"ECO: Efficient Convolutional Network for Online Video Understanding, European Conference on Computer Vision (ECCV), 2018.”
paper: https://arxiv.org/pdf/1804.09066.pdf
code: https://github.com/mzolfaghari/ECO-efficient-video-understanding
PyTorch implementation : https://github.com/mzolfaghari/ECO-pytorch
论文主要提出了在保证比较好的识别率的同时提高识别速度的方法。文中说在一块Tesla P100 GPU上ECO 达到675fps,
ECO Lite 达到970fps。
那具体是怎么做的呢?
1. 对视频图像进行合理的采样。
作者认为视频图像的上下帧信息有很多冗余,于是把输入视频分为N个相同长度的sections,对每个块随机采取一帧图像进行输入进行处理。
2. 采用合适的网络结构。
先用2D网络对输入图像进行处理,再把所有块的2D网络输出送到一个3D网络进行分类。网络结构图如下:
2D-Net: 用的是the BN-Inception architecture (until inception-3c layer)
相关论文:Ioffffe, S., Szegedy, C.: Batch normalization: Accelerating deep network training by reducing internal covariate shift.
相关论文:.Tran, D., Ray, J., Shou, Z., Chang, S., Paluri, M.: Convnet architecture search for spatiotemporal feature learning.
2D-Nets: 用的是the BN-Inception architecture from inception-4a layer until last pooling layer
3. 训练细节
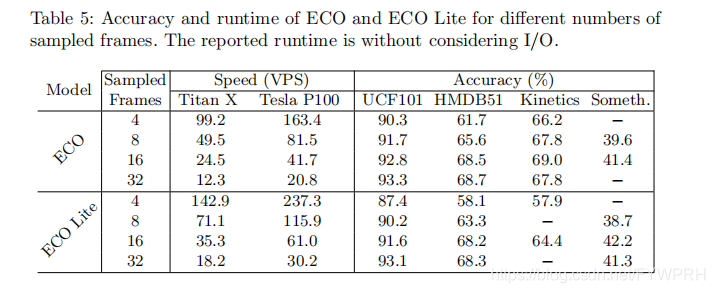
论文复现课程链接:https://aistudio.baidu.com/aistudio/education/group/info/1340