import torch
from torch import nn as nn
import torch.nn.functional as F
from ultralytics.nn.modules.conv import autopad, Conv, RepConv
class RepBottleneck(nn.Module):
"""Rep bottleneck."""
def __init__(self, c1, c2, shortcut=True, g=1, k=(3, 3), e=0.5):
"""Initializes a RepBottleneck module with customizable in/out channels, shortcut option, groups and expansion
ratio.
"""
super().__init__()
c_ = int(c2 * e) # hidden channels
self.cv1 = RepConv(c1, c_, k[0], 1)
self.cv2 = Conv(c_, c2, k[1], 1, g=g)
self.add = shortcut and c1 == c2
def forward(self, x):
"""Forward pass through RepBottleneck layer."""
return x + self.cv2(self.cv1(x)) if self.add else self.cv2(self.cv1(x))
class RepCSP(nn.Module):
"""Rep CSP Bottleneck with 3 convolutions."""
def __init__(self, c1, c2, n=1, shortcut=True, g=1, e=0.5):
"""Initializes RepCSP layer with given channels, repetitions, shortcut, groups and expansion ratio."""
super().__init__()
c_ = int(c2 * e) # hidden channels
self.cv1 = Conv(c1, c_, 1, 1)
self.cv2 = Conv(c1, c_, 1, 1)
self.cv3 = Conv(2 * c_, c2, 1) # optional act=FReLU(c2)
self.m = nn.Sequential(
*(RepBottleneck(c_, c_, shortcut, g, e=1.0) for _ in range(n))
)
def forward(self, x):
"""Forward pass through RepCSP layer."""
return self.cv3(torch.cat((self.m(self.cv1(x)), self.cv2(x)), 1))
class RepNCSPELAN4(nn.Module):
"""CSP-ELAN."""
def __init__(self, c1, c2, c3, c4, n=1):
"""Initializes CSP-ELAN layer with specified channel sizes, repetitions, and convolutions."""
super().__init__()
self.c = c3 // 2
self.cv1 = Conv(c1, c3, 1, 1)
self.cv2 = nn.Sequential(RepCSP(c3 // 2, c4, n), Conv(c4, c4, 3, 1))
self.cv3 = nn.Sequential(RepCSP(c4, c4, n), Conv(c4, c4, 3, 1))
self.cv4 = Conv(c3 + (2 * c4), c2, 1, 1)
def forward(self, x):
"""Forward pass through RepNCSPELAN4 layer."""
y = list(self.cv1(x).chunk(2, 1))
y.extend((m(y[-1])) for m in [self.cv2, self.cv3])
return self.cv4(torch.cat(y, 1))
def forward_split(self, x):
"""Forward pass using split() instead of chunk()."""
y = list(self.cv1(x).split((self.c, self.c), 1))
y.extend(m(y[-1]) for m in [self.cv2, self.cv3])
return self.cv4(torch.cat(y, 1))
class ADown(nn.Module):
"""ADown."""
def __init__(self, c1, c2):
"""Initializes ADown module with convolution layers to downsample input from channels c1 to c2."""
super().__init__()
self.c = c2 // 2
self.cv1 = Conv(c1 // 2, self.c, 3, 2, 1)
self.cv2 = Conv(c1 // 2, self.c, 1, 1, 0)
def forward(self, x):
"""Forward pass through ADown layer."""
x = torch.nn.functional.avg_pool2d(x, 2, 1, 0, False, True)
x1, x2 = x.chunk(2, 1)
x1 = self.cv1(x1)
x2 = torch.nn.functional.max_pool2d(x2, 3, 2, 1)
x2 = self.cv2(x2)
return torch.cat((x1, x2), 1)
class SPPELAN(nn.Module):
"""SPP-ELAN."""
def __init__(self, c1, c2, c3, k=5):
"""Initializes SPP-ELAN block with convolution and max pooling layers for spatial pyramid pooling."""
super().__init__()
self.c = c3
self.cv1 = Conv(c1, c3, 1, 1)
self.cv2 = nn.MaxPool2d(kernel_size=k, stride=1, padding=k // 2)
self.cv3 = nn.MaxPool2d(kernel_size=k, stride=1, padding=k // 2)
self.cv4 = nn.MaxPool2d(kernel_size=k, stride=1, padding=k // 2)
self.cv5 = Conv(4 * c3, c2, 1, 1)
def forward(self, x):
"""Forward pass through SPPELAN layer."""
y = [self.cv1(x)]
y.extend(m(y[-1]) for m in [self.cv2, self.cv3, self.cv4])
return self.cv5(torch.cat(y, 1))
class Silence(nn.Module):
"""Silence."""
def __init__(self):
"""Initializes the Silence module."""
super(Silence, self).__init__()
def forward(self, x):
"""Forward pass through Silence layer."""
return x
class CBLinear(nn.Module):
"""CBLinear."""
def __init__(self, c1, c2s, k=1, s=1, p=None, g=1):
"""Initializes the CBLinear module, passing inputs unchanged."""
super(CBLinear, self).__init__()
self.c2s = c2s
self.conv = nn.Conv2d(c1, sum(c2s), k, s, autopad(k, p), groups=g, bias=True)
def forward(self, x):
"""Forward pass through CBLinear layer."""
outs = self.conv(x).split(self.c2s, dim=1)
return outs
class CBFuse(nn.Module):
"""CBFuse."""
def __init__(self, idx):
"""Initializes CBFuse module with layer index for selective feature fusion."""
super(CBFuse, self).__init__()
self.idx = idx
def forward(self, xs):
"""Forward pass through CBFuse layer."""
target_size = xs[-1].shape[2:]
res = [
F.interpolate(x[self.idx[i]], size=target_size, mode="nearest")
for i, x in enumerate(xs[:-1])
]
out = torch.sum(torch.stack(res + xs[-1:]), dim=0)
return out
一、__all__ = ("RepNCSPELAN4", "ADown", "SPPELAN", "CBFuse", "CBLinear", "Silence")
1、加入task.py文件

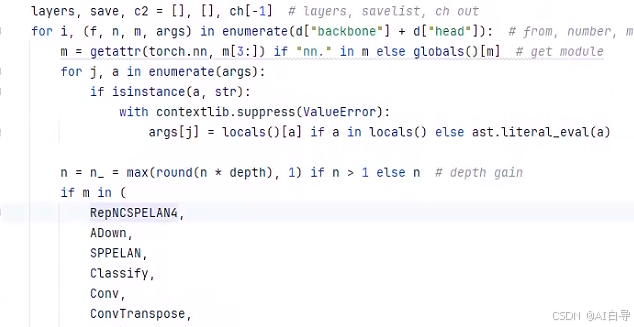
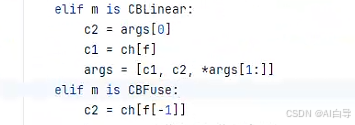
elif m is cBLinear:
c2 = args[0]
c1 = ch[f]
args = [c1,c2,*args[1:]]
elif m is CBFuse:
c2 = ch[f[-1]]
2、配置文件
# parameters
nc: 80 # number of classes
# gelan backbone
backbone:
- [-1, 1, Conv, [64, 3, 2]] # 0-P1/2
- [-1, 1, Conv, [128, 3, 2]] # 1-P2/4
- [-1, 1, RepNCSPELAN4, [256, 128, 64, 1]] # 2
- [-1, 1, ADown, [256]] # 3-P3/8
- [-1, 1, RepNCSPELAN4, [512, 256, 128, 1]] # 4
- [-1, 1, ADown, [512]] # 5-P4/16
- [-1, 1, RepNCSPELAN4, [512, 512, 256, 1]] # 6
- [-1, 1, ADown, [512]] # 7-P5/32
- [-1, 1, RepNCSPELAN4, [512, 512, 256, 1]] # 8
- [-1, 1, SPPELAN, [512, 256]] # 9
head:
- [-1, 1, nn.Upsample, [None, 2, "nearest"]]
- [[-1, 6], 1, Concat, [1]] # cat backbone P4
- [-1, 1, RepNCSPELAN4, [512, 512, 256, 1]] # 12
- [-1, 1, nn.Upsample, [None, 2, "nearest"]]
- [[-1, 4], 1, Concat, [1]] # cat backbone P3
- [-1, 1, RepNCSPELAN4, [256, 256, 128, 1]] # 15 (P3/8-small)
- [-1, 1, ADown, [256]]
- [[-1, 12], 1, Concat, [1]] # cat head P4
- [-1, 1, RepNCSPELAN4, [512, 512, 256, 1]] # 18 (P4/16-medium)
- [-1, 1, ADown, [512]]
- [[-1, 9], 1, Concat, [1]] # cat head P5
- [-1, 1, RepNCSPELAN4, [512, 512, 256, 1]] # 21 (P5/32-large)
- [[15, 18, 21], 1, Detect, [nc]] # Detect(P3, P4, P5)