Spark 的五大核心要素包括:
- partition
- partitioner
- compute func
- dependency
- preferredLocation
RDD每次通过Transformation(map、flatMap、reduceByKey等等)进行转换后都会得到一个新的RDD,本篇文章以ShuffledRDD和JdbcRDD、HadoopRDD为例子,下面来介绍一下:
1、partition
(1)、partition的定义:
我们常说,partition 是 RDD 的数据单位,代表了一个分区的数据。但这里千万不要搞错了,partition 是逻辑概念,是代表了一个分片的数据,而不是包含或持有一个分片的数据。让我们来看看 Partition 的定义帮助理解:
trait Partition extends Serializable {
// Get the partition's index within its parent RDD
def index: Int
// A better default implementation of HashCode
override def hashCode(): Int = index
override def equals(other: Any): Boolean = super.equals(other)
}
查看spark源码,trait Partition的定义很简单,序列号index和hashCode方法。Partition和RDD是伴生的,即每一种RDD都有其对应的Partition实现,所以,分析Partition主要是分析其子类。ShuffledRDDPartition、JdbcPartition和HadoopPartition。
ShuffledRDD:
private[spark] class ShuffledRDDPartition(val idx: Int) extends Partition {
override val index: Int = idx
}
override def getPartitions: Array[Partition] = {
Array.tabulate[Partition](part.numPartitions)(i => new ShuffledRDDPartition(i))
}
JdbcRDD:
private[spark] class JdbcPartition(idx: Int, val lower: Long, val upper: Long) extends Partition
override def index: Int = idx
}
查看JdbcPartition实现,相比Partition,主要多了lower和upper这两个字段,spark sql在读取数据库时可根据这两个字段以及numpartitions这三个字段划分数据,例如lowerBound为1,upperBound为100,numPartitions为3则数据划分为(1,33)(34,66)(67,100)三个partition
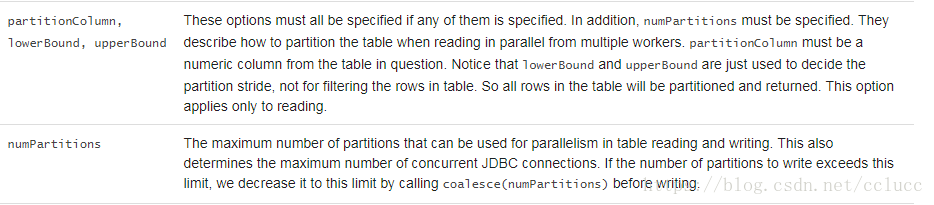
val jdbcDF = spark.read
.format("jdbc")
.option("url", "jdbc:postgresql:dbserver")
.option("dbtable", "schema.tablename")
.option("user", "username")
.option("password", "password")
.option("lowerBound", 1)
.option("upperBound", 100)
.option("numPartitions", 3)
.load()
override def getPartitions: Array[Partition] = {
// bounds are inclusive, hence the + 1 here and - 1 on end
val length = BigInt(1) + upperBound - lowerBound
(0 until numPartitions).map { i =>
val start = lowerBound + ((i * length) / numPartitions)
val end = lowerBound + (((i + 1) * length) / numPartitions) - 1
new JdbcPartition(i, start.toLong, end.toLong)
}.toArray
}
HadoopRDD:
private[spark] class HadoopPartition(rddId: Int, override val index: Int, s: InputSplit)
extends Partition {
val inputSplit = new SerializableWritable[InputSplit](s)
override def hashCode(): Int = 31 * (31 + rddId) + index
override def equals(other: Any): Boolean = super.equals(other)
/**
* Get any environment variables that should be added to the users environment when running pipes
* @return a Map with the environment variables and corresponding values, it could be empty
*/
def getPipeEnvVars(): Map[String, String] = {
val envVars: Map[String, String] = if (inputSplit.value.isInstanceOf[FileSplit]) {
val is: FileSplit = inputSplit.value.asInstanceOf[FileSplit]
// map_input_file is deprecated in favor of mapreduce_map_input_file but set both
// since it's not removed yet
Map("map_input_file" -> is.getPath().toString(),
"mapreduce_map_input_file" -> is.getPath().toString())
} else {
Map()
}
envVars
}
}
相比Partition,HadoopPartition则多了
InputSplit
。spark切分hdfs文件,调用的是Hadoop的API
override def getPartitions: Array[Partition] = {
val jobConf = getJobConf()
// add the credentials here as this can be called before SparkContext initialized
SparkHadoopUtil.get.addCredentials(jobConf)
val allInputSplits = getInputFormat(jobConf).getSplits(jobConf, minPartitions)
val inputSplits = if (ignoreEmptySplits) {
allInputSplits.filter(_.getLength > 0)
} else {
allInputSplits
}
val array = new Array[Partition](inputSplits.size)
for (i <- 0 until inputSplits.size) {
array(i) = new HadoopPartition(id, i, inputSplits(i))
}
array
}
(2)、partition在RDD中的使用
RDD 由若干个 partition 组成,共有三种生成方式:
从 Scala 集合中创建,通过调用 SparkContext#makeRDD 或 SparkContext#parallelize
加载外部数据来创建 RDD,例如从 HDFS 文件、mysql 数据库读取数据等
由其他 RDD 执行 transform 操作转换而来
那么,在使用上述方法生成 RDD 的时候,会为 RDD 生成多少个 partition 呢?一般来说,加载 Scala 集合或外部数据来创建 RDD 时,是可以指定 partition 个数的,若指定了具体值,那么 partition 的个数就等于该值,比如:
val rdd1 = sc.makeRDD( scalaSeqData, 3 ) //< 指定 partition 数为3val rdd2 = sc.textFile( hdfsFilePath, 10 ) //< 指定 partition 数为10
若没有指定具体的 partition 数时的 partition 数为多少呢?
对于从 Scala 集合中转换而来的 RDD:默认的 partition 数为 defaultParallelism,该值在不同的部署模式下不同:
Local 模式:本机 cpu cores 的数量
Mesos 模式:8
Yarn:max(2, 所有 executors 的 cpu cores 个数总和)
对于从外部数据加载而来的 RDD:默认的 partition 数为 min(defaultParallelism, 2)
对于执行转换操作而得到的 RDD:视具体操作而定,如 map 得到的 RDD 的 partition 数与 父 RDD 相同;union 得到的 RDD 的 partition 数为父 RDDs 的 partition 数之和...
(3)、partition数量影响以及调整
Partition数量太少 ,太少的影响显而易见,就是资源不能充分利用,例如local模式下,有16core,但是Partition数量仅为8的话,有一半的core没利用到。
Partition数量太多,资源利用没什么问题,但是导致task过多,task的序列化和传输的时间开销增大
调整:reparation是coalesce(numPartitions, shuffle = true),repartition不仅会调整Partition数,也会将Partitioner修改为hashPartitioner,产生shuffle操作。coalesce函数可以控制是否shuffle,但当shuffle为false时,只能减小Partition数,无法增大
repartition和coalesce是对RDD的分区进行重新划分,repartition只是coalesce接口中shuffle为true的简易实现,所以这里主要讨论coalesce合并函数该如何设置shuffle参数,这里分三种情况,假设RDD为N个分区,需要重新划分M个分区
1、如果N< M,一般情况下N个分区有数据分布不均的状况,利用HashPartitioner函数将数据重新分区为M个,这时需要将shuffle设置为true
2、如果N>M并且N和M相差不多,比如N是1000,M是100,那么就可以将N个分区中的若干个分区合并成一个新的分区,最终合并成M个分区,这时可以将shuffle的参数设置为false(在shuffle为false的情况下,设置M>N,coalesce是不起作用的),不进行shuffle过程,父RDD和子RDD之间是窄依赖关系
3、如果N>M并且N和M差距悬殊大,比如N是1000,M是1,这个时候如果把shuffle设置成false,由于父子RDD是窄依赖,它们同处在一个stage中,就可能会造成spark程序运行的并行度不够,从而影响性能,比如在M为1时,由于只有一个分区,所以只会有一个任务在运行,为了是coalesce之前的操作有更好的并行度,可以将shuffle参数设置为true。
2、partitioner
(1)、partitioner的定义
partitioner 即分区器,说白了就是决定 RDD 的每一条消息应该分到哪个分区。但只有 k, v 类型的 RDD 才能有 partitioner(当然,非 key, value 类型的 RDD 的 partitioner 为 None。
partitioner 为 None 的 RDD 的 partition 的数据要么对应数据源的某一段数据,要么来自对父 RDDs 的 partitions 的处理结果。
我们先来看看 Partitioner 的定义及注释说明:
abstract class Partitioner extends Serializable {
//< 返回 partition 数量
def numPartitions: Int
//< 返回 key 应该属于哪个
partition def getPartition(key: Any): Int
}
默认partitioner的选择策略,首先按照RDD的partition数从多到少排序,然后依次遍历RDD,如果其partitioner存在侧返回,如果所有RDD都不存在partitioner,则返回HashPartitioner。如果
spark.default.parallelism不为空则Partition数为spark.default.parallelism或者所有RDD中Partition数最大者
object Partitioner {
def defaultPartitioner(rdd: RDD[_], others: RDD[_]*): Partitioner = {
val rdds = (Seq(rdd) ++ others)
val hasPartitioner = rdds.filter(_.partitioner.exists(_.numPartitions > 0))
val hasMaxPartitioner: Option[RDD[_]] = if (hasPartitioner.nonEmpty) {
Some(hasPartitioner.maxBy(_.partitions.length))
} else {
None
}
val defaultNumPartitions = if (rdd.context.conf.contains("spark.default.parallelism")) {
rdd.context.defaultParallelism
} else {
rdds.map(_.partitions.length).max
}
// If the existing max partitioner is an eligible one, or its partitions number is larger
// than the default number of partitions, use the existing partitioner.
if (hasMaxPartitioner.nonEmpty && (isEligiblePartitioner(hasMaxPartitioner.get, rdds) ||
defaultNumPartitions < hasMaxPartitioner.get.getNumPartitions)) {
hasMaxPartitioner.get.partitioner.get
} else {
new HashPartitioner(defaultNumPartitions)
}
}
.......
}
Partitioner 共有两种实现,分别是 HashPartitioner 和 RangePartitioner
HashPartitioner :
class HashPartitioner(partitions: Int) extends Partitioner {
require(partitions >= 0, s"Number of partitions ($partitions) cannot be negative.")
//直接返回主构造函数中传入的 partitions 参数
def numPartitions: Int = partitions
//为参数 key 计算一个 hash 值,该哈希值对 partition 个数取余结果为正,则该结果即该 key 归属的 partition index;否则,以该结果加上 partition 个数为 partition index
def getPartition(key: Any): Int = key match {
case null => 0
case _ => Utils.nonNegativeMod(key.hashCode, numPartitions)
}
override def equals(other: Any): Boolean = other match {
case h: HashPartitioner =>
h.numPartitions == numPartitions
case _ =>
false
}
override def hashCode: Int = numPartitions
}
从上面的分析来看,当 key, value 类型的 RDD 的 key 的 hash 值分布不均匀时,会导致各个 partition 的数据量不均匀,极端情况下一个 partition 会持有整个 RDD 的数据而其他 partition 则不包含任何数据,这显然不是我们希望看到的,这时就需要 RangePartitioner 出马了。
(2)、partitioner
的使用
使用Partitioner必须满足两个前提,1、RDD是k-v形式,如RDD[(K, V)],2、有shuffle操作。在使用SparkContext构建RDD的时候,RDD的partitioner信息,一开始为None,只有在key-value类型的RDD中可以设置partitioner信息,在使用partitioner优化RDD的代码的时,最常用到的还是几个链接操作,cogroup、join、leftOuterJoin、rightOuterJoin、fullOuterJoin,这几个操作最终都是调用cogroup方法进行链接操作.
Partitioner是在shuffle阶段起作用
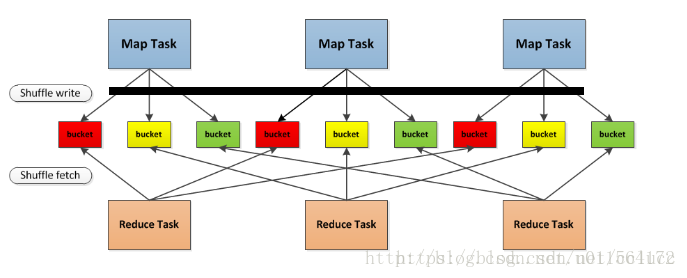
如上图,在shuffle中,map阶段处理结果使用ShuffleWriter,根据Partitioner逻辑写到不同bucket中,不同的bucket后续被不同的reducer使用,源码如下:
ShuffleMapTask.runTask:
try {
val manager = SparkEnv.get.shuffleManager
writer = manager.getWriter[Any, Any](dep.shuffleHandle, partitionId, context)
writer.write(rdd.iterator(partition, context).asInstanceOf[Iterator[_ <: Product2[Any, Any]]])
writer.stop(success = true).get
} catch {
.....
}
SortShuffleManager.getWriter:
override def getWriter[K, V](
handle: ShuffleHandle,
mapId: Int,
context: TaskContext): ShuffleWriter[K, V] = {
numMapsForShuffle.putIfAbsent(
handle.shuffleId, handle.asInstanceOf[BaseShuffleHandle[_, _, _]].numMaps)
val env = SparkEnv.get
handle match {
case unsafeShuffleHandle: SerializedShuffleHandle[K @unchecked, V @unchecked] =>
new UnsafeShuffleWriter(
env.blockManager,
shuffleBlockResolver.asInstanceOf[IndexShuffleBlockResolver],
context.taskMemoryManager(),
unsafeShuffleHandle,
mapId,
context,
env.conf)
case bypassMergeSortHandle: BypassMergeSortShuffleHandle[K @unchecked, V @unchecked] =>
new BypassMergeSortShuffleWriter(
env.blockManager,
shuffleBlockResolver.asInstanceOf[IndexShuffleBlockResolver],
bypassMergeSortHandle,
mapId,
context,
env.conf)
case other: BaseShuffleHandle[K @unchecked, V @unchecked, _] =>
new SortShuffleWriter(shuffleBlockResolver, other, mapId, context)
}
}
BypassMergeSortShuffleWriter.writer:
while (records.hasNext()) {
final Product2<K, V> record = records.next();
final K key = record._1();
partitionWriters[partitioner.getPartition(key)].write(key, record._2());
}
SortShuffleWriter.writer:
override def write(records: Iterator[Product2[K, V]]): Unit = {
sorter = if (dep.mapSideCombine) {
new ExternalSorter[K, V, C](
context, dep.aggregator, Some(dep.partitioner), dep.keyOrdering, dep.serializer)
} else {
new ExternalSorter[K, V, V](
context, aggregator = None, Some(dep.partitioner), ordering = None, dep.serializer)
}
sorter.insertAll(records)
....
}
ExternalSorter.insertAll:
def insertAll(records: Iterator[Product2[K, V]]): Unit = {
.....
while (records.hasNext) {
addElementsRead()
kv = records.next()
map.changeValue((getPartition(kv._1), kv._1), update)
maybeSpillCollection(usingMap = true)
}
.....
}
private def getPartition(key: K): Int = {
if (shouldPartition) partitioner.get.getPartition(key) else 0
}
3、compute
(1)、compute 的定义
compute 方法返回该分区的iterator,
在计算链中,无论一个RDD有多么复杂,其最终都会调用内部的compute函数来计算一个分区的数据。
RDD抽象类要求其所有子类都必须实现compute方法。
任何一个 RDD 的任意一个 partition 都首先是通过 compute 函数计算出的,之后才能进行 cache 或 checkpoint
override def compute(split: Partition, context: TaskContext): Iterator[U] =
f(context, split.index, firstParent[T].iterator(split, context))
(2)、compute 的调用
RDD 的
def iterator(split: Partition, context: TaskContext): Iterator[T]
方法用来获取 split 指定的 Partition 对应的数据的迭代器,有了这个迭代器就能一条一条取出数据来按 compute chain 来执行一个个transform 操作。iterator 的实现如下:
final def iterator(split: Partition, context: TaskContext): Iterator[T] = {
if (storageLevel != StorageLevel.NONE) {
SparkEnv.get.cacheManager.getOrCompute(this, split, context, storageLevel)
} else {
computeOrReadCheckpoint(split, context)
}
}
def 前加了 final 说明该函数是不能被子类重写的,其先判断 RDD 的 storageLevel 是否为 NONE,若不是,则尝试从缓存中读取,读取不到则通过计算来获取该 Partition 对应的数据的迭代器;若是,尝试从 checkpoint 中获取 Partition 对应数据的迭代器,若 checkpoint 不存在则通过计算来获取。
private[spark] def computeOrReadCheckpoint(split: Partition, context: TaskContext): Iterator[T] =
{
if (isCheckpointedAndMaterialized) {
firstParent[T].iterator(split, context)
} else {
compute(split, context)
}
}
private[spark] def getOrCompute(partition: Partition, context: TaskContext): Iterator[T] = {
val blockId = RDDBlockId(id, partition.index)
var readCachedBlock = true
// This method is called on executors, so we need call SparkEnv.get instead of sc.env.
SparkEnv.get.blockManager.getOrElseUpdate(blockId, storageLevel, elementClassTag, () => {
readCachedBlock = false
computeOrReadCheckpoint(partition, context)
}) match {
case Left(blockResult) =>
if (readCachedBlock) {
val existingMetrics = context.taskMetrics().inputMetrics
existingMetrics.incBytesRead(blockResult.bytes)
new InterruptibleIterator[T](context, blockResult.data.asInstanceOf[Iterator[T]]) {
override def next(): T = {
existingMetrics.incRecordsRead(1)
delegate.next()
}
}
} else {
new InterruptibleIterator(context, blockResult.data.asInstanceOf[Iterator[T]])
}
case Right(iter) =>
new InterruptibleIterator(context, iter.asInstanceOf[Iterator[T]])
}
}
(3)、compute 的实现
ShuffledRDD
override def compute(split: Partition, context: TaskContext): Iterator[(K, C)] = {
val dep = dependencies.head.asInstanceOf[ShuffleDependency[K, V, C]]
SparkEnv.get.shuffleManager.getReader(dep.shuffleHandle, split.index, split.index + 1, context)
.read()
.asInstanceOf[Iterator[(K, C)]]
}
JdbcRDD:
override def compute(thePart: Partition, context: TaskContext): Iterator[T] = new NextIterator[T]
{
context.addTaskCompletionListener{ context => closeIfNeeded() }
val part = thePart.asInstanceOf[JdbcPartition]
val conn = getConnection()
val stmt = conn.prepareStatement(sql, ResultSet.TYPE_FORWARD_ONLY, ResultSet.CONCUR_READ_ONLY)
val url = conn.getMetaData.getURL
if (url.startsWith("jdbc:mysql:")) {
// setFetchSize(Integer.MIN_VALUE) is a mysql driver specific way to force
// streaming results, rather than pulling entire resultset into memory.
// See the below URL
// dev.mysql.com/doc/connector-j/5.1/en/connector-j-reference-implementation-notes.html
stmt.setFetchSize(Integer.MIN_VALUE)
} else {
stmt.setFetchSize(100)
}
logInfo(s"statement fetch size set to: ${stmt.getFetchSize}")
stmt.setLong(1, part.lower)
stmt.setLong(2, part.upper)
val rs = stmt.executeQuery()
override def getNext(): T = {
if (rs.next()) {
mapRow(rs)
} else {
finished = true
null.asInstanceOf[T]
}
}
}
4、dependency
(1)、dependency的定义
依赖, 用于表示RDD之间的因果关系, 一个dependency表示一个parent rdd, 所以在RDD中使用Seq[Dependency[_]]来表示所有的依赖关系
abstract class Dependency[T] extends Serializable {
def rdd: RDD[T]
}
dependency有以下几种实现:
RDD 依赖是一个 Seq 类型:dependencies_ : Seq[Dependency[_]]
,因为一个 RDD 可以有多个父 RDD。共有两种依赖:
- 窄依赖:父 RDD 的 partition 至多被一个子 RDD partition 依赖
- 宽依赖:父 RDD 的 partition 被多个子 RDD partitions 依赖
NarrowDependency:
abstract class NarrowDependency[T](_rdd: RDD[T]) extends Dependency[T] {
/**
* Get the parent partitions for a child partition.
* @param partitionId a partition of the child RDD
* @return the partitions of the parent RDD that the child partition depends upon
*/
def getParents(partitionId: Int): Seq[Int]
override def rdd: RDD[T] = _rdd
}
窄依赖共有两种实现,一种是一对一的依赖,即 OneToOneDependency
OneToOneDependency:
class OneToOneDependency[T](rdd: RDD[T]) extends NarrowDependency[T](rdd) {
override def getParents(partitionId: Int): List[Int] = List(partitionId)
}
从其 getParents 方法可以看出 OneToOneDependency 的依赖关系下,子 RDD 的 partition 仅依赖于唯一 parent RDD 的相同 index 的 partition。另一种窄依赖的实现是 RangeDependency,它仅仅被 UnionRDD 使用,UnionRDD 把多个 RDD 合成一个 RDD,这些 RDD 是被拼接而成,其 getParents 实现如下:
class RangeDependency[T](rdd: RDD[T], inStart: Int, outStart: Int, length: Int)
extends NarrowDependency[T](rdd) {
override def getParents(partitionId: Int): List[Int] = {
if (partitionId >= outStart && partitionId < outStart + length) {
List(partitionId - outStart + inStart)
} else {
Nil
}
}
}
宽依赖只有一种实现,即 ShuffleDependency
class ShuffleDependency[K: ClassTag, V: ClassTag, C: ClassTag](
@transient private val _rdd: RDD[_ <: Product2[K, V]],
val partitioner: Partitioner,
val serializer: Serializer = SparkEnv.get.serializer,
val keyOrdering: Option[Ordering[K]] = None,
val aggregator: Option[Aggregator[K, V, C]] = None,
val mapSideCombine: Boolean = false)
extends Dependency[Product2[K, V]] {
override def rdd: RDD[Product2[K, V]] = _rdd.asInstanceOf[RDD[Product2[K, V]]]
private[spark] val keyClassName: String = reflect.classTag[K].runtimeClass.getName
private[spark] val valueClassName: String = reflect.classTag[V].runtimeClass.getName
// Note: It's possible that the combiner class tag is null, if the combineByKey
// methods in PairRDDFunctions are used instead of combineByKeyWithClassTag.
private[spark] val combinerClassName: Option[String] =
Option(reflect.classTag[C]).map(_.runtimeClass.getName)
val shuffleId: Int = _rdd.context.newShuffleId()
val shuffleHandle: ShuffleHandle = _rdd.context.env.shuffleManager.registerShuffle(
shuffleId, _rdd.partitions.length, this)
_rdd.sparkContext.cleaner.foreach(_.registerShuffleForCleanup(this))
}
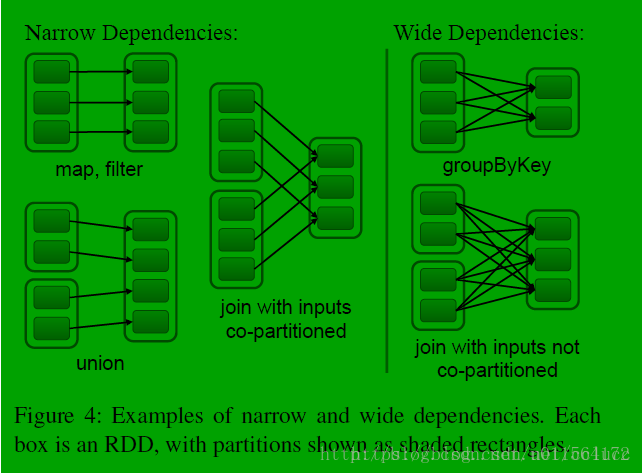
(2)、dependency的调用
到处都在调用
5、preferredLocation
(1)、preferredLocation的定义
对于每个分区而言,返回数据本地化计算的节点。RDD的优先位置,返回的是此RDD的每个partition所存储的位置,这个位置和Spark的调度有关(任务本地化),Spark会根据这个位置信息,尽可能的将任务分配到数据块所存储的位置,以从Hadoop中读取数据生成RDD为例,preferredLocations返回每一个数据块所在的机器名或者IP地址,如果每一个数据块是多份存储的(HDFS副本数),那么就会返回多个机器地址。
要注意的是,并不是每个 RDD 都有 preferedLocation,比如从 Scala 集合中创建的 RDD 就没有,而从 HDFS 读取的 RDD 就有,其 partition 对应的优先位置及对应的 block 所在的各个节点。
数据本地化可以对Spark任务的性能产生重大影响。如果数据和操作数据的代码在一块,计算通常会很快。但是如果数据和代码不在一起,就必须将一方移动到另一方。通常,将序列化的代码块从一个地方发送到另一个地方要比发送数据更快,因为代码的大小比数据要小得多(这也是大数据计算核心思想之一:计算向数据移动)。Spark围绕这个数据本地化的一般原则构建它的调度。
数据本地化是指数据与运行代码之间的距离。根据数据的当前位置,有几个本地化级别。从近到远:
- PROCESS_LOCAL 数据位于与运行代码相同的JVM中。这是最好的地方。
- NODE_LOCAL 数据位于同一节点上。示例:可能在同一个节点上的HDFS中,或者在同一个节点上的另一个executor中。这比PROCESS_LOCAL稍微慢一些,因为数据必须在进程之间来回移动。
- NO_PREF 数据在任何地方都可以同样快速地访问,并且没有本地偏好。
- RACK_LOCAL 数据位于同一台服务器上。数据在同一机架上的不同服务器上,所以需要通过网络发送,通常是通过一个交换机。
- ANAY 数据在网络上的其他地方,而不是在同一个机架上。
Spark倾向于将所有任务都安排在最佳的本地化级别,但这并不总是可行的。在没有任何空闲的executor来处理未处理数据的情况下,Spark将切换到较低的本地化级别。有两种选择:a)等到一个繁忙的CPU释放出来,在同一台服务器上启动一个数据任务,或者,b)立即在一个需要移动数据的较远的地方启动一个新的任务。
Spark通常做的是等待一个繁忙的CPU释放的希望。一旦超时过期,它就开始将数据从远处移动到空闲CPU。等待时间可通过以下配置参数配置:
spark.locality.wait 默认3s
spark.locality.wait.process 默认与spark.locality.wait保持一致,可以单独指定
spark.locality.wait.node 默认与spark.locality.wait保持一致,可以单独指定
spark.locality.wait.rack 默认与spark.locality.wait保持一致,可以单独指定
配置demo:spark.locality.wait.process:10,spark.locality.wait.node:5,spark.locality.wait.rack:3
(2)、preferredLocation的实现
final def preferredLocations(split: Partition): Seq[String] = {
checkpointRDD.map(_.getPreferredLocations(split)).getOrElse {
getPreferredLocations(split)
}
}
ShuffledRDD:
override protected def getPreferredLocations(partition: Partition): Seq[String] = {
val tracker = SparkEnv.get.mapOutputTracker.asInstanceOf[MapOutputTrackerMaster]
val dep = dependencies.head.asInstanceOf[ShuffleDependency[K, V, C]]
tracker.getPreferredLocationsForShuffle(dep, partition.index)
}
https://blog.youkuaiyun.com/u011564172/article/details/53611109