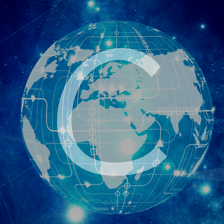
降维技术
淘淘图兔兔呀
这个作者很懒,什么都没留下…
展开
-
Generalized Autoencoder:A Neural Network Framework for Dimensionality Reduction——1. Introduction 引言
“Real-world data, such as images of faces and digits, usually have a high dimension which leads to the well-known curse of dimensionality in statistical pattern recognition.”现实世界中的数据,如人脸和数字图像,通常具有很高的维数,这就导致了统计模式识别中众所周知的维数诅咒。“Various methods of dimensiona原创 2020-12-03 11:48:48 · 309 阅读 · 0 评论 -
Generalized Autoencoder:A Neural Network Framework for Dimensionality Reduction——Abstract 摘要
“They just use each instance to reconstruct itself and ignore to explicitly model the data relation so as to discover the underlying effective manifold structure.”他们只是利用每个实例对自身进行重构,而忽略对数据关系的显式建模,从而发现底层有效的流形结构。...原创 2020-12-02 23:48:50 · 282 阅读 · 0 评论 -
Principal component analysis:a review and recent developments——2. The basic method基本方法
(a) Principal component analysis as an exploratory tool for data analysis“We seek a linear combination of the columns of matrix X with maximum variance.”我们寻求一个方差最大的矩阵X的列的线性组合。原创 2020-12-02 21:57:07 · 200 阅读 · 0 评论 -
Analysis of a complex of statistical variables into principal components——1. Introduction引言
“Consider n variables attaching to each individual of a population. These statistical variables x~1 x2, . . . , xn”原创 2020-12-02 16:17:13 · 661 阅读 · 0 评论 -
Principal component analysis:a review and recent developments——1. Introduction引言
“Its idea is simple—reduce the dimensionality of a dataset, while preserving as much ‘variability’ (i.e. statistical information) as possible.”它的想法很简单——降低数据集的维数,同时保留尽可能多的“可变性”(即统计信息)。“This means that ‘preserving as much variability as possible’ translate原创 2020-12-02 11:59:12 · 264 阅读 · 0 评论 -
Principal component analysis:a review and recent developments——摘要
“Large datasets are increasingly common and are often difficult to interpret. Principal component analysis (PCA) is a technique for reducing the dimensionality of such datasets, increasing interpretability but at the same time minimizing information loss.”原创 2020-12-02 11:12:09 · 354 阅读 · 0 评论