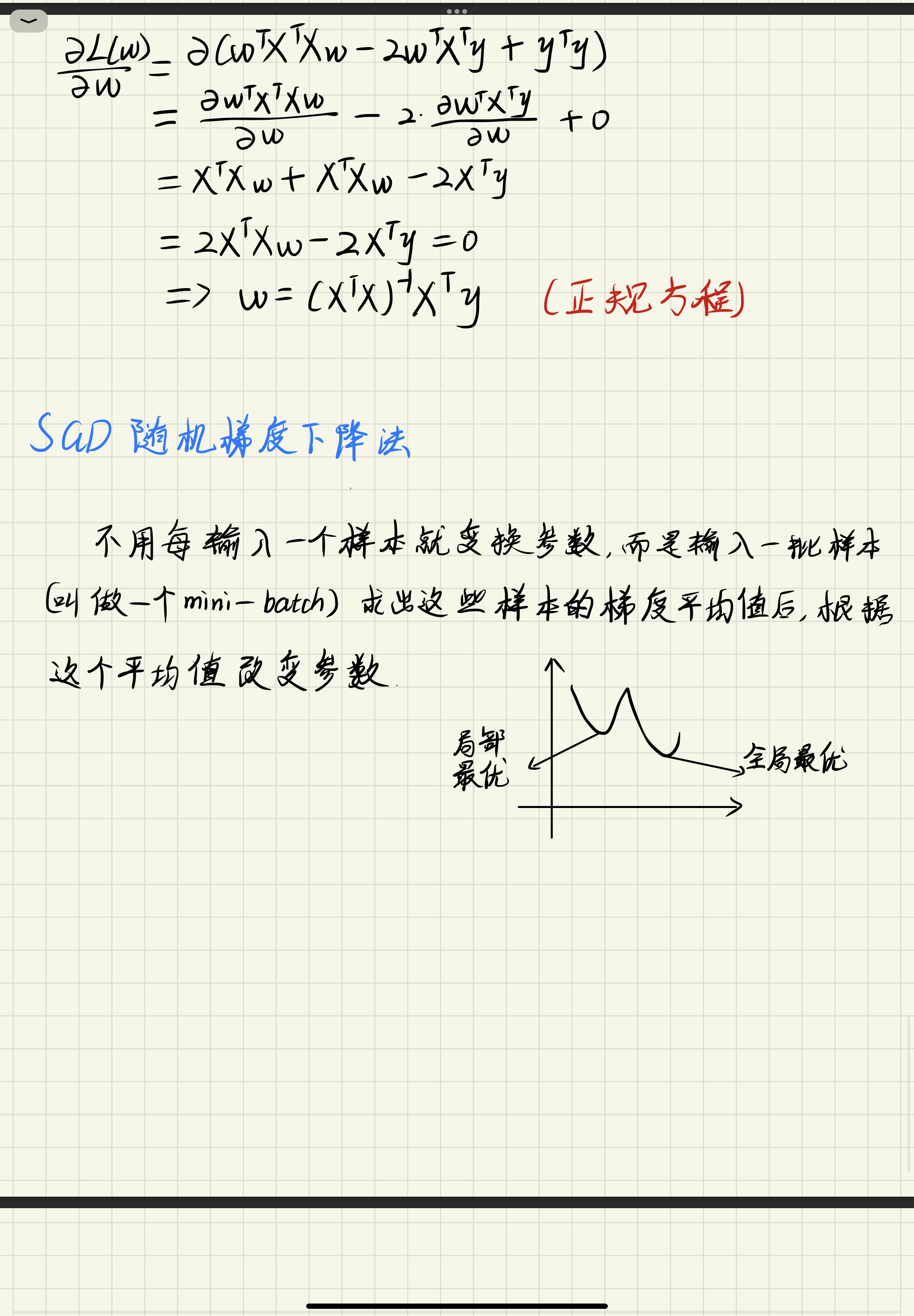
三、 代码
数据集:200万条训练数据 该数据集为一个出租车数据集的部分数据

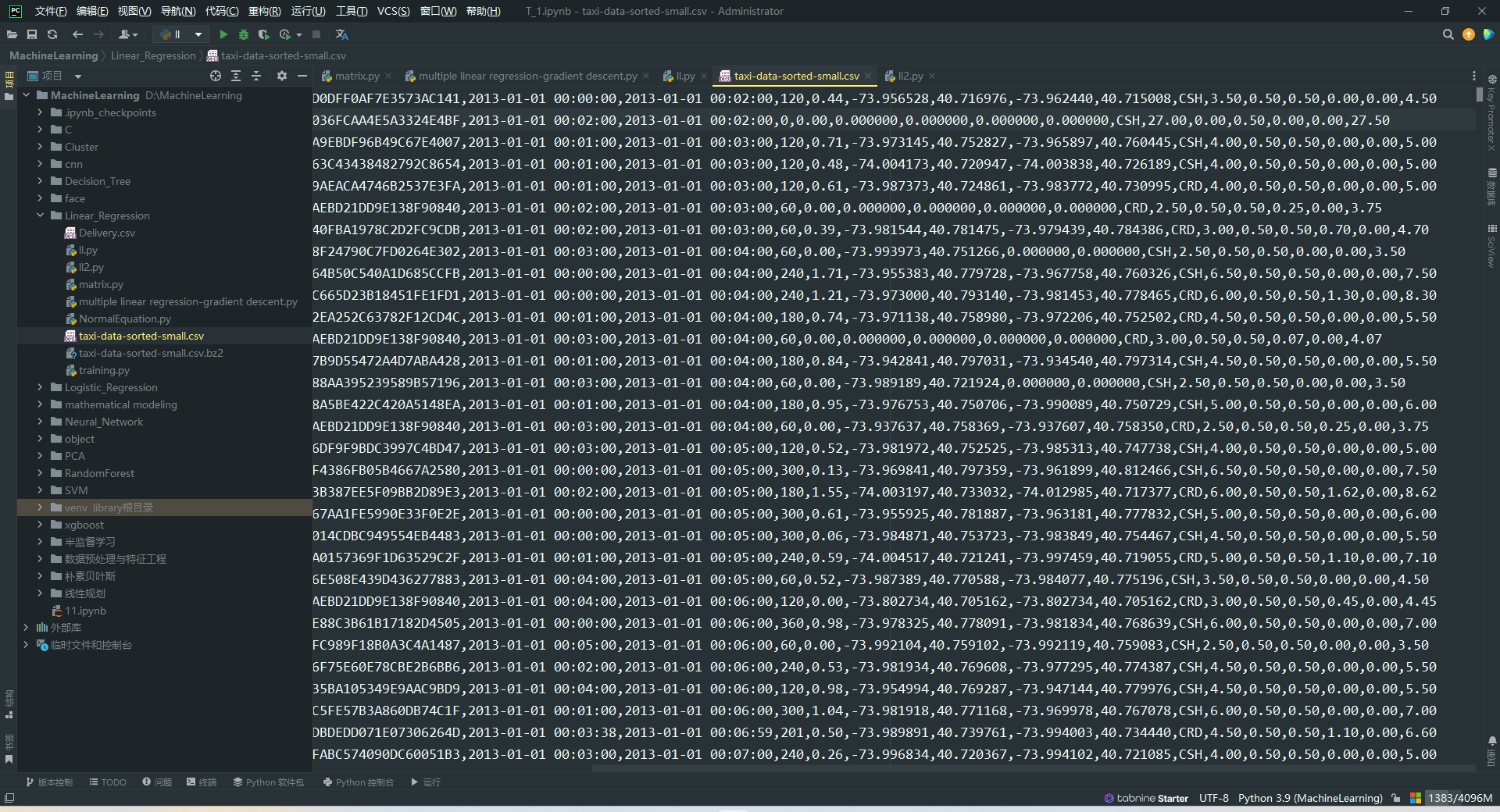
通过for循环实现损失函数梯度下降
import numpy as np
import time
# 读入数据
data = np.matrix(np.loadtxt("taxi-data-sorted-small.csv", delimiter=",", usecols=(4, 5, 11, 12, 16)))
# 切分数据
x_data = np.insert(data[:, :-1], 0, 1, axis=1) # 第0列插入1 对应于参数 theta0 即常数项
y_data = data[:, -1]
# 学习率learning rate
lr = 0.0001
# 参数
theta0 = 0
theta1 = 0
theta2 = 0
# 最大迭代次数
epochs = 2
# 最小二乘法
def compute_error(theta0, theta1, theta2, x_data, y_data):
totalError = 0
for i in range(0, len(x_data)):
totalError += (y_data[i] - (theta1 * x_data[i, 0] + theta2 * x_data[i, 1] + theta0)) ** 2
return totalError / (float(len(x_data))*2)
def gradient_descent_runner(x_data, y_data, theta0, theta1, theta2, lr, epochs):
# 计算总数据量
m = float(len(x_data))
# 循环epochs次
for i in range(epochs):
theta0_grad = 0
theta1_grad = 0
theta2_grad = 0
# 计算梯度的总和再求平均
for j in range(0, len(x_data)):
theta0_grad += -(1 / m) * (y_data[j] - (theta1 * x_data[j, 0] + theta2 * x_data[j, 1] + theta0))
theta1_grad += -(1 / m) * x_data[j, 0] * (
y_data[j] - (theta1 * x_data[j, 0] + theta2 * x_data[j, 1] + theta0))
theta2_grad += -(1 / m) * x_data[j, 1] * (
y_data[j] - (theta1 * x_data[j, 0] + theta2 * x_data[j, 1] + theta0))
# 更新参数
theta0 = theta0 - (lr * theta0_grad)
theta1 = theta1 - (lr * theta1_grad)
theta2 = theta2 - (lr * theta2_grad)
return theta0, theta1, theta2
print("Starting theta0 = {0}, theta1 = {1}, theta2 = {2}, error = {3}".
format(theta0, theta1, theta2, compute_error(theta0, theta1, theta2, x_data, y_data)))
print("Running...")
start = time.time()
theta0, theta1, theta2 = gradient_descent_runner(x_data, y_data, theta0, theta1, theta2, lr, epochs)
print('epoch=2 训练运行时间:', time.time()-start)
print("After {0} iterations theta0 = {1}, theta1 = {2}, theta2 = {3}, error = {4}".
format(epochs, theta0, theta1, theta2, compute_error(theta0, theta1, theta2, x_data, y_data)))
运行结果:

2. 通过numpy矩阵优化
import numpy as np
import time
def compute_error(theta0, x, y):
# 最小二乘法
inner = np.power((x * theta0) - y, 2)
return np.sum(inner) / (2 * len(x))
def gradient_descent_runner(x, y, theta0, l, epochs):
for i in range(epochs):
theta0 -= l / len(x) * x.T * ((x * theta0) - y)
return theta0
# 读入数据
data = np.matrix(np.loadtxt("taxi-data-sorted-small.csv", delimiter=",", usecols=(4, 5, 11, 12, 16)))
# 切分数据
x_data = np.insert(data[:, :-1], 0, 1, axis=1) # 第0列插入1 对应于参数 theta0 即常数项
y_data = data[:, -1]
lr = 0.0000001 # 学习率learning rate
theta = np.matrix([[0], [0], [0], [0], [0]],dtype='float64') # 参数
epochs0 = 100 # 最大迭代次数
print("Starting theta0 = {0}, theta1 = {1}, theta2 = {2}, theta3 = {3}, theta4 = {4}, \n error = {5}".
format(theta[0,0], theta[1,0], theta[2,0], theta[3,0], theta[4,0], compute_error(theta, x_data, y_data)))
print("Running...")
start = time.time()
theta = gradient_descent_runner(x_data, y_data, theta, lr, epochs0) # 梯度下降
print('epoch=100 通过numpy矩阵优化的训练运行时间:', time.time()-start)
print("After {0} iterations theta0 = {1}, theta1 = {2}, theta2 = {3}, theta3 = {4}, theta4 = {5}, \n error = {6}".
format(epochs0, theta[0,0], theta[1,0], theta[2,0], theta[3,0], theta[4,0], compute_error(theta, x_data, y_data)))
运行结果:

3. 正规方程
import numpy as np
import time
def compute_error(theta0, x, y):
# 最小二乘法
inner = np.power((x * theta0) - y, 2)
return np.sum(inner) / (2 * len(x))
# 读入数据
data = np.matrix(np.loadtxt("taxi-data-sorted-small.csv", delimiter=",", usecols=(4, 5, 11, 12, 16)))
# 切分数据
x_data = np.insert(data[:, :-1], 0, 1, axis=1) # 第0列插入1 对应于参数 theta0 即常数项
y_data = data[:, -1]
print("Running...")
start = time.time()
k = np.linalg.pinv(x_data.T * x_data) * x_data.T * y_data # 正规方程
print('正规方程训练时间:', time.time()-start)
print("After iterations theta0 = {0}, theta1 = {1}, theta2 = {2}, theta3 = {3}, theta4 = {4},\n error = {5}".
format(k[0,0], k[1,0], k[2,0], k[3,0], k[4,0], compute_error(k[:5], x_data, y_data)))
运行结果:

四、总结
for循环的梯度下降 epoch=2时,训练用时用了343.7秒,损失函数值为3.13924235e+09
矩阵优化后的 epoch=100时,训练用时用了8.09秒,损失函数值为 27.197452
正规方程 训练用时用了0.23秒,损失函数值为2.107677
综上: 正规方程>矩阵优化后>for循环的梯度下降