论文摘要:
We propose a novel multi-task learning architecture,which allows learning of task-specific feature-level attention. Our design, the Multi-Task Attention Network (MTAN), consists of a single shared network containing a global feature pool, together with a soft-attention module for each task. These modules allow for learning of task-specific features from the global features, whilst simultaneously allowing for features to be shared across different tasks. The architecture can be trained end-to-end and can be built upon any feed-forward neural network, is simple to implement, and is parameter efficient. We evaluate our approach on a variety of datasets, across both image-to-image predictions and image classification tasks. We show that our architecture is state-of-the-art in multi-task learning compared to existing methods, and is also less sensitive to various weighting schemes in the multi-task loss function. Code is available at https://github.com/lorenmt/mtan.
该论文提出了一种基于soft-attention模块的多任务学习框架,该框架包括一个主网络用来产生所有任务共享的feature,在此基础上,每个任务通过soft-attention模块从主网络从获取对自己有用的feature进行计算,最后达到多任务计算的效果。
- 框架构造
框架由一个主网络和K(任务数量)个子网络构成。主网络用来给所有任务共享特征,同时每个任务包括一个具有attention module模块的子网络。子网络通过attention module对主网络进行特征筛选。
同时,网络在整体架构上使用了encoder-decoder的模式,encoder对输入进行编码,decoder对encoder的输出进行解码操作,在encoder和decoder操作中都有attention module模块进行特征选择。encoder操作和decoder操作时相互对称的操作。
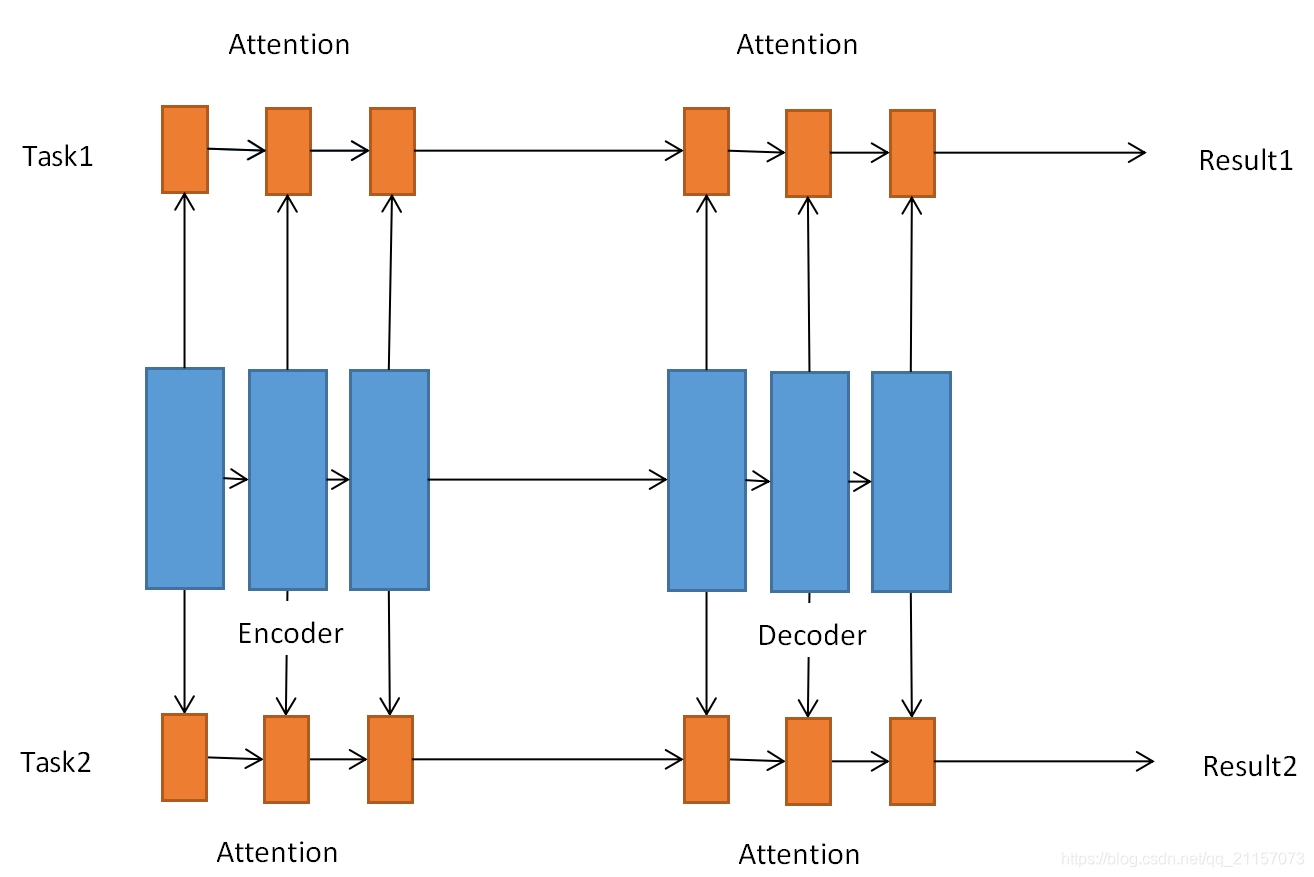
- 特定任务的注意力(Task Specific Attention Module)
通过在每个任务上的soft-attention-mask实现对主网络的特征筛选。 - 模型损失
模型分别对 semantic segmentation,depth estimation,surface normals三个任务共同进行了训练。所以任务的损失分为三个部分:
1.对于semantic segmentation,语义分割任务需要预测每个像素点的分类,所以使用逐像素的交叉熵损失:


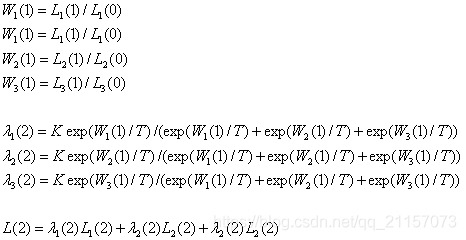
- 实验代码
代码主要使用pytorch构建,主要是net的 forward()函数这一块,跑一遍就可以知道网络的结构了:
首先,在forward中完成了encoder和decoder的传递,并将每一层的输出都保存起来,为后续操作做准备:
for i in range(5):
if i == 0:
g_encoder[i][0] = self.encoder_block[i](x)
g_encoder[i][1] = self.conv_block_enc[i](g_encoder[i][0])
g_maxpool[i], indices[i] = self.down_sampling(g_encoder[i][1])
else:
g_encoder[i][0] = self.encoder_block[i](g_maxpool[i - 1])
g_encoder[i][1] = self.conv_block_enc[i](g_encoder[i][0])
g_maxpool[i], indices[i] = self.down_sampling(g_encoder[i][1])
for i in range(5):
if i == 0:
g_upsampl[i] = self.up_sampling(g_maxpool[-1], indices[-i - 1])
g_decoder[i][0] = self.decoder_block[-i - 1](g_upsampl[i])
g_decoder[i][1] = self.conv_block_dec[-i - 1](g_decoder[i][0])
else:
g_upsampl[i] = self.up_sampling(g_decoder[i - 1][-1], indices[-i - 1])
g_decoder[i][0] = self.decoder_block[-i - 1](g_upsampl[i])
g_decoder[i][1] = self.conv_block_dec[-i - 1](g_decoder[i][0])
网络encoder和decoder都有五层,每层有三个块进行各种卷积和上下采样,如下图所示,图中encoder和decoder层置画出了三层:
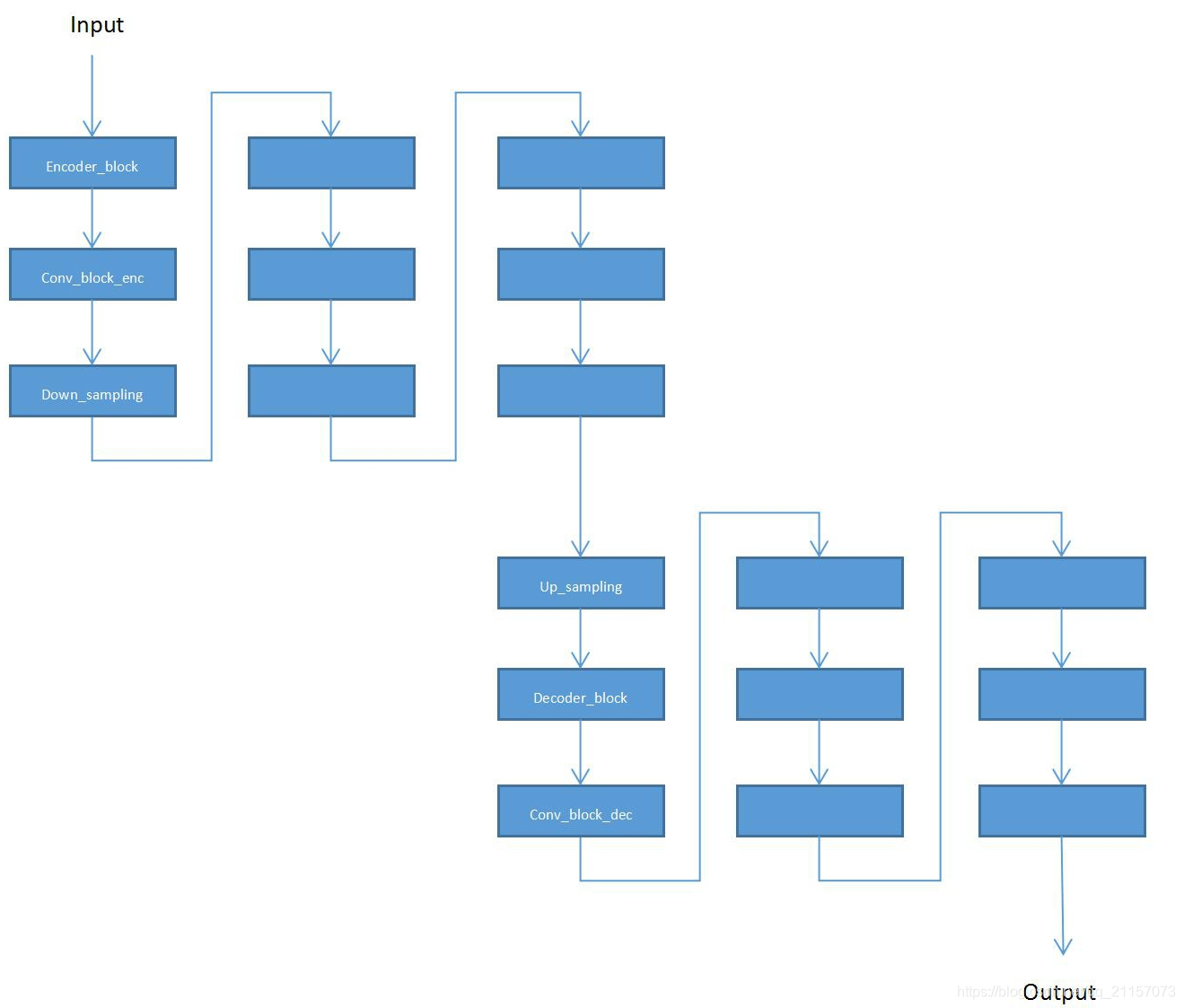
for i in range(3):
for j in range(5):
if j == 0:
atten_encoder[i][j][0] = self.encoder_att[i][j](g_encoder[j][0])
atten_encoder[i][j][1] = (atten_encoder[i][j][0]) * g_encoder[j][1]
atten_encoder[i][j][2] = self.encoder_block_att[j](atten_encoder[i][j][1])
atten_encoder[i][j][2] = F.max_pool2d(atten_encoder[i][j][2], kernel_size=2, stride=2)
else:
atten_encoder[i][j][0] = self.encoder_att[i][j](torch.cat((g_encoder[j][0], atten_encoder[i][j - 1][2]), dim=1))
atten_encoder[i][j][1] = (atten_encoder[i][j][0]) * g_encoder[j][1]
atten_encoder[i][j][2] = self.encoder_block_att[j](atten_encoder[i][j][1])
atten_encoder[i][j][2] = F.max_pool2d(atten_encoder[i][j][2], kernel_size=2, stride=2)
为三个任务中的每一层都添加一个attention,用来产生每个任务的mask,只画出一个任务的前两层attention如下图所示:
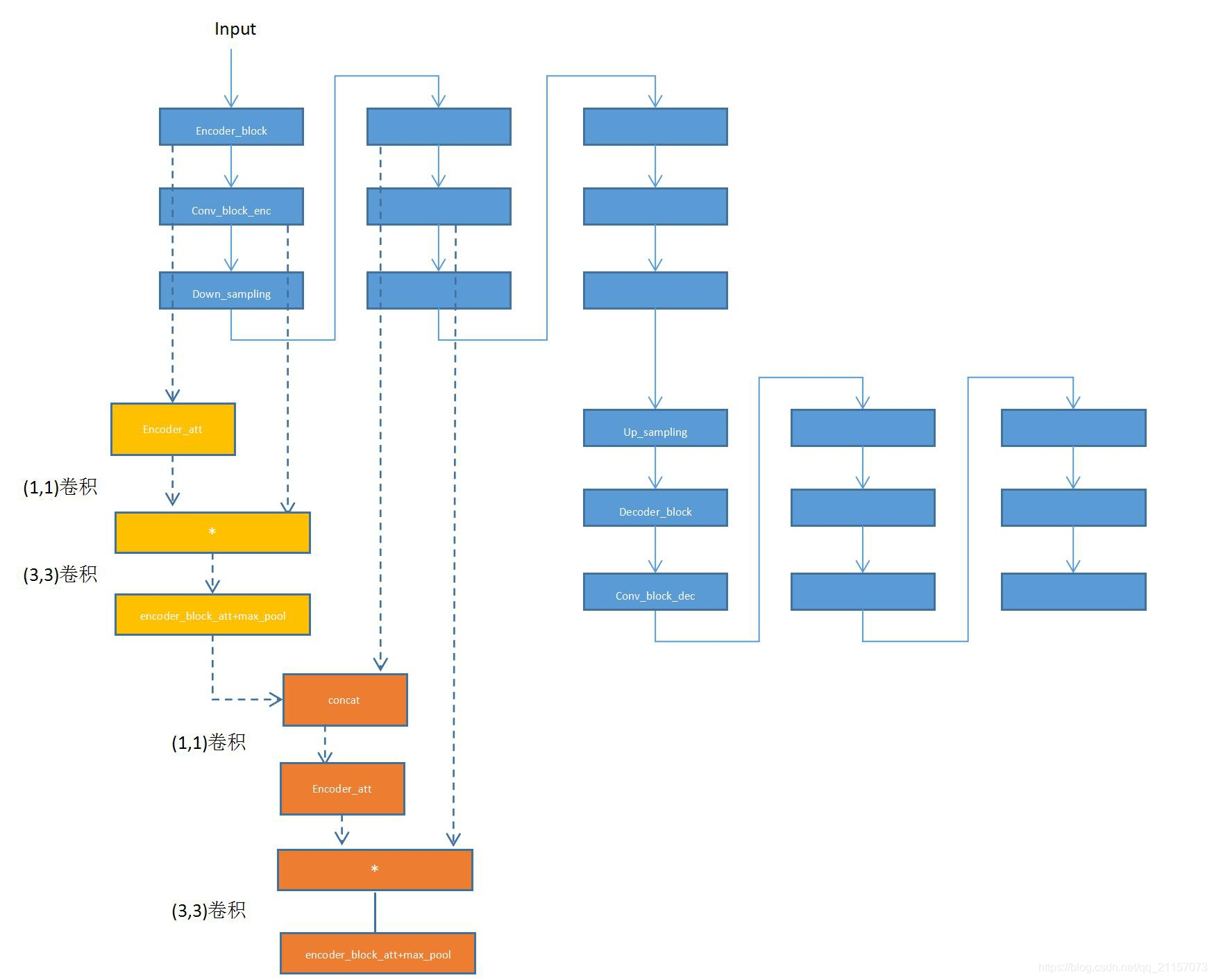
for j in range(5):
if j == 0:
# F.interpolate 上采样或者下采样数据
atten_decoder[i][j][0] = F.interpolate(atten_encoder[i][-1][-1], scale_factor=2, mode='bilinear', align_corners=True)
atten_decoder[i][j][0] = self.decoder_block_att[-j - 1](atten_decoder[i][j][0])
atten_decoder[i][j][1] = self.decoder_att[i][-j - 1](torch.cat((g_upsampl[j], atten_decoder[i][j][0]), dim=1))
atten_decoder[i][j][2] = (atten_decoder[i][j][1]) * g_decoder[j][-1]
else:
atten_decoder[i][j][0] = F.interpolate(atten_decoder[i][j - 1][2], scale_factor=2, mode='bilinear', align_corners=True)
atten_decoder[i][j][0] = self.decoder_block_att[-j - 1](atten_decoder[i][j][0])
atten_decoder[i][j][1] = self.decoder_att[i][-j - 1](torch.cat((g_upsampl[j], atten_decoder[i][j][0]), dim=1))
atten_decoder[i][j][2] = (atten_decoder[i][j][1]) * g_decoder[j][-1]
也是三个任务一共十五层添加attention,最后输出decoder的attention的结果:
这只是一个任务的attention,如果要三个的话,其中下半部分要重复三次。。。。(太难画了)
5. 实验结果
为了验证网络的设计,作者设计了很多实验进行验证。首先设计了单独的啥都没用的encoder-decoder用来解决单个任务;使用文章提出的网络架构来解决单个任务;传统的multi-task网络,在最后一层分离;传统的网络但是使用软参数共享;此外因为网络架构和cross-stitch网络很相似,还设计了cross-stitch网络进行验证;最后使用文章中提出的网络解决多任务,结果如下: