说在前面:本人使用的是PPLCNetV2_base 模型进行分类的,其他的没有试过,不过感觉应该一样
首先
需要安装cuda和cudnn 这个就比较坑了,本人装了以后预测不准,可能装坏了,不过使用cpu也可以啊
先卸载之前的
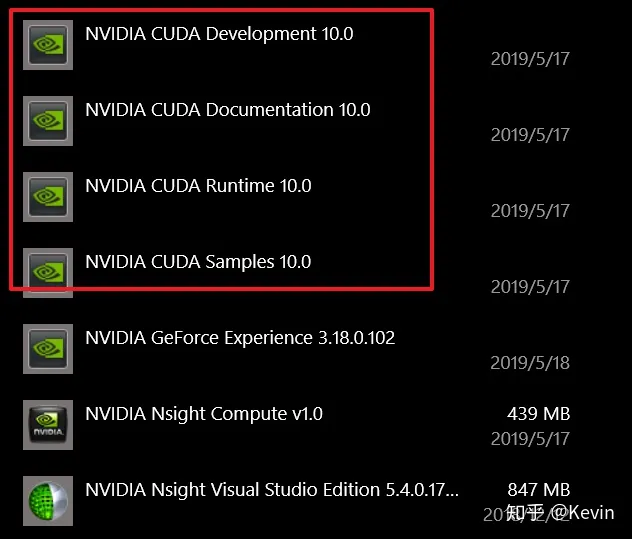
cuda 的安装
CUDA Toolkit Archive | NVIDIA Developer
该网站提供你想要的版本,本人使用的是cuda11.7.0
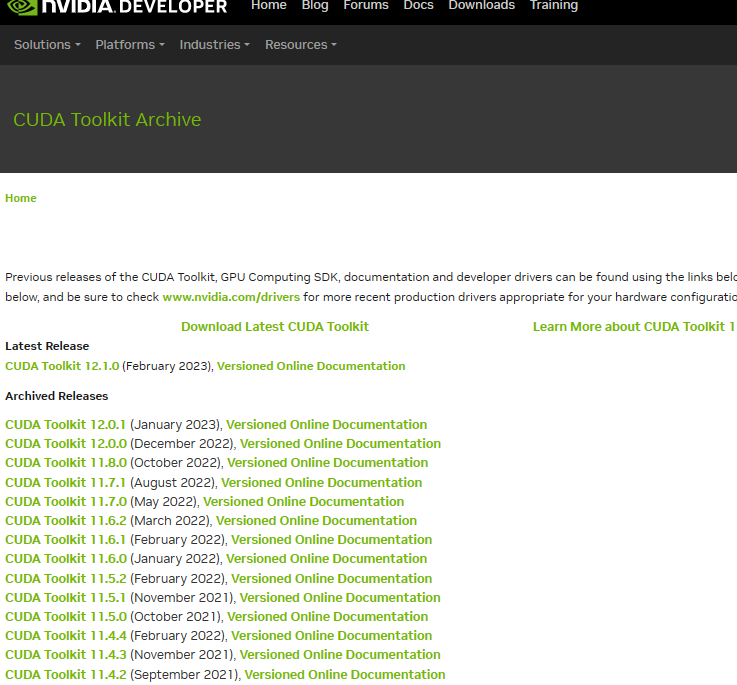
选择自定义安装,去掉第二个
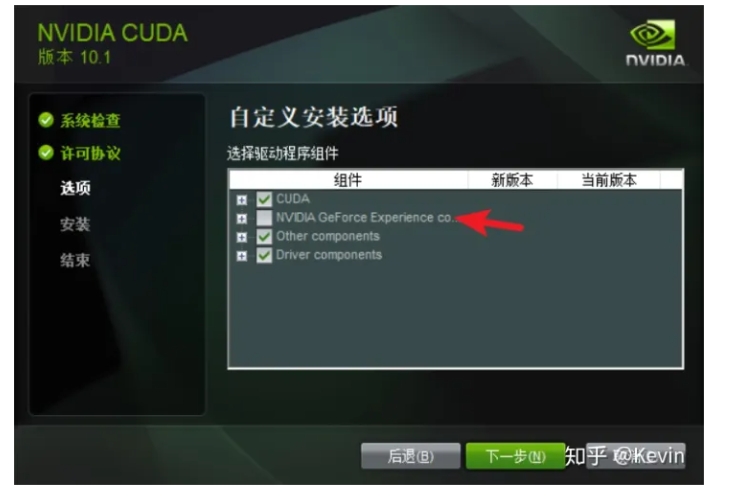
设置环境变量
Cudnn安装
cuDNN Archive | NVIDIA Developer
这个链接对应着许多版本,本人选择的是,许要注意cuda的版本号
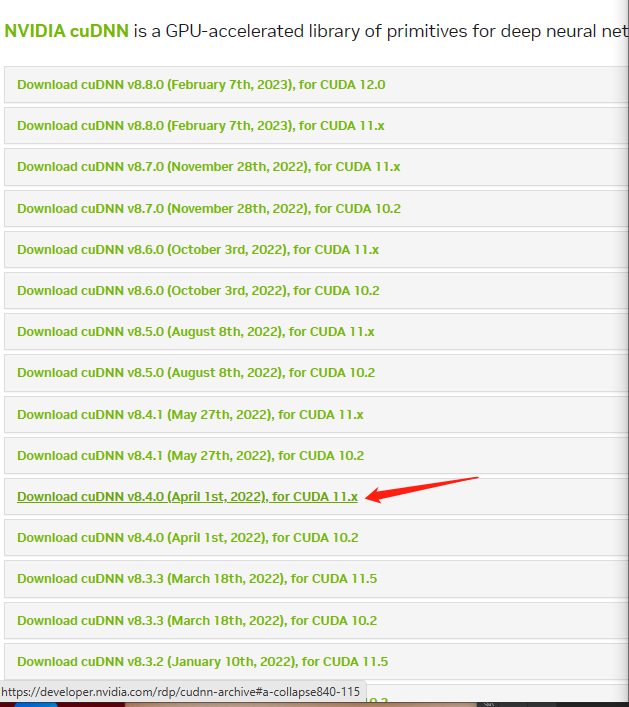
将下载到的压缩包解压到 cuda 的安装路径C:\Program Files\NVIDIA GPU Computing Toolkit\CUDA\v11.7下
安装paddle-gpu
pip install paddlepaddle-gpu==2.4.2
下载PaddleClas 源码
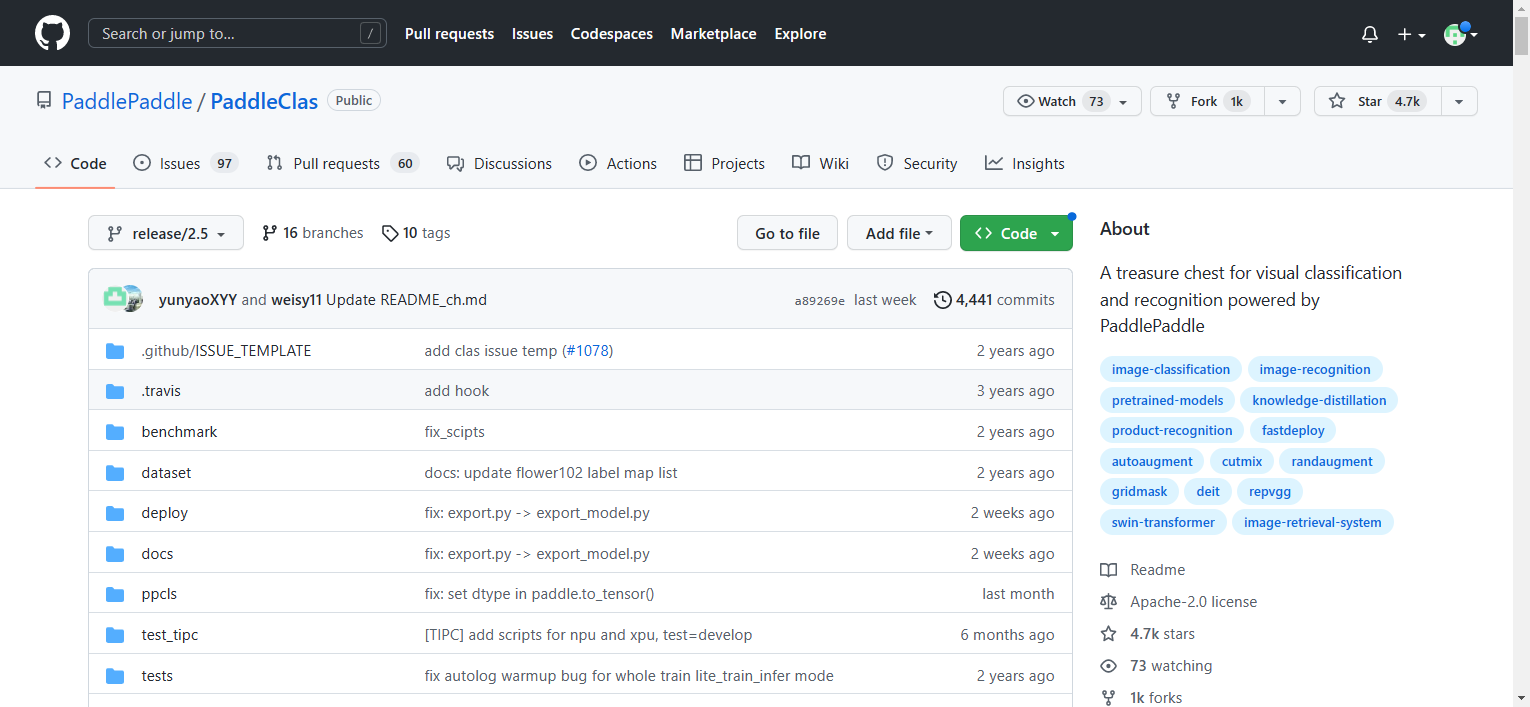
下载模型安装依赖:pip install --upgrade -r requirements.txt

运行
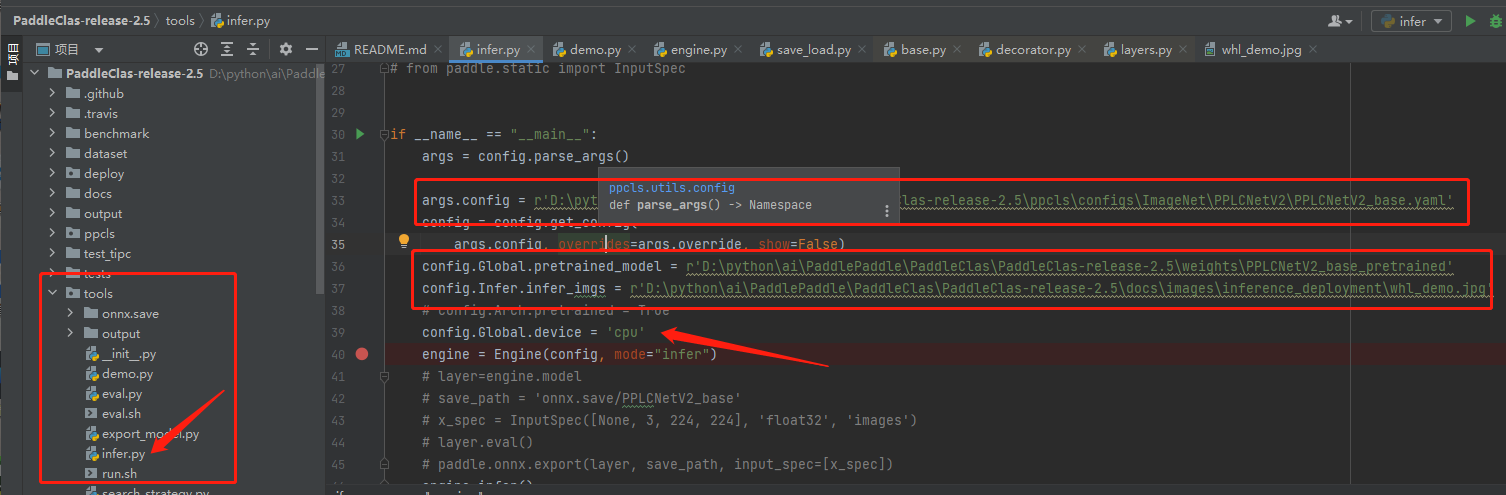
结果
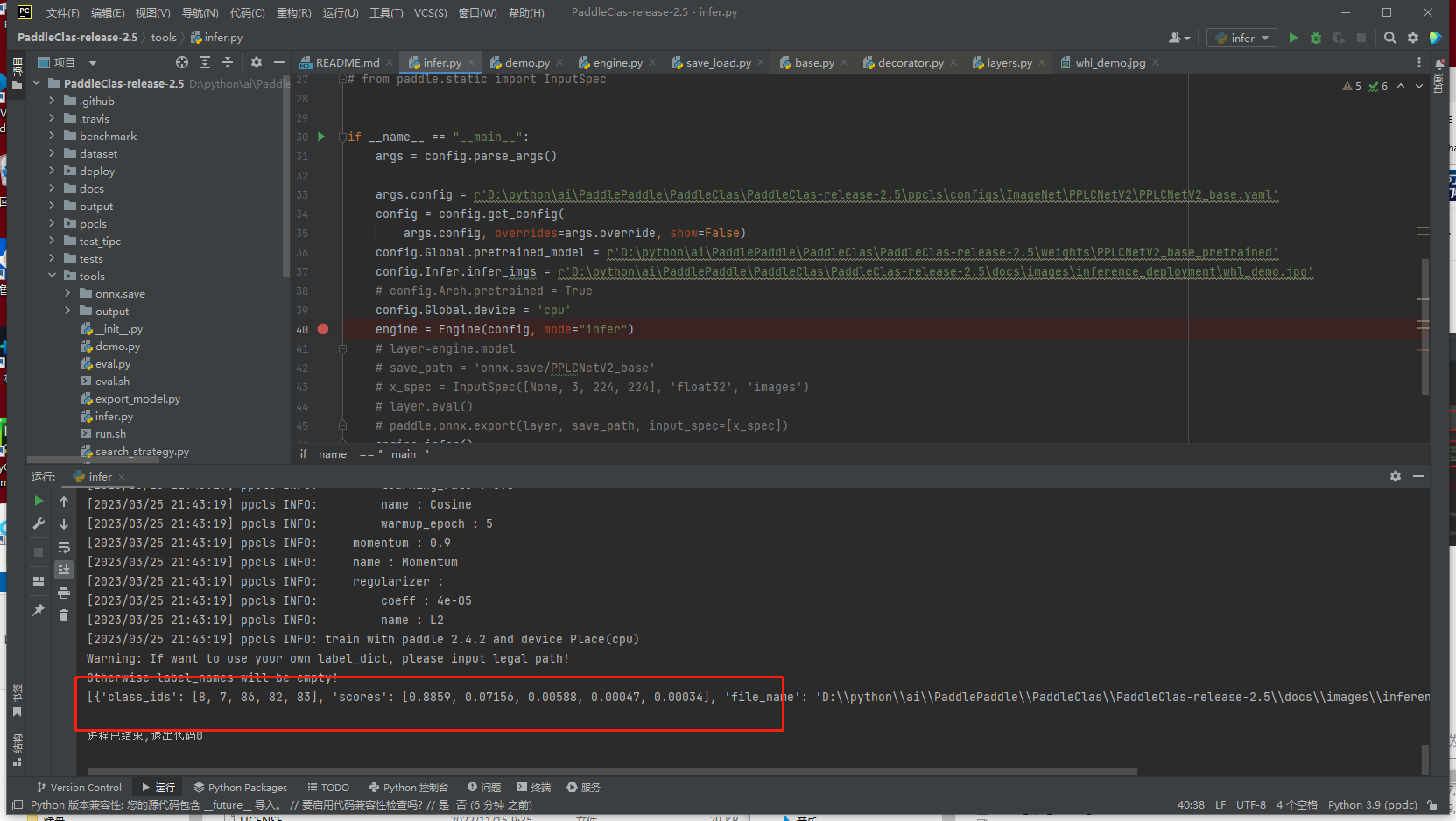
将模型导出onnx
需要下载 Paddle2ONNX 模块,可以的话可以把源码下载下来有案例
# Copyright (c) 2021 PaddlePaddle Authors. All Rights Reserved.
#
# Licensed under the Apache License, Version 2.0 (the "License");
# you may not use this file except in compliance with the License.
# You may obtain a copy of the License at
#
# http://www.apache.org/licenses/LICENSE-2.0
#
# Unless required by applicable law or agreed to in writing, software
# distributed under the License is distributed on an "AS IS" BASIS,
# WITHOUT WARRANTIES OR CONDITIONS OF ANY KIND, either express or implied.
# See the License for the specific language governing permissions and
# limitations under the License.
from __future__ import absolute_import
from __future__ import division
from __future__ import print_function
import os
import sys
__dir__ = os.path.dirname(os.path.abspath(__file__))
sys.path.append(os.path.abspath(os.path.join(__dir__, '../')))
from ppcls.utils import config
from ppcls.engine.engine import Engine
import paddle
from paddle.static import InputSpec
if __name__ == "__main__":
args = config.parse_args()
args.config = r'D:\python\ai\PaddlePaddle\PaddleClas\PaddleClas-release-2.5\ppcls\configs\ImageNet\PPLCNetV2\PPLCNetV2_base.yaml'
config = config.get_config(
args.config, overrides=args.override, show=False)
config.Global.pretrained_model = r'D:\python\ai\PaddlePaddle\PaddleClas\PaddleClas-release-2.5\weights\PPLCNetV2_base_pretrained'
config.Infer.infer_imgs = r'D:\python\ai\PaddlePaddle\PaddleClas\PaddleClas-release-2.5\docs\images\inference_deployment\whl_demo.jpg'
# config.Arch.pretrained = True
config.Global.device = 'cpu'
engine = Engine(config, mode="infer")
layer=engine.model
save_path = 'onnx.save/PPLCNetV2_base'
x_spec = InputSpec([None, 3, 224, 224], 'float32', 'images')
layer.eval()
paddle.onnx.export(layer, save_path, input_spec=[x_spec])
engine.infer()
结果
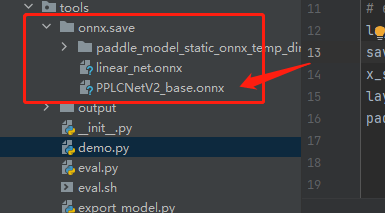
使用onnxruntime进行推理
import onnxruntime
import cv2
import torch
import numpy as np
import onnxruntime
import torch.nn.functional as F
x = np.random.random((2, 784)).astype('float32')
onnx_file = r'D:\python\ai\PaddlePaddle\PaddleClas\PaddleClas-release-2.5\tools\onnx.save\PPLCNetV2_base.onnx'
images = cv2.imread(r'D:\python\ai\PaddlePaddle\PaddleClas\PaddleClas-release-2.5\docs\images\inference_deployment\whl_demo.jpg', 1)
images=cv2.resize(images, (224, 224))
images = images[:, :, ::-1].transpose(2, 0, 1)
# images = np.ascontiguousarray(images)
images = images.astype('float32')
# images = torch.from_numpy(images).to("cpu")
# images = images.float() # uint8 to fp16/32
images = images / 255.0 # 0 - 255 to 0.0 - 1.0
if len(images.shape) == 3:
images = images[None] # expand for batch dim
ort_sess = onnxruntime.InferenceSession(onnx_file, providers=['CPUExecutionProvider'])
ort_inputs = {ort_sess.get_inputs()[0].name: images}
ort_outs = ort_sess.run([ort_sess.get_outputs()[0].name], ort_inputs)
ort_outs=np.array(ort_outs, dtype=np.float32)
# ort_outs=ort_outs.float()
x=torch.from_numpy(ort_outs).to("cpu")
x = F.softmax(x,dim =-1)
# x = softmax(x)
x = x.numpy()
y = []
for idx, probs in enumerate(x):
index =probs[0].argsort(axis=0)[-5:][::-1].astype(
"int32") if not False else np.where(
probs >= 0.5)[0].astype("int32")
clas_id_list = []
score_list = []
label_name_list = []
for i in index:
clas_id_list.append(i.item())
score_list.append(probs[0][i].item())
result = {
"class_ids": clas_id_list,
"scores": np.around(
score_list, decimals=5).tolist(),
}
# if file_names is not None:
# result["file_name"] = file_names[idx]
if label_name_list is not None:
result["label_names"] = label_name_list
y.append(result)
print(y)
# print(ort_outs)
结果:

备注:因为我转换图片比较粗暴,所以概率可能有点不一样
为解决问题:我的gpu运行不了,如何有可以直接使用的cuda和cudnn 包请给我来一份