一、深度神经网络
简介
深度神经网络和基本神经网络的差别在于规模大小。至少包含两层隐含层的神经网络被称为深度神经网络。
实现
主要由Lasagne和nolearn两个库来完成规模更大,定制化程度更高的神经网络。这两个库依赖于擅长处理数学表达式的Theano库。
Theano库:创建和运行数学表达式的工具。在Theano中,我们定义函数要做什么而不是怎么做 ;Theano能只在需要时对表达式求值,而不是定义式。
Lasagne库:专门用来构建神经网络的,使用Theano进行计算。Lasagne实现了内置网络层、删除层、噪音层、卷积层和池化层等。
卷积层使用少量相 互连接的神经元,分析一部分输入值(比如我们这里的一张图像),便于神经网络实现对数据的 标准转换,比如对图像数据的转换 。
池 化层接收某个区域最大输出值,可以降低图像中的微小变动带来的噪音,减少(down-sample, 降采样)信息量,这样后续各层所需工作量也会相应减少 。
用Lasagne创建一个简单的卷积神经网络
# 1、加载数据集
import numpy as np
from sklearn.datasets import load_iris
iris = load_iris()
X = iris.data.astype(np.float32)
# Lasagne对数据类型有特殊要求,因此,需要把类别值转换为int32类型(在原始数据集中用int64类型存储)
y_true = iris.target.astype(np.int32)
# 划分训练集和验证集
from sklearn.model_selection import train_test_split
X_train, X_test, y_train, y_test = train_test_split(X, y_true, random_state=14)
# 2、创建卷积神经网络(数据集有4个特征和3个类别)
import lasagne
input_layer = lasagne.layers.InputLayer(shape=(10, X.shape[1]))
hidden_layer = lasagne.layers.DenseLayer(input_layer, num_units=12, nonlinearity=lasagne.nonlinearities.sigmoid)
output_layer = lasagne.layers.DenseLayer(hidden_layer, num_units=3, nonlinearity=lasagne.nonlinearities.softmax)
# 定义几个Theano训练函数训练创建的网络。
import theano.tensor as T
net_input = T.matrix('net_input')
net_output = output_layer.get_output(net_input)
true_output = T.ivector('true_output')
# 定义损失函数
loss = T.mean(T.nnet.categorical_crossentropy(net_output, true_output))
# 定义修改网络权重的函数
all_params = lasagne.layers.get_all_params(output_layer)
updates = lasagne.updates.sgd(loss, all_params, learning_rate=0.1)
# 创建两个Theano函数,一个训练网络,一个获取网络输出
import theano
train = theano.function([net_input, true_output], loss, updates=updates)
get_output = theano.function([net_input], net_output)
for n in range(1000):
train(X_train, y_train)
y_output = get_output(X_test)
import numpy as np
y_pred = np.argmax(y_output, axis=1)
from sklearn.metrics import f1_score
print(f1_score(y_test, y_pred))
三、用nolearn实现神经网络
nolearn对Lasagne进行了封装,与Lasasgne相比,使用nolearn无法对 一些细节进行调整。
from PIL import Image, ImageDraw, ImageFont
from skimage import transform as tf
# 创建生成验证码的函数
def create_captcha(text, shear=0, size=(100, 30)):
im = Image.new("L", size, "black")
draw = ImageDraw.Draw(im)
font = ImageFont.truetype('./data/Coval-Regular.otf', 22)
draw.text((0, 0), text, fill=1, font=font)
# 把PIL图像转换为numpy数组
image = np.array(im)
affine_tf = tf.AffineTransform(shear=shear)
image = tf.warp(image, affine_tf)
return image / image.max()
image = create_captcha("GENE", shear=0.5)
from matplotlib import pyplot as plt
plt.imshow(image, cmap='Greys')
plt.show()
from skimage.measure import label, regionprops
def segment_image(image):
labeled_image = label(image > 0)
subimages = []
for region in regionprops(labeled_image):
start_x, start_y, end_x, end_y = region.bbox
subimages.append(image[start_x:end_x, start_y:end_y])
if len(subimages) == 0:
return [image, ]
return subimages
# 1、创建数据集
from sklearn.utils import check_random_state
random_state = check_random_state(14)
letters = list("ABCDEFGHIJKLMNOPQRSTUVWXYZ")
shear_values = np.arange(0, 0.5, 0.05)
def generate_sample(random_state=None):
random_state = check_random_state(random_state)
letter = random_state.choice(letters)
shear = random_state.choice(shear_values)
return create_captcha(letter, shear=shear, size=(20, 20)), letters.index(letter)
# 多数据集创建
dataset, targets = zip(*(generate_sample(random_state) for i in range(3000)))
dataset = np.array(dataset, dtype='float')
targets = np.array(targets)
# 数据集调整
from sklearn.preprocessing import OneHotEncoder
onehot = OneHotEncoder()
y = onehot.fit_transform(targets.reshape(targets.shape[0], 1))
y = y.todense()
# 训练集调整
from skimage.transform import resize
dataset = np.array([resize(segment_image(sample)[0], (20, 20)) for sample in dataset])
X = dataset.reshape((dataset.shape[0], dataset.shape[1] * dataset.shape[2]))
X = X / X.max()
X = X.astype(np.float32)
# 数据集分割
from sklearn.model_selection import train_test_split
X_train, X_test, y_train, y_test = train_test_split(X, y, train_size=0.9)
# 2、创建神经网络
from lasagne import layers
layers = [
('input', layers.InputLayer),
('hidden', layers.DenseLayer),
('output', layers.DenseLayer),
]
from lasagne import updates
from nolearn.lasagen import NeuralNet
from lasagne.nonlinearities import sigmoid, softmax
net1 = NeuralNet(
layers=layers,
input_shape=X.shape,
hidden_num_units=100,
output_num_units=26,
hidden_nonlinearity=sigmoid,
output_nonlinearity=softmax,
hidden_b=np.zeros((100,), dtype=np.float32),
update=updates.momentum,
update_learing_rate=0.9,
update_momentum=0.1,
regression=True,
max_epochs=1000,
)
# 3、训练+评估
net1.fit(X_train, y_train)
y_pred = net1.predict(X_test)
y_pred = y_pred.argmax(axis=1)
assert len(y_pred) == len(X_test)
if len(y_test.shape) > 1:
y_test = y_test.argmax(axis=1)
print(f1_score(y_test, y_pred))
四、CIFAR图像分类
# 1.查看cifar的batch1数据集
batch1_filename = './data/cifar-10-batches-py/data_batch_1'
import pickle
# 图像数据文件格式为pickle,pickle文件是Python2生成的,而我们要用Python3打开。所以在打开时需要把编码设置成latin
def unpickle(filename):
with open(filename, 'rb') as fo:
return pickle.load(fo, encoding='latin1')
batch1 = unpickle(batch1_filename)
image_index = 100
image = batch1['data'][image_index]
image = image.reshape((32, 32, 3), order='F')
import numpy as np
image = np.rot90(image, -1)
from matplotlib import pyplot as plt
plt.imshow(image)
plt.show()
# 2.加载cifar数据集
import numpy as np
batches = []
for i in range(1, 6):
batch_filename = './data/cifar-10-batches-py/data_batch_{}'.format(i)
batches.append(unpickle(batch_filename))
X = np.vstack([batch['data'] for batch in batches])
# 像素值归一化,并转换为GPU虚拟机唯一支持的数据类型
X = np.array(X) / X.max()
X = X.astype(np.float32)
from sklearn.preprocessing import OneHotEncoder
y = np.hstack(batch['labels'] for batch in batches).flatten()
y = OneHotEncoder().fit_transform(y.reshape(y.shape[0], 1)).todense()
y = y.astype(np.float32)
from sklearn.model_selection import train_test_split
X_train, X_test, y_train, y_test = train_test_split(X, y, test_size=0.2)
X_train = X_train.reshape(-1, 3, 32, 32)
X_test = X_test.reshape(-1, 3, 32, 32)
# 3.使用nolearn创建神经网络
from lasagne import layers
layers = [
('input', layers.InputLayer),
('conv1', layers.Conv2DLayer),
('pool1', layers.MaxPool2DLayer),
('conv2', layers.Conv2DLayer),
('pool2', layers.MaxPool2DLayer),
('conv3', layers.Conv2DLayer),
('pool3', layers.MaxPool2DLayer),
('hidden4', layers.DenseLayer),
('hidden5', layers.DenseLayer),
('output', layers.DenseLayer),
]
from nolearn.lasagen import NeuralNet
nnet = NeuralNet(layers=layers,
input_shape=(None, 3, 32, 32),
conv1_num_filters=32,
conv1_filter_size=(3, 3),
conv2_num_filters=64,
conv2_filter_size=(2, 2),
conv3_num_filters=128,
conv3_filter_size=(2, 2),
pool1_ds=(2, 2),
pool2_ds=(2, 2),
pool3_ds=(2, 2),
hidden4_num_units=500,
hidden5_num_units=500,
output_num_units=10,
output_nonlinearity=softmax,
update_learning_rate=0.01,
update_momentum=0.9,
regression=True,
max_epochs=100,
verbose=1)
# 4.训练并评估
nnet.fit(X_train, y_train)
from sklearn.metrics import f1_score
y_pred = nnet.predict(X_test)
print(f1_score(y_test.argmax(axis=1), y_pred.argmax(axis=1)))
五、使用kersa实现神经网络对MNIST分类
这里我们使用kersa提供MNIST数据集,训练集为 60,000 张28×28=78428×28=784像素灰度图像,测试集为 10,000 同规格图像,总共 10 类数字标签。
# 1.加载数据集
from keras.datasets import mnist
(x_train, y_train), (x_test, y_test) = mnist.load_data()
from matplotlib import pyplot as plt
plt.figure(figsize=(12, 10))
x, y = 8, 6
for i in range(x*y):
plt.subplot(y, x, i+1)
plt.imshow(x_train[i], interpolation='nearest')
plt.show()
# 数据集变换,x->(6000, 784),y->(6000,1)
import keras
x_train = x_train.reshpe(60000, 784)
x_test = x_test.reshape(60000, 784)
x_train = x_train.astype('float32')
x_test = x_test.astype('float32')
x_train /= 255
x_test /= 255
y_train = keras.utils.to_categorical(y_train, 10)
y_test = keras.utils.to_categorical(y_test, 10)
# 2.创建网络
from keras.models import Sequential
from keras.layers import Dense, Dropout
model = Sequential()
# 创建输入层
model.add(Dense(512, activation='relu', input_shape=(784,)))
model.add(Dropout(0.2))
# 创建layer2
model.add(Dense(512, activation='relu'))
model.add(Dropout(0.2))
# 创建输出层
model.add(Dense(10, acitivation='softmax'))
# 网络模型的介绍
print(model.summary())
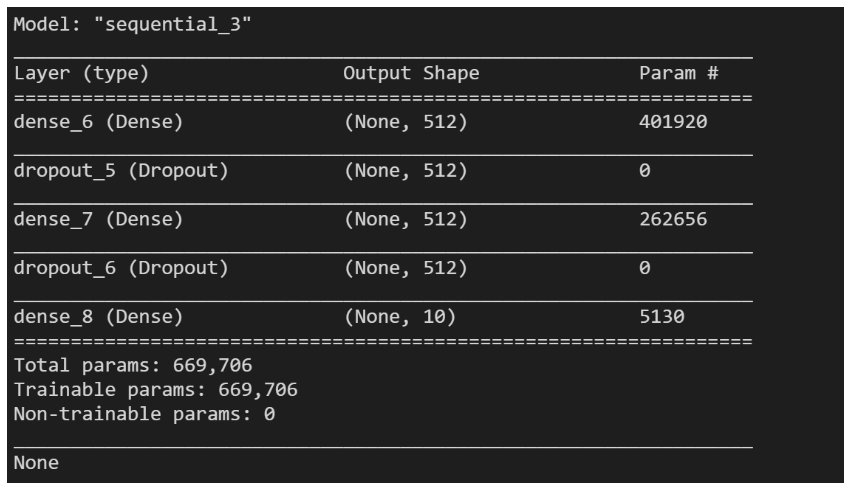
Dropout应用于输入。Dropout 包括在训练中每次更新时, 将输入单元的按比率随机设置为 0, 这有助于防止过拟合。简单点来说,他就是这样的,随机(按比率)将某一个输入值变成0,这样他就没办法向下一层传递信息。
接着我们就来讲一下如何配置这个模型,在kersa中,配置一个模型至少需要两个参数loss和optimizer。其中loss就是损失函数,optimizer就是优化器,也就是前篇博客提到的优化方法,比如说梯度下降法,牛顿法等等,metrics代表在训练和测试期间的模型评估标准。
# 配置模型
from keras.optimizers import RMSprop
model.compile(loss='categorical_crossentropy',
optimizer=RMSprop(),
metrics=['accuracy'])
history = model.fit(x_train, y_train,
batch_size=128,
epochs=32,
verbose=1,
validation_data=(x_test, y_test))
score = model.evaluate(x_test, y_test, verbose=0)
print("Test loss:", score[0])
print("Test accuracy", score[1])

我们将每一次轮训练以及测试对应的loss和accuracy画出来:
# 绘制训练过程中训练集和测试集合的准确率值
plt.plot(history.history['accuracy'])
plt.plot(history.history['val_accuracy'])
plt.title('Model accuracy')
plt.ylabel('Accuracy')
plt.xlabel('Epoch')
plt.legend(['Train', 'Test'], loc='upper left')
plt.show()
# 绘制训练过程中训练集和测试集合的损失值
plt.plot(history.history['loss'])
plt.plot(history.history['val_loss'])
plt.title('Model loss')
plt.ylabel('Loss')
plt.xlabel('Epoch')
plt.legend(['Train', 'Test'], loc='upper left')
plt.show()
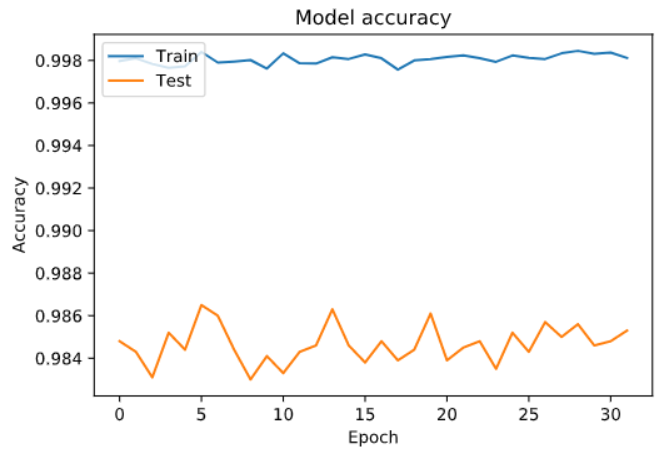
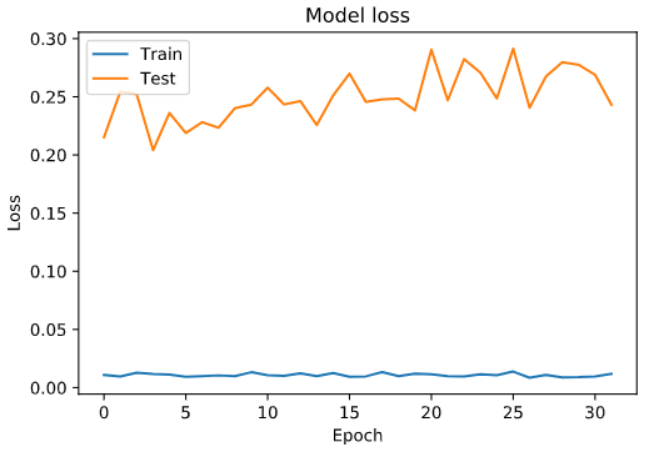
六、小结
使用Lasagne和nolearn库来构建神经网络,很多数据处理工作交由Theano来做。
使用kersa实现神经网络。