文章目录
- Introduction
- Algorithm 1: PAWCS ***
- Algorithm 2: SuBSENSE ****
- Algorithm 3: LOBSTER *
- Algorithm 4: WeightedMovingVariance ***
- Algorithm 5: CodeBook ****
- Algorithm 6: SigmaDelta ****
- Algorithm 7: WeightedMovingMean ****
- Algorithm 8: AdaptiveBackgroundLearning *****
- Algorithm 9: MixtureOfGaussianV2 *****
- Algorithm 10:ViBe **
- Algorithm 11:TwoPoints *****
- Algorithm 12: AdaptiveSelectiveBackgroundLearning **
- Algorithm 13: FrameDifference ****
- Algorithm 14: StaticFrameDifference **
Introduction
I have tested the whole algorithms of bgslibrary
afternoon, which include 14 background subtraction methods. And I make a summary for it.
Top:
Algorithm 2: SuBSENSE ****
Algorithm 5: CodeBook ****
Algorithm 6: SigmaDelta ****
Algorithm 7: WeightedMovingMean ****
Algorithm 8: AdaptiveBackgroundLearning *****
Algorithm 9: MixtureOfGaussianV2 *****
Algorithm 11:TwoPoints *****
Algorithm 13: FrameDifference ****
Algorithm 1: PAWCS ***
This algorithm is the slowest one among all the methods, each frame should cost 2.77 s (Equivalent to 0.36 Hz) to subtract background. What amazing about this algorithm is that The Object of interest will be vanished graudually after 600-frames, so it causes a wonderful background-subtract result.
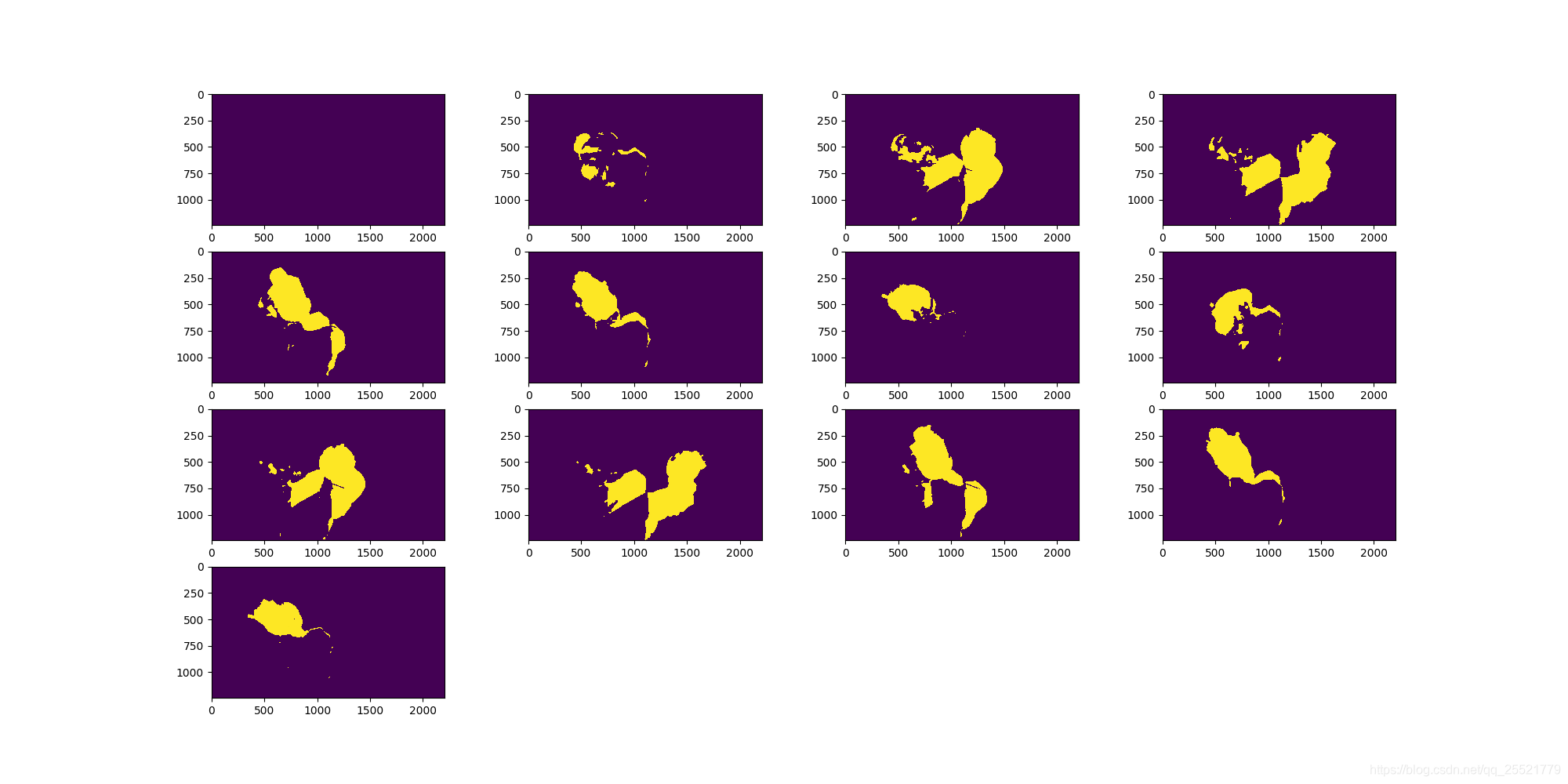
Algorithm 2: SuBSENSE ****
this algorithm is very slow (1.43 s, Equivalent to 0.70 Hz), and its results are not satisfied. the admirable point is that the background model constructed by SuBSENSE is amazing, it can almost vanishing the object of interest in background model. And SuBSENSE is nearly twice as fast as the PAWCS method.
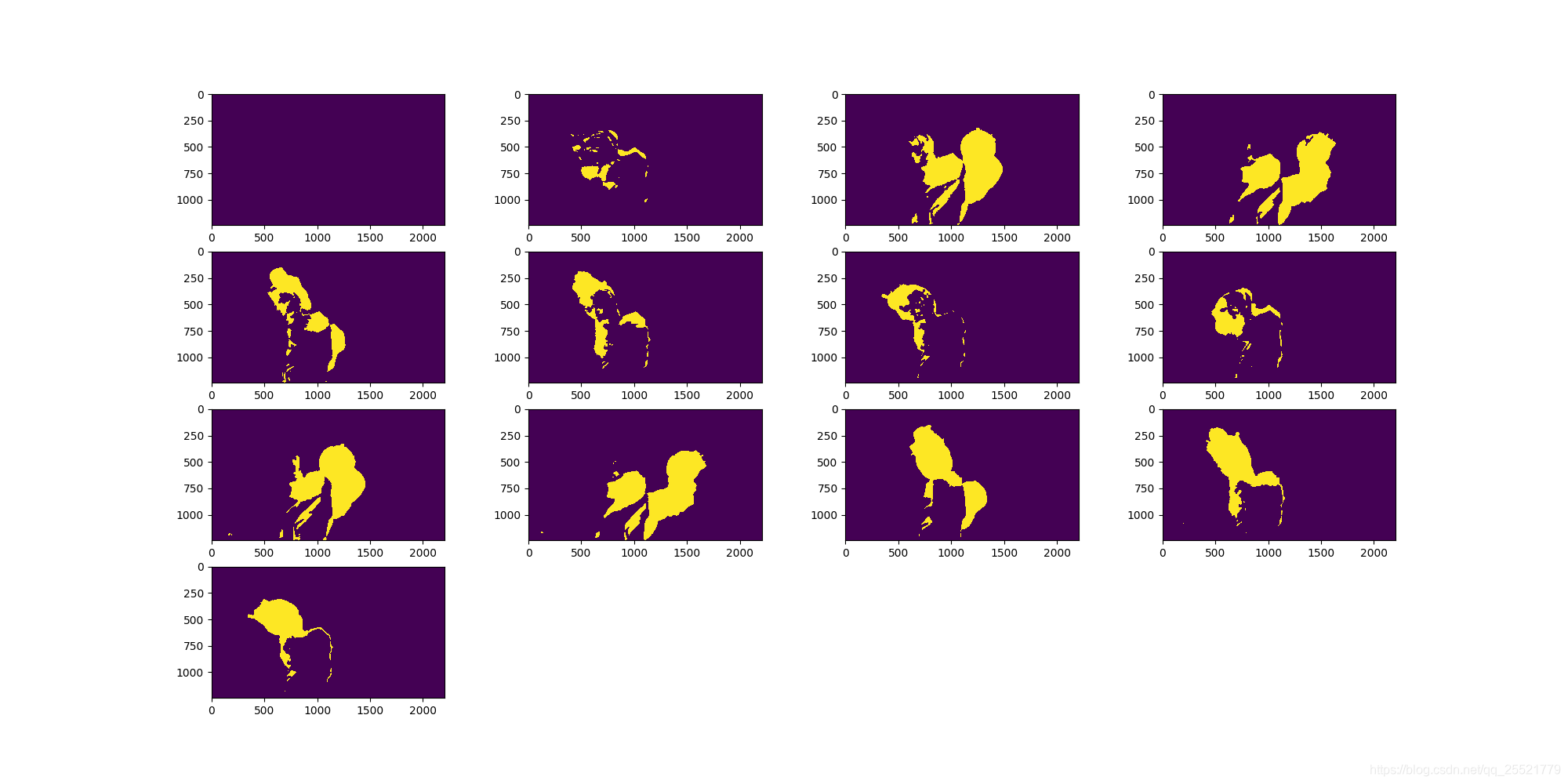
Algorithm 3: LOBSTER *
This algorithm is also extreamly slow (859.00 ms, 1.16 Hz), and its result is terrible. With program performing, the object of interest in background model becomes blurred, which means the background model is effective gradually.
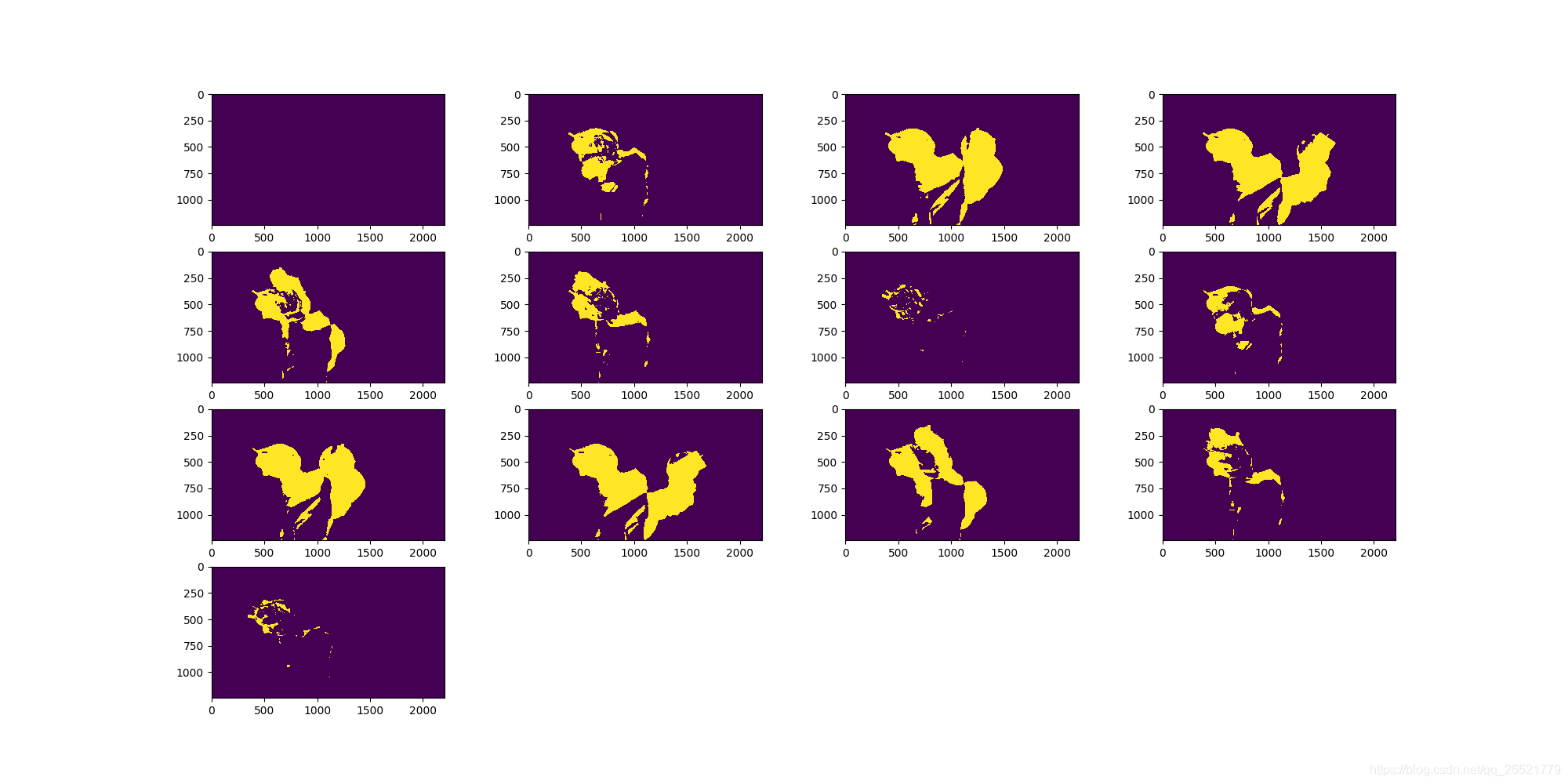
Algorithm 4: WeightedMovingVariance ***
The average processing time of this algorithm is 152.01 ms (Equivalent to 6.57 Hz), and the result of it is not bad. However it would unwork when the object of interest is moving very slow, even static.
Algorithm 5: CodeBook ****
The average processing time of this algorithm is 71.94 ms(Equivalent to 13.90 Hz), its results are the most of quanlity. At start, It may not handle the fast moving object. With the sequence increaing, it comes to a better state.
Algorithm 6: SigmaDelta ****
The average processing time of this algorithm is 77.79 ms(Equivalent to 12.86 Hz). The result of this algorithm is worse than codebook. It is clear to see that background model still contains particial object of interest, which have shown in the background subtracted results.
Algorithm 7: WeightedMovingMean ****
The average processing time of this method is 76.45 ms (Equivalent to 13.08 Hz). The result of WeightedMovingMean is similar to the WeightedMovingVariance, but this algorithm is twice faster than the latter one. The background model constructed by WeightedMovingMean seems to be obtained by weighted average previous frames, it is clear to see the afterglow of moving object. This method will also unwork when the object of interest moves slowly or stays still.
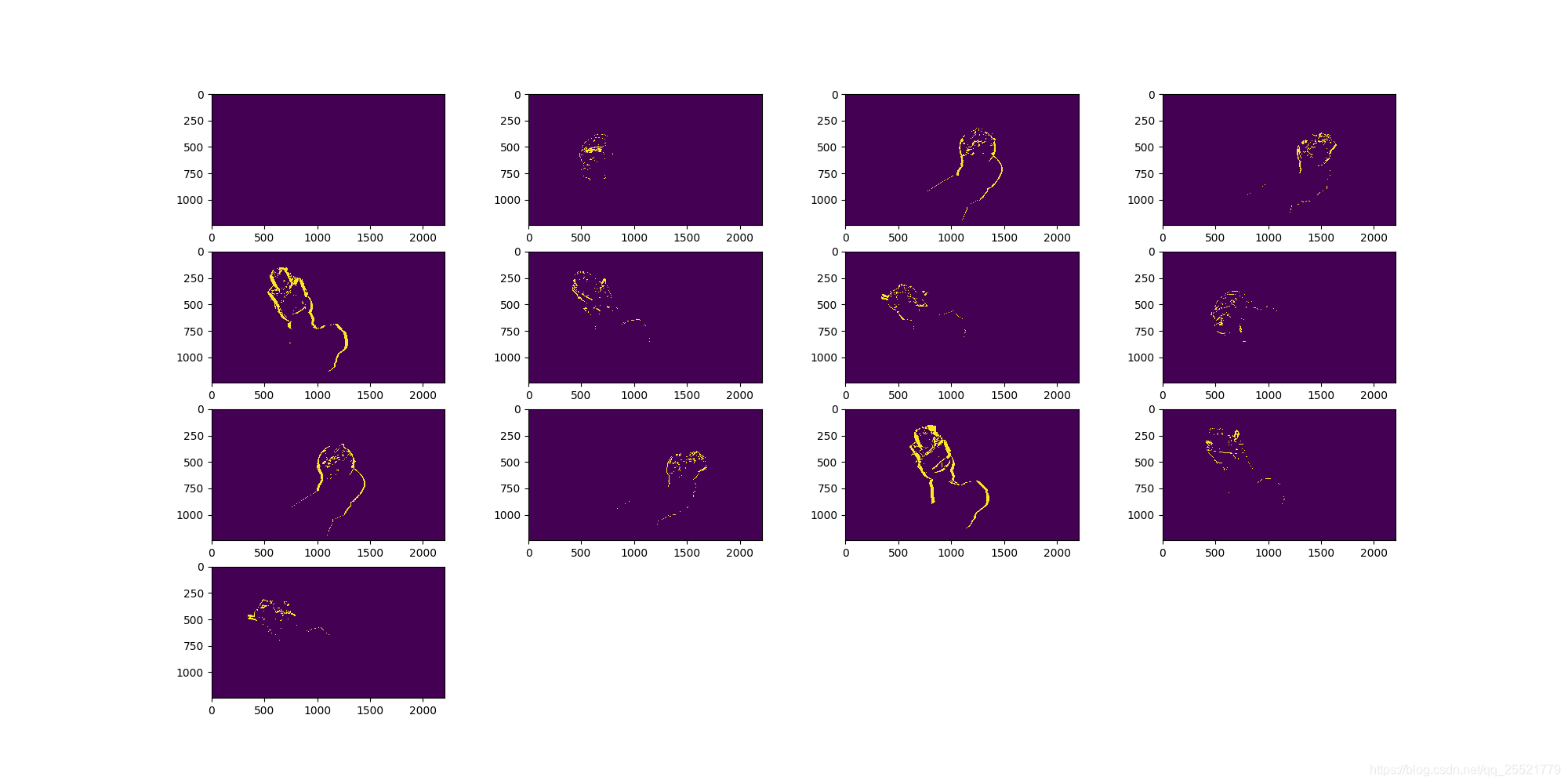
Algorithm 8: AdaptiveBackgroundLearning *****
The average processing time of this algorithm is 69.45 ms(Equivalent to 14.40 Hz), its background model is very admirable where the object of interest is almost vanished when it is moving. There are some afterglow in the background subtract results, because the object is not totally eliminated from background model. I think it can be solved by increasing threshold value. Anyway, the AdaptiveBackgroundLearning is a wonderful method and it can work all the time whether the object is moving or static.
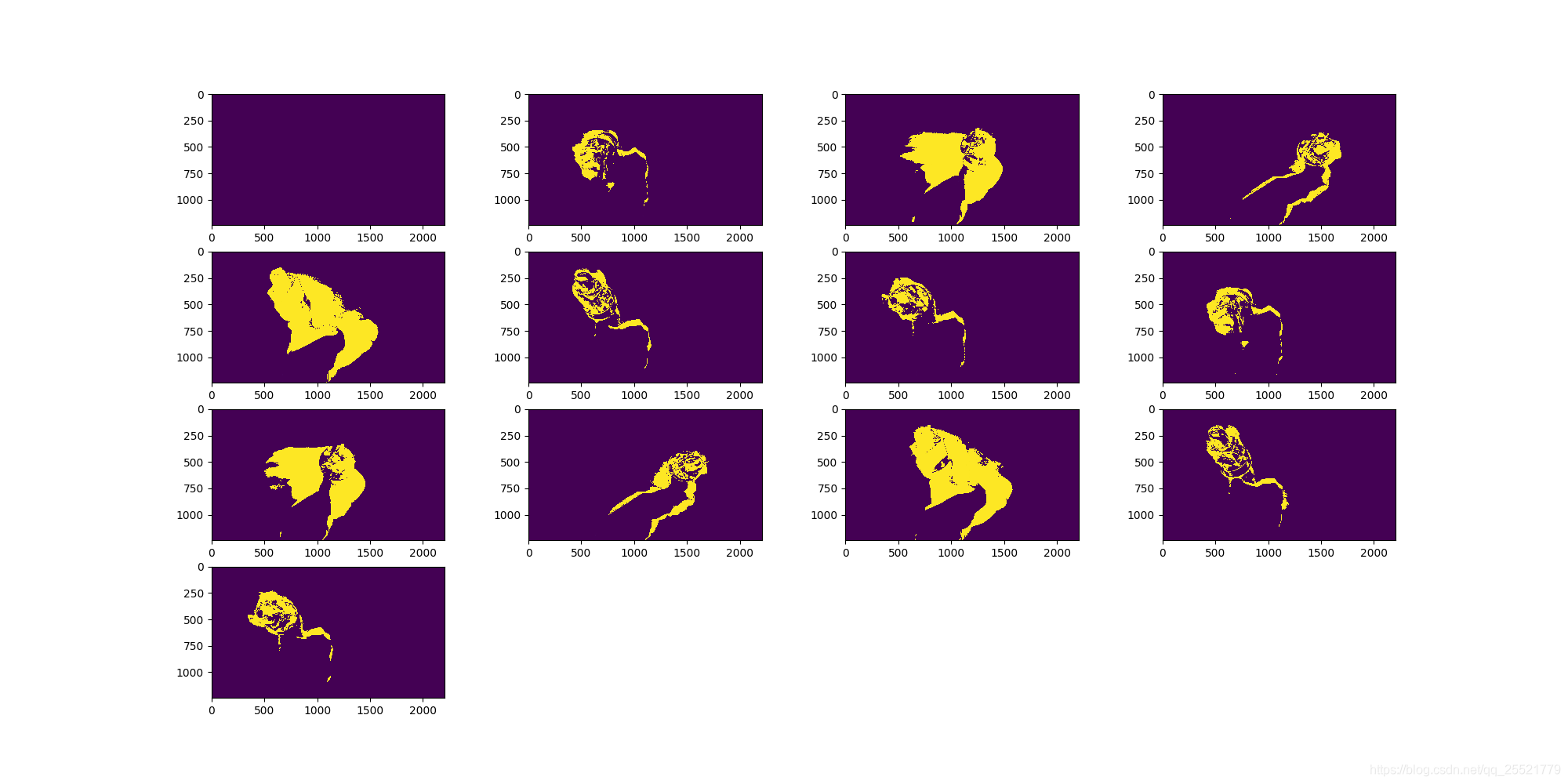
Algorithm 9: MixtureOfGaussianV2 *****
The average processing time of this algorithm is about 69.13 ms(Equivalent to 14.46 Hz), and its background model is also amazing. The background subtract results is admirable, but object of interest would disappear in results when it is static, which may caused by a high threshold value.
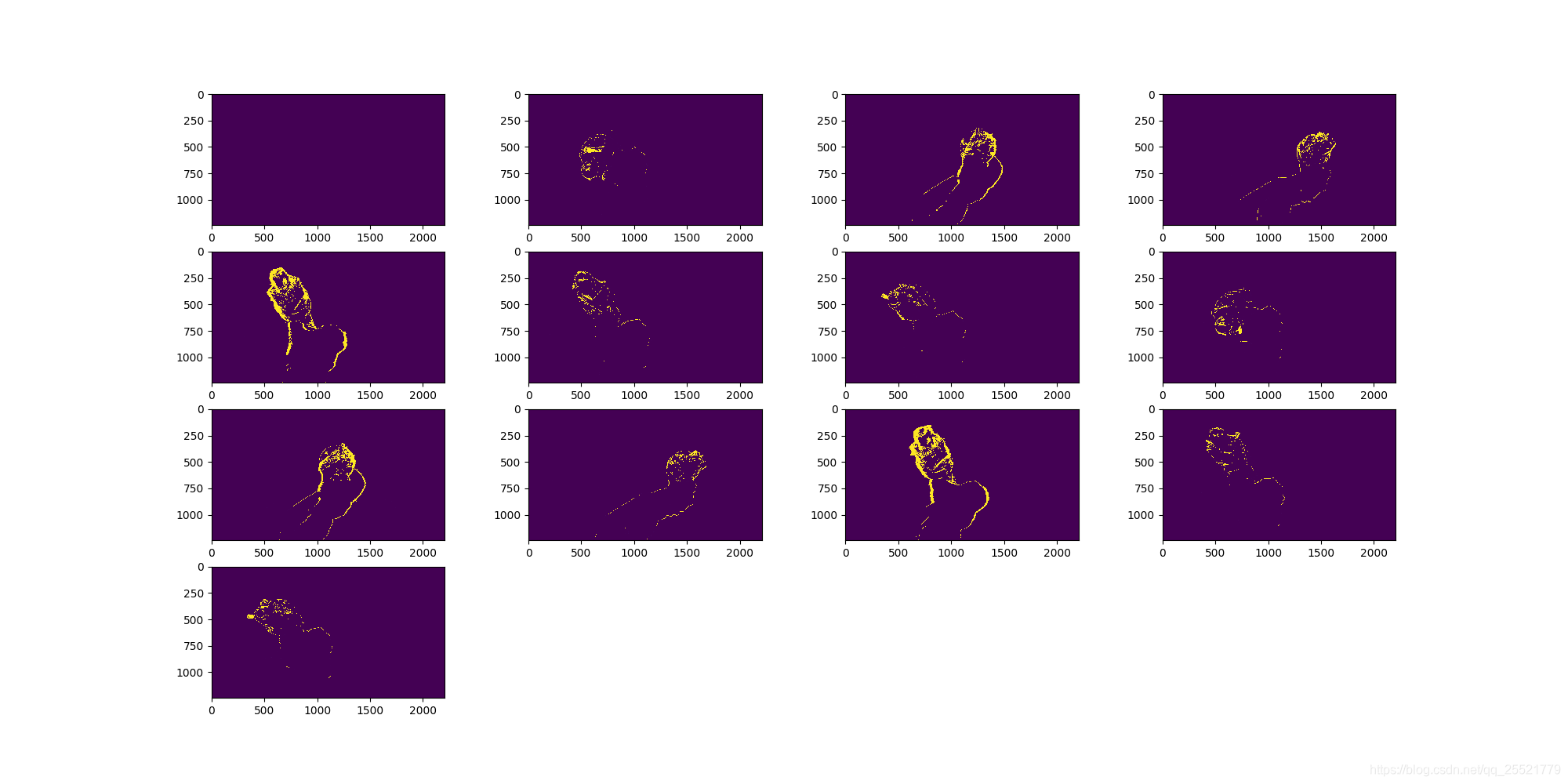
Algorithm 10:ViBe **
The average processing time of this method is about 66.88 ms(Equivalent to 14.95 Hz), The result of this algorithm and the background model are unsatisfied.
Algorithm 11:TwoPoints *****
The average processing time of this method is about 51.25 ms(Equivalent to 19.51 Hz). The background model will be updated and tend to the real background gradually, but the process of background estimation is slow. I think the convergence speed of background esitimation can be accelerated.
Algorithm 12: AdaptiveSelectiveBackgroundLearning **
The average processing time of this method is about 45.38 ms(Equivalent to 22.03 Hz), its background subtract results is not good, beacuse the object of interest in background model was not eliminated by update.
Algorithm 13: FrameDifference ****
The average processing time of this algorithm is 36.55 ms(Equivalent to 27.36 Hz), The background subtracted result contains all the moving objects, so the object of interest will vanish if it changes slowly. In a word, It can subtract background clearly and easily, but in some case it may stop working.
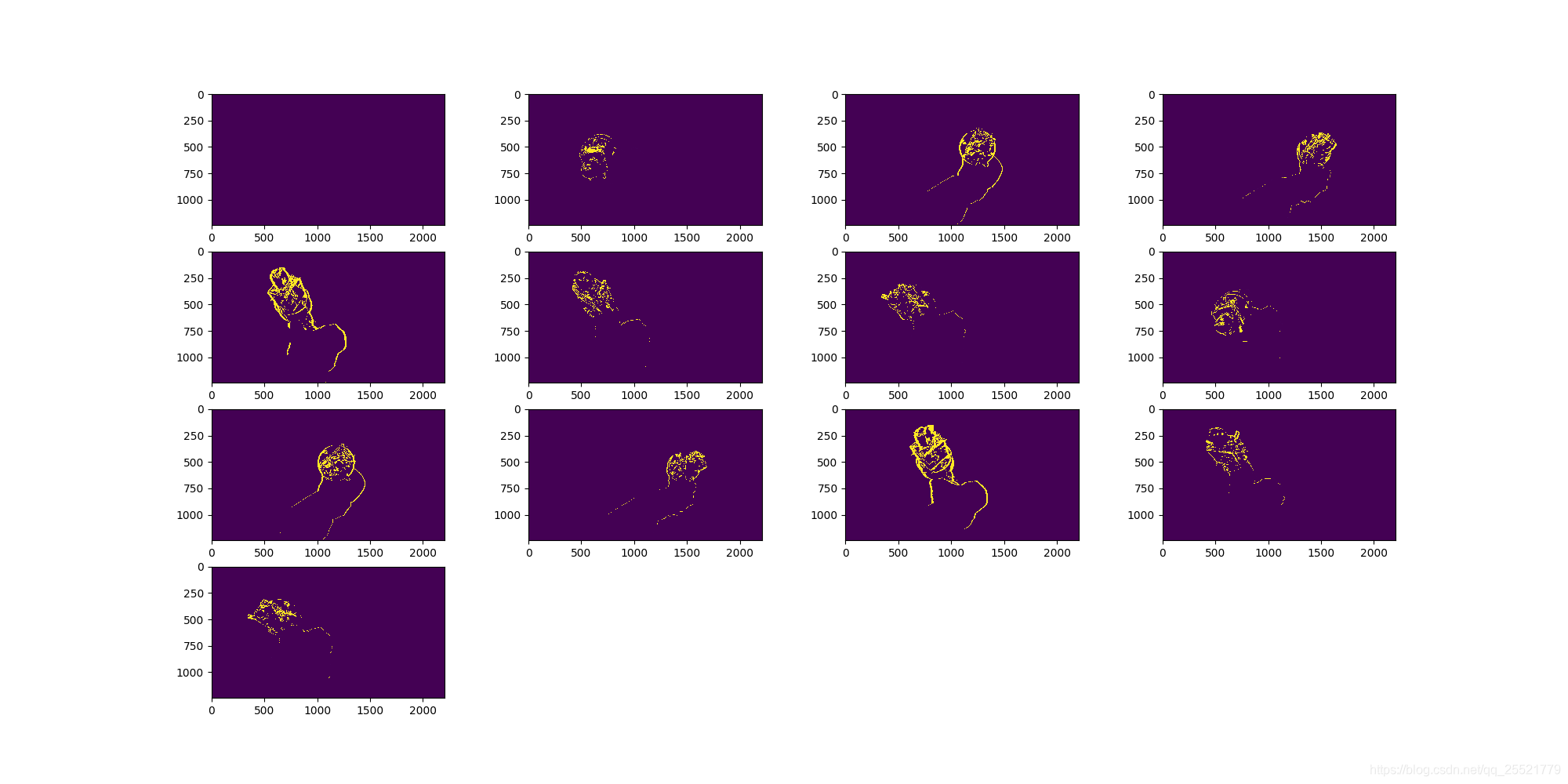
Algorithm 14: StaticFrameDifference **
The average processing time of this method is 42.45 ms(Equivalent to 23.56 Hz). In principle, It is similar to the Algorithm 13 – FrameDifference, the difference between two methods is the update fashion of background model. The ‘static’ in StaticFrameDifference means that the background model would not update after initialization. Therefore, it’s faster than FrameDifference.
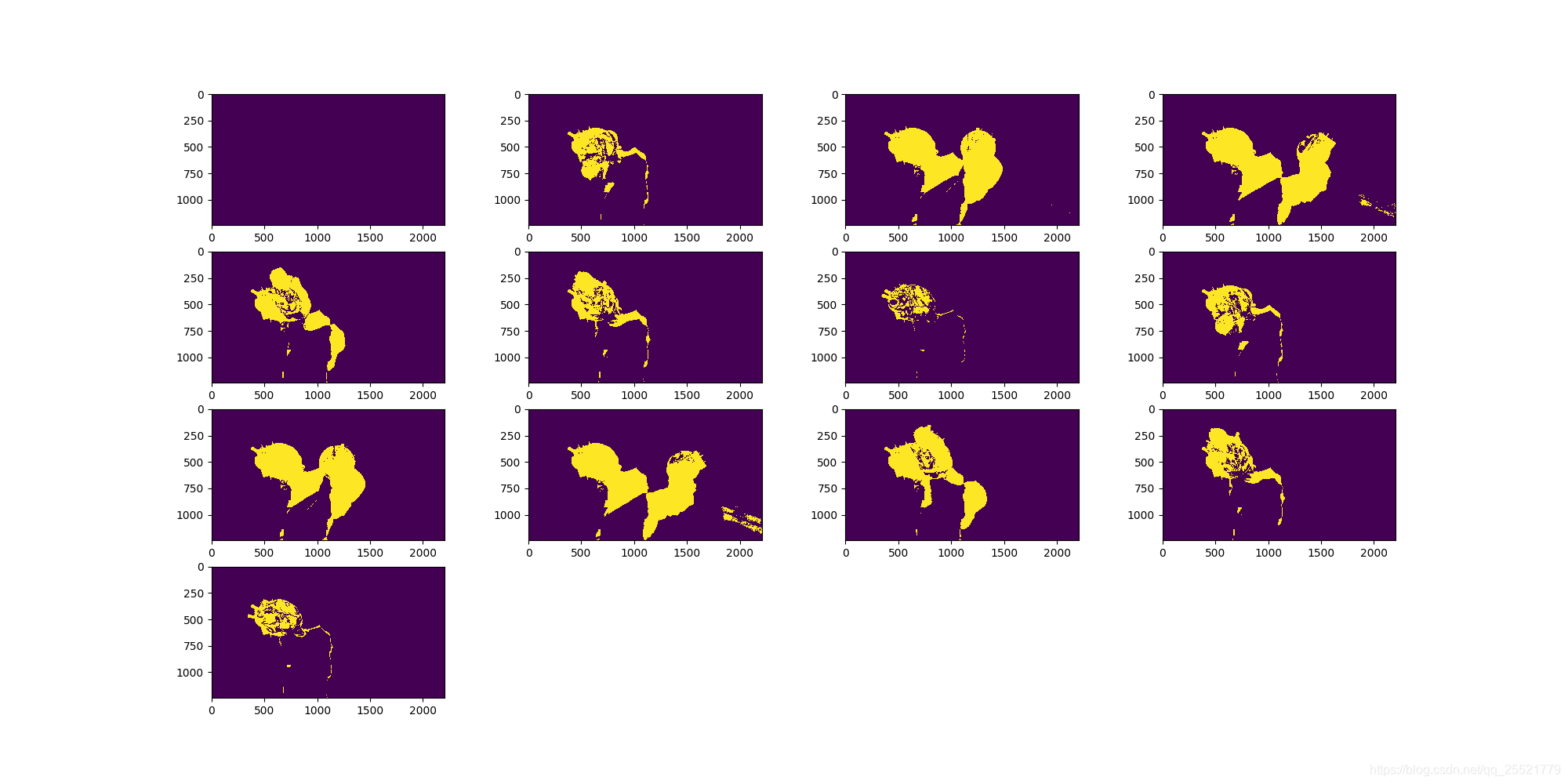