tf.nn.embedding_lookup的作用就是找到embedding data中对应行下的vector
tf.nn.embedding_lookup(params, ids, partition_strategy='mod', name=None, validate_indices=True, max_norm=None)#寻找params中索引为ids的vector
import pandas as pd
import numpy as np
from pandas import Series,DataFrame
import tensorflow as tf
p=tf.Variable(tf.random_normal([5,1]))#生成5*1的张量
b = tf.nn.embedding_lookup(p, [1, 3])#查找张量中的序号为1和3的
with tf.Session() as sess:
sess.run(tf.global_variables_initializer())
print(sess.run(b))
print('------')
print(sess.run(p))
[[0.5533718]
[0.6643605]]
------
[[ 0.16127495]
[ 0.5533718 ]
[-0.6191211 ]
[ 0.6643605 ]
[-1.9890579 ]]
tf.gather是从'params'中,按照axis坐标和indices标注的元素下标,把这些元素抽取出来组成新的tensor.
tf.gather(params, indices, validate_indices=None, name=None, axis=0)
import tensorflow as tf
temp = tf.range(0,10)*10 + tf.constant(1,shape=[10])
temp2 = tf.gather(temp,[2,5,8])
with tf.Session() as sess:
print(sess.run(temp))
print('------')
print(sess.run(temp2))
[ 1 11 21 31 41 51 61 71 81 91]
------
[21 51 81]
扫描下方二维码关注领取程序员必备千套ppt模板,300本精选好书,丰富面经:
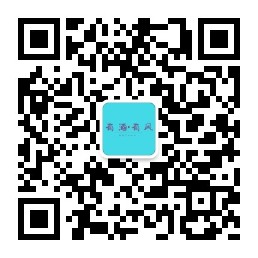