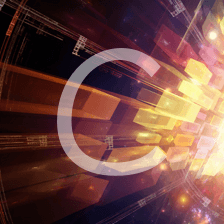
论文阅读
文章平均质量分 68
BLvren_
这个作者很懒,什么都没留下…
展开
-
Optimal Transport for knowledge distillation
OT 的应用场景往往是样本是在Euclidean上,而限制了其在structural data(graph, trees, time series)上的应用,或者跨space上的应用,这些统称为incomparable space情况。Wassertein distance作为比较概率分布的有用工具,得益于两点:1.它是距离;2. weak convergence of the probability measure.那么可以用样本的pm代替真实的pm....原创 2021-10-15 13:58:16 · 329 阅读 · 0 评论 -
20-CVPR-Distilling Knowledge from Graph Convolutional Networks
知识蒸馏知识蒸馏指的是模型压缩的思想,通过将预先训练的繁琐网络作为教师模型,知识蒸馏的目的是学习一个紧凑的学生模型,期望通过转移教师的知识来掌握教师的专业知识。KD在15-nipsworskshop-Distilling the knowledge in a neural network.被首先提出。20-CVPR-Distilling Knowledge from Graph Convolutional Networks摘要现有的知识蒸馏方法侧重于CNN,其中输入样本如图像位于网络域,并在很大程原创 2021-09-25 11:45:24 · 553 阅读 · 0 评论 -
18-NIPS-Differential Properties of Sinkhorn Approximation for Learning with Wasserstein Distance
摘要由于entropic regularization的计算优势,Optimal transport的应用得到了很多关注。但是,在很多情况下,Wasserstein Distance的sinkhorn approximation会被替换成一个regularized版本,不够准确但是容易微分。在这个工作中我们刻画原始的sinkhorn距离的微分特性,证明它和它的regularized版本同样smoothness,并且我们显式地提供了计算它的梯度的高效算法。这个结果对于理论和应用都很有用,一方面,高阶平滑为原创 2021-07-22 20:32:30 · 301 阅读 · 0 评论 -
An Efficient Approach to Informative Feature Extraction from Multimodal Data
文章目录摘要论文题目:An Efficient Approach to Informative Feature Extractionfrom Multimodal Data作者:Tsinghua-Berkeley Shenzhen Institute, Tencent AI Lab,Department of EECS, Massachusetts Institute of Technology时间:2018论文链接:https://arxiv.org/abs/1811.08979Github链原创 2020-09-13 23:43:24 · 728 阅读 · 3 评论 -
On The Power of Curriculum Learning in Training Deep Networks 论文阅读
文章目录摘要1 简介相关模型2 Curriculum learning2.2 Curriculum learning Method2.3 Scoring and Pacing Functions3 Empirical Evaluation论文题目:On The Power of Curriculum Learning in Training Deep Networks作者:Guy Hacohen Daphna Weinshall (The Hebrew University of Jerusalem)原创 2020-09-07 22:35:52 · 1079 阅读 · 1 评论 -
Item Tagging for Information Retrieval: A Tripartite Graph Neural Network based Approach 用于信息检索的项目标签
文章目录摘要简介Tagging 方法动机和总览动机总览TagGNN-IT节点表示TagGNN-IT Propagation损失2.3 TagGNN-QI2.3.1 边表示2.3.2 TagGNN-QI Propagation2.3.3 损失2.4 TagGNN2.5 Training and Inference2.5.1 Training2.5.2 Inference3 实验3.1 数据集3.2 Experimental Setup3.3 基准3.4 Performance Comparison (Q原创 2020-07-09 13:32:07 · 2403 阅读 · 0 评论