文章列表
1.自己编写深度学习框架ANNbox【高仿tensorflow】__01实现全连接.
2.自己编写深度学习框架ANNbox【高仿tensorflow】__02实现不同的优化方法.
3.自己编写深度学习框架ANNbox【高仿tensorflow】__03文本情感分析.
4.自己编写深度学习框架ANNbox【高仿tensorflow】__04卷积神经网络编写:实现AlexNet.
5.自己编写深度学习框架ANNbox【高仿tensorflow】__05循环神经网络编写:实现RNN(01).
…
自己编写深度学习框架ANNbox _02实现不同的优化方法
本节主要描述不同优化方法在MiniFlow中的实现方法,具体的公式及数学原理可详参【深度学习基础模型算法原理及编程实现–10.优化方法:从梯度下降到NAdam】。将不同优化方法类单独写在optimization.py文件中。
2.1 SGD
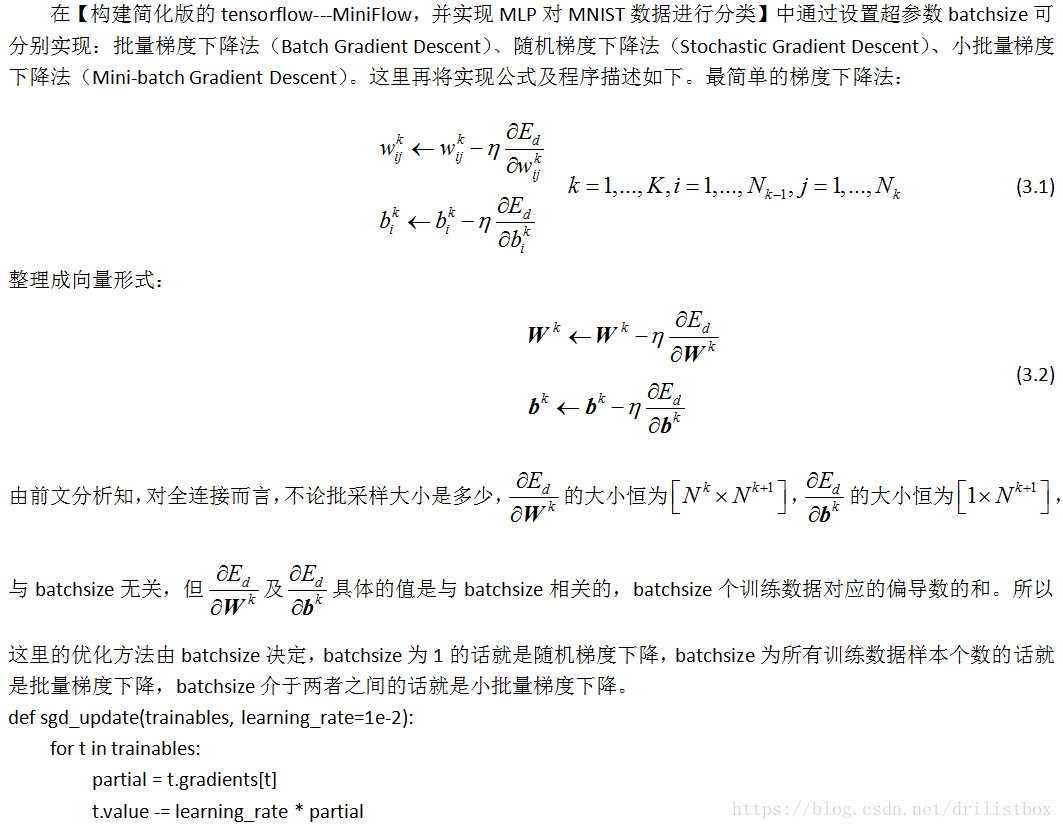
class GradientDescentOptimizer(Node):
def __init__(self,learning_rate=1e-2):
self.learning_rate=learning_rate
def minimize(self,loss):
'''暂时不知道怎么联系起来'''
self.loss=loss
return self
def run(self,feed_dict):
# print('optimization_forward_and_backward')
self.loss.check_graph(feed_dict)
graph = forward_and_backward(feed_dict,self.loss.L)
'''SGD_update'''
for t in trainables:
partial = t.gradients[t]
t.value -= self.learning_rate * partial
return graph
2.1.1 仿真算例
from tensorflow.examples.tutorials.mnist import input_data
import ANNbox as tf
import matplotlib.pyplot as plt
import numpy as np
import sys
import time
#loaddata
mnist = input_data.read_data_sets("./MNISTDat", one_hot=True)
#sess = tf.InteractiveSession()
# Create the model
in_units = 784
o_units = 10
W1 = tf.Variable(np.loadtxt('W1_784_10.txt'), name='image')
b1 = tf.Variable(tf.zeros([o_units]))
x = tf.placeholder(tf.float32, [None, in_units])
mal = tf.matmul(x, W1)
y = mal + b1
y_ = tf.placeholder(tf.float32, [None, o_units])
#cross_entropy = tf.reduce_sum(tf.nn.softmax_cross_entropy_with_logits(labels=y_, logits=y))
cross_entropy = tf.nn.softmax_cross_entropy_with_logits(labels=y_, logits=y, name='cost') #loss = tf.reduce_mean(cost)
#cross_entropy = tf.nn.cross_entropy_with_logits(labels=y_, logits=tf.nn.softmax(y), name='cost')
epochs = 1
m = 50000
batch_size = 64*2*2
learning_rate=1e-3
Momentum_rate=0.5
steps_per_epoch = m // batch_size
train_step = tf.train.GradientDescentOptimizer(learning_rate).minimize(cross_entropy)
#train_step = tf.train.MomentumOptimizer(learning_rate,Momentum_rate).minimize(cross_entropy)
#train_step = tf.train.AdagradOptimizer(0.3).minimize(cross_entropy)
start_time=time.time()
#sess=tf.Session()
tf.global_variables_initializer().run()
#sess.run(tf.global_variables_initializer())
loss_list,acc_train_list,acc_test_list=[],[],[]
for _ in range(epochs):
for i in range(steps_per_epoch):
batch_xs=mnist.train.images[i*batch_size:(i+1)*batch_size]
batch_ys=mnist.train.labels[i*batch_size:(i+1)*batch_size]
'''train'''
train_step.run({x: batch_xs, y_: batch_ys})
loss_list.append(np.mean(cross_entropy.my_eval({x: batch_xs, y_: batch_ys})))
acc_train_list.append(np.mean((np.argmax(y.my_eval({x: batch_xs, y_: batch_ys}),1) == np.argmax(batch_ys,1)).astype(int)))
acc_test_list.append(np.mean((np.argmax(y.my_eval({x: mnist.test.images, y_: mnist.test.labels}),1) == np.argmax(mnist.test.labels,1)).astype(int)))
sys.stdout.write("\rprocess: {}/{}, loss:{:.5f}, acc_train:{:.2f}, acc_test:{:.2f}".format(i, steps_per_epoch, loss_list[-1], acc_train_list[-1], acc_test_list[-1]))
plt.figure()
# plt.subplot(211)
plt.plot(range(len(loss_list)),loss_list,label=u'loss')
# plt.subplot(212)
plt.plot(range(len(loss_list)),acc_train_list,label=u'acc_train')
plt.plot(range(len(loss_list)),acc_test_list,label=u'acc_test')
plt.ylim([0,1])
plt.title('ANNbox')
plt.legend()
plt.show()
end_time = time.time()
print('total_time:',end_time-start_time)
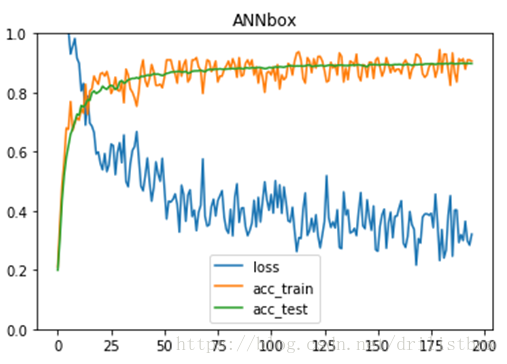
2.2 SGD with Momentum(SGDM)
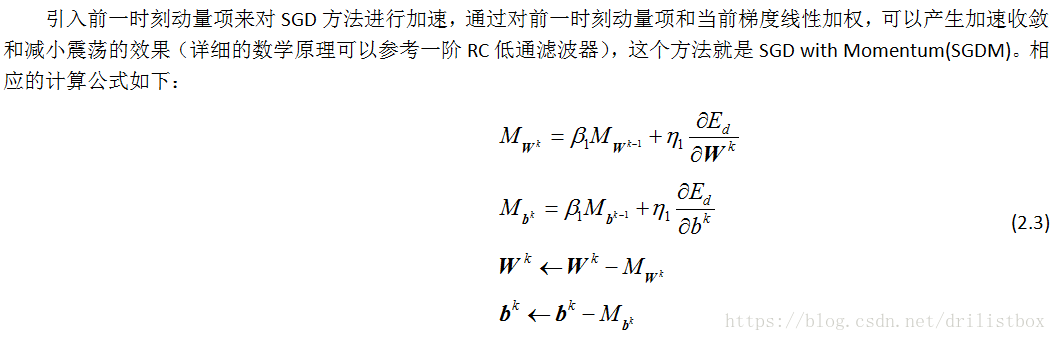
相应的代码实现如下:
class MomentumOptimizer(Node):
def __init__(self,learning_rate=1e-2, momentum_rate=0.9):
self.learning_rate=learning_rate
self.momentum_rate=momentum_rate
self.isMomentumInitial=False
def minimize(self,loss):
'''暂时不知道怎么联系起来'''
isLEnd[0]=True
self.loss=loss
# print('47_isLEnd:',isLEnd[0])
return self
def run(self,feed_dict):
# graph = forward_and_backward(feed_dict,self.L)
self.loss.check_graph(feed_dict)
# print('MomentumOptimizer中self.loss.L中所含的节点')
# for l_ in self.loss.L:
# print(l_.name)
graph = forward_and_backward(feed_dict,self.loss.L)
if(self.isMomentumInitial==False):
self.momentum = {t: np.zeros_like(t.gradients[t]) for t in trainables}
self.isMomentumInitial=True
'''SGD_update'''
for t in trainables:
self.momentum[t] *= self.momentum_rate
self.momentum[t] += self.learning_rate*t.gradients[t]
t.value -= self.momentum[t]
# t.value -= self.learning_rate * t.gradients[t]
return graph
2.2.1 仿真算例
from tensorflow.examples.tutorials.mnist import input_data
import ANNbox as tf
import matplotlib.pyplot as plt
import numpy as np
import sys
import time
#loaddata
mnist = input_data.read_data_sets("./../MNISTDat", one_hot=True)
#sess = tf.InteractiveSession()
# Create the model
in_units = 784
o_units = 10
W1=tf.Variable(tf.truncated_normal([in_units, o_units], stddev=0.1))
b1 = tf.Variable(tf.zeros([o_units]))
x = tf.placeholder(tf.float32, [None, in_units])
mal = tf.matmul(x, W1)
y = mal + b1
y_ = tf.placeholder(tf.float32, [None, o_units])
#cross_entropy = tf.reduce_sum(tf.nn.softmax_cross_entropy_with_logits(labels=y_, logits=y))
cross_entropy = tf.nn.softmax_cross_entropy_with_logits(labels=y_, logits=y, name='cost') #loss = tf.reduce_mean(cost)
#cross_entropy = tf.nn.cross_entropy_with_logits(labels=y_, logits=tf.nn.softmax(y), name='cost')
epochs = 1
m = 50000
batch_size = 64*2*2
learning_rate=1e-3
Momentum_rate=0.9
steps_per_epoch = m // batch_size
#train_step = tf.train.GradientDescentOptimizer(learning_rate).minimize(cross_entropy)
train_step = tf.train.MomentumOptimizer(learning_rate,Momentum_rate).minimize(cross_entropy)
#train_step = tf.train.AdagradOptimizer(0.3).minimize(cross_entropy)
start_time=time.time()
#sess=tf.Session()
tf.global_variables_initializer().run()
#sess.run(tf.global_variables_initializer())
loss_list,acc_train_list,acc_test_list=[],[],[]
for _ in range(epochs):
for i in range(steps_per_epoch):
batch_xs=mnist.train.images[i*batch_size:(i+1)*batch_size]
batch_ys=mnist.train.labels[i*batch_size:(i+1)*batch_size]
'''train'''
train_step.run({x: batch_xs, y_: batch_ys})
loss_list.append(np.mean(cross_entropy.my_eval({x: batch_xs, y_: batch_ys})))
acc_train_list.append(np.mean((np.argmax(y.my_eval({x: batch_xs, y_: batch_ys}),1) == np.argmax(batch_ys,1)).astype(int)))
acc_test_list.append(np.mean((np.argmax(y.my_eval({x: mnist.test.images, y_: mnist.test.labels}),1) == np.argmax(mnist.test.labels,1)).astype(int)))
sys.stdout.write("\rprocess: {}/{}, loss:{:.5f}, acc_train:{:.2f}, acc_test:{:.2f}".format(i, steps_per_epoch, loss_list[-1], acc_train_list[-1], acc_test_list[-1]))
plt.figure()
# plt.subplot(211)
plt.plot(range(len(loss_list)),loss_list,label=u'loss')
# plt.subplot(212)
plt.plot(range(len(loss_list)),acc_train_list,label=u'acc_train')
plt.plot(range(len(loss_list)),acc_test_list,label=u'acc_test')
plt.ylim([0,1])
plt.title('ANNbox')
plt.legend()
plt.show()
end_time = time.time()
print('total_time:',end_time-start_time)
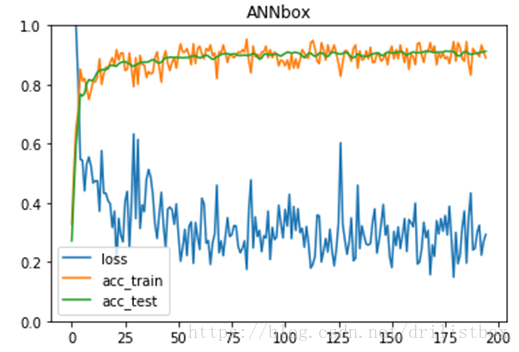
对比上一小结中的SGD方法,不难发现SGDM方法的收敛速度更快,但是收敛过程震荡小并不是很明显,这是因为每次梯度更新中用到的样本数batchsize较多,使得迭代过程原本就很稳定,从而SGDM并没有太明显的减震效果,如果减少样本数,那SGDM的减震效果还是很明显的。