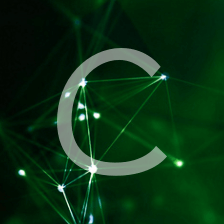
paper
文章平均质量分 92
二叉树不是树_ZJY
这个作者很懒,什么都没留下…
展开
-
【P18】Refining Network Intents for Self-Driving Networks
SIGCOMMAugust 2018Abstract现有的IBN研究方案未能利用网络运营商的知识和反馈来验证或改进意图的转换。本文介绍了一个新的意图重塑过程,它使用机器学习和来自运营商的反馈,将运营商的话语翻译成网络配置。重塑过程使用一个序列到序列的学习模型来从自然语言中提取意图,并使用操作员的反馈来改进学习。原型==使用自然语言与网络操作员互动,再将操作员的输入翻译成中间表示(其结构足够便于精确翻译),最后翻译成SDN规则==。实验表明,对于有5000个条目的数据集,实现了0.99的相关系数.原创 2021-08-19 01:05:25 · 409 阅读 · 0 评论 -
【P17】Neural Machine Translation with Monolingual Translation Memory
ACL 2021Abstract1 简介2 相关工作2.1 TM-augmented NMTFeng等人(2017)用双语词典增强了NMT,以解决不经常出现的单词翻译问题。Gu等人(2018)提出了一个模型,检索与测试源句相似的例子,并用键值记忆网络对检索的源-目标对进行编码。Cao和Xiong(2018);Cao等人(2019)使用门控机制来平衡翻译记忆的影响。Zhang等人(2018)通过检索n-grams和上调检索n-grams的概率来提出引导模型。Bulte和Tezcan(2.原创 2021-08-09 12:54:16 · 627 阅读 · 0 评论 -
【P16】GRAPPA: Grammar-Augmented Pre-Training for Table Semantic Parsing
GRAPPA: Grammar-Augmented Pre-Training for Table Semantic Parsing原创 2021-04-18 20:02:30 · 1423 阅读 · 0 评论 -
【P15】Structure-Grounded Pretraining for Text-to-SQL
Structure-Grounded Pretraining for Text-to-SQLAbstract1. Introduction2. Related Works3. Methodology3.1 Context-free Grammar Pre-training(GP)3.2 Question-Schema Serialization and Encoding4. Experimental ResultsNAACL,2021https://arxiv.org/pdf/2010.12773.p原创 2021-04-14 15:12:47 · 473 阅读 · 0 评论 -
【P14】GP: Context-free Grammar Pre-training for Text-to-SQL Parsers
GP: Context-free Grammar Pre-training for Text-to-SQL ParsersAbstract1. Introduction2. Related Works3. Methodology3.1 Context-free Grammar Pre-training(GP)3.2 Question-Schema Serialization and Encoding4. Experimental ResultsJournal of Artificial Intellig原创 2021-03-09 16:07:36 · 412 阅读 · 0 评论 -
【P13】SeqGenSQL - A Robust Sequence Generation Model for Structured
SeqGenSQL - A Robust Sequence Generation Model for StructuredAbstract3 Model3.1 Question Augmentation3.2 Reversed Trainer Model(Data Augmentation)3.3 Gated Extraction Network3.4 Execution Guided Inferencehttps://arxiv.org/abs/2011.03836Implementation: h原创 2021-03-06 22:49:42 · 332 阅读 · 1 评论 -
【P12】Semantic Parsing with Semi-Supervised Sequential Autoencoders
Semantic Parsing with Semi-Supervised Sequential Autoencoders1 Introduction2 Model1 Introduction在本文中,我们专注于学习从输入序列x到输出序列y的映射,在这些领域中,后者很容易获得,但以(x,y)对形式的注释是稀疏或昂贵的,并提出了一种新的架构,以适应序列转导任务的半监督训练。为此,我们用自动编码目标增强了转导目标(x 7 y),其中输入序列被视为一个潜伏变量(y 7 x 7 y),从而可以从标记的对和未配对原创 2021-02-28 15:13:53 · 407 阅读 · 1 评论 -
【P11】X-SQL: reinforce schema representation with context
X-SQL: reinforce schema representation with contextAbstract1 Introduction2 Neural ArchitectureAbstract针对 NL-to-SQL 的解析问题,本论文提出了一种新的网络体系结构X-SQL。利用bert风格的预训练模型(MT-DNN)的上下文输出来增强结构模式表示,并**结合类型信息( type information)**来学习用于下游任务的新模式表示。1 IntroductionX-SQL 从以下三个原创 2021-02-06 00:34:12 · 704 阅读 · 0 评论 -
【P10】Bridging Textual and Tabular Data for Cross-Domain Text-to-SQL Semantic Parsing
Bridging Textual and Tabular Data forCross-Domain Text-to-SQL Semantic ParsingAbstract1 Introduction1.1 任务介绍1.2 两个问题2 Related work2.1 expression fragmentation 表达式分片问题2.2 operand-context separation 操作数-上下文分离问题3 EPT: Expression-Pointer Transformer3.1 Input原创 2021-02-05 21:52:46 · 938 阅读 · 0 评论 -
Memory Network
Memory Network总结:Memory Networks相关的论文和实现深度学习和自然语言处理中的attention和memory机制原创 2021-01-31 21:05:45 · 484 阅读 · 1 评论 -
【P9】Point to the Expression:Solving Algebraic Word Problems using the Expression-Pointer Transformer
Point to the Expression: Solving Algebraic Word Problems using the Expression-Pointer Transformer Model原创 2021-01-22 17:51:30 · 514 阅读 · 0 评论 -
【P8】Mention Extraction and Linking for SQL Query Generation
Mention Extraction and Linking for SQL Query GenerationAbstract1 Introduction2 Method2.1 Extractor2.2 Schema Linking as Matching2.3 AGG prediction enhancement2.4 Automatic Annotation via Alignment3 Experiment3.1 Results4 Related Work5 Conclusion and Future原创 2020-11-20 23:00:08 · 950 阅读 · 4 评论 -
【P7】CodeBERT: A Pre-Trained Model for Programming and Natural Languages
【P7】CodeBERT: A Pre-Trained Model for Programming and Natural LanguagesAbstract1 Introduction2 Background2.1 Pre-Trained Models in NLP2.2 多模态Pre-Trained ModelsAbstract我们提出了CodeBERT,一个用于编程语言(PL)和自然语言(NL)的双模态预训练模型。CodeBERT可以学习通用的表示方法,支持下游NL-PL应用,如自然语言代码搜索、原创 2020-11-15 18:55:16 · 2441 阅读 · 0 评论 -
【P5】Attention Is All You Need
Attention Is All You Need1 模型结构EncoderDecoder2 Attention机制2.1 Scaled Dot-Product Attention2.2 Multi-Head Attention33.1 Positional Encodings1 模型结构 Transformer 是基于经典的Encoder-Decoder架构:Encoder 的输入由 Input Embedding 和 Positional Embedding 求和组成。Decoder原创 2020-11-13 00:49:55 · 487 阅读 · 0 评论 -
【P4】Safely and Automatically Updating In-Network ACL Configurations with Intent Language
【P4】Safely and Automatically Updating In-Network ACL Configurations with Intent LanguageABSTRACT1 INTRODUCTION1.1 Our Approach: Jinjing2 BACKGROUND AND MOTIVATION2.1 Background: ACL2.2 动机和目标3 JINJING OVERVIEW3.1 Intent Language: LAI3.2 A Running Example3.3原创 2020-11-08 22:43:53 · 824 阅读 · 0 评论 -
意图网络-意图获取和转译
意图网络-意图转译原创 2020-11-05 16:02:43 · 1952 阅读 · 0 评论 -
【P3】IBN(意图网络)的最新进展
[P3]Recent Advances in Intent-Based Networking: A SurveyAbstractI. INTRODUCTIONII. IBN 标准化工作III. INTENT-BASED PLATFORMSIV. DISCUSSIONS, CHALLENGES AND FUTURE DIRECTIONSV. CONCLUSIONSAbstractI. INTRODUCTION目前,大多数运营商都涉及复杂的网络配置步骤,其中配置和执行更新与底层异构和多样化的基础设施密切相原创 2020-11-05 15:58:49 · 1348 阅读 · 0 评论 -
【P2】A Design of IoT Device Configuration Translator for Intent-Based IoT-Cloud Services
A Design of IoT Device Configuration Translator for Intent-Based IoT-Cloud ServicesAbstractI. INTRODUCTIONII. RELATED WORKAbstract本文提出了一种用于IoT云服务的IoT设备配置转换器,使不具备IoT环境专业知识的用户能够有效配置其IoT设备。用户的高级配置(基于自然语言)通过NETCONF(网络配置协议)传递给IoT-cloud平台上的翻译器。翻译器使用自动机理论和数据原创 2020-11-04 09:51:49 · 538 阅读 · 0 评论 -
【P1】Incorporating External Knowledge through Pre-training for Natural Language to Code Generation
【P1】Incorporating External Knowledge through Pre-training for Natural Language to Code GenerationAbstract1 Introduction2 Approach2.1 整体框架2.2 挖掘NL-code pairs2.3 API文档2.4 Re-sampling API Knowledge3 ExperimentsAbstract 开放领域代码生成致力于从自然语言生成通用变成语言(例如Python)。本文原创 2020-10-30 22:09:16 · 835 阅读 · 0 评论