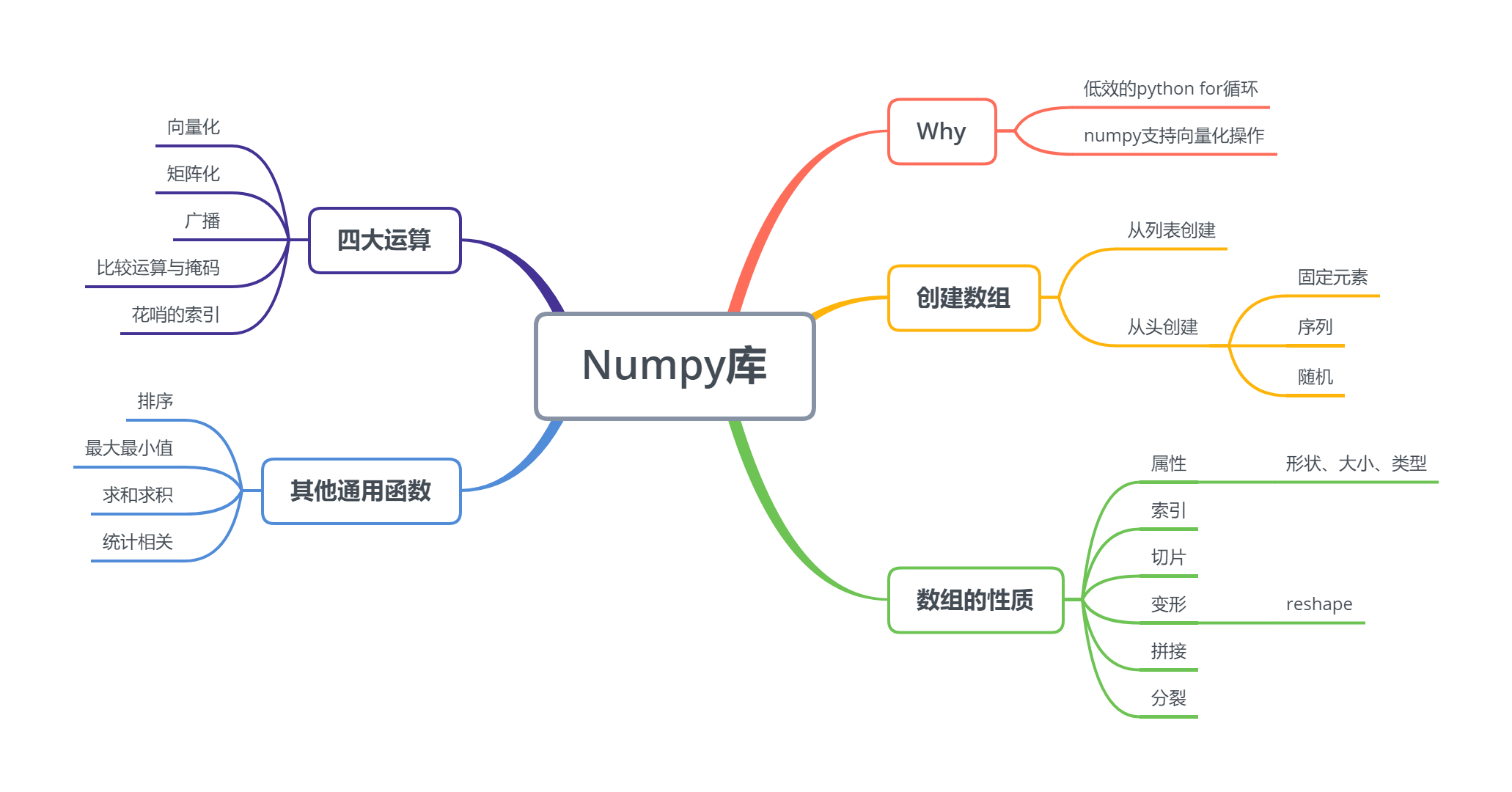
第十一章 Numpy库
11.1 为什么要用Numpy
11.1.1 低效的Python for循环
【例】 求100万个数的倒数
def compute_reciprocals(values):
res = []
for value in values: # 每遍历到一个元素,就要判断其类型,并查找适用于该数据类型的正确函数
res.append(1/value)
return res
values = list(range(1, 1000000))
%timeit compute_reciprocals(values)
145 ms ± 13.7 ms per loop (mean ± std. dev. of 7 runs, 10 loops each)
%timeit :ipython中统计运行时间的魔术方法(多次运行取平均值)
import numpy as np
values = np.arange(1, 1000000)
%timeit 1/values
5.99 ms ± 33.9 µs per loop (mean ± std. dev. of 7 runs, 100 loops each)
实现相同计算,Numpy的运行速度是Python循环的25倍,产生了质的飞跃
11.1.2 Numpy为什么如此高效
Numpy 是由C语言编写的
1、编译型语言VS解释型语言
C语言执行时,对代码进行整体编译,速度更快
2、连续单一类型存储VS分散多变类型存储
(1)Numpy数组内的数据类型必须是统一的,如全部是浮点型,而Python列表支持任意类型数据的填充
(2)Numpy数组内的数据连续存储在内存中,而Python列表的数据分散在内存中
这种存储结构,与一些更加高效的底层处理方式更加的契合
3、多线程VS线程锁
Python语言执行时有线程锁,无法实现真正的多线程并行,而C语言可以
11.1.3 什么时候用Numpy
在数据处理的过程中,遇到使用“Python for循环” 实现一些向量化、矩阵化操作的时候,要优先考虑用Numpy
如: 1、两个向量的点乘
2、矩阵乘法
11.2 Numpy数组的创建
11.2.1 从列表开始创建
import numpy as np
x = np.array([1, 2, 3, 4, 5])
print(x)
[1 2 3 4 5]
print(type(x))
print(x.shape)
<class 'numpy.ndarray'>
(5,)
- 设置数组的数据类型
x = np.array([1, 2, 3, 4, 5], dtype="float32")
print(x)
print(type(x[0]))
[1. 2. 3. 4. 5.]
<class 'numpy.float32'>
- 二维数组
x = np.array([[1, 2, 3],
[4, 5, 6],
[7, 8, 9]])
print(x)
print(x.shape)
[[1 2 3]
[4 5 6]
[7 8 9]]
(3, 3)
11.2.2 从头创建数组
(1)创建长度为5的数组,值都为0
np.zeros(5, dtype=int)
array([0, 0, 0, 0, 0])
(2)创建一个2*4的浮点型数组,值都为1
np.ones((2, 4), dtype=float)
array([[1., 1., 1., 1.],
[1., 1., 1., 1.]])
(3)创建一个3*5的数组,值都为8.8
np.full((3, 5), 8.8)
array([[8.8, 8.8, 8.8, 8.8, 8.8],
[8.8, 8.8, 8.8, 8.8, 8.8],
[8.8, 8.8, 8.8, 8.8, 8.8]])
(4)创建一个3*3的单位矩阵
np.eye(3)
array([[1., 0., 0.],
[0., 1., 0.],
[0., 0., 1.]])
(5)创建一个线性序列数组,从1开始,到15结束,步长为2
np.arange(1, 15, 2)
array([ 1, 3, 5, 7, 9, 11, 13])
(6)创建一个4个元素的数组,这四个数均匀的分配到0~1
np.linspace(0, 1, 4)
array([0. , 0.33333333, 0.66666667, 1. ])
(7)创建一个10个元素的数组,形成1~10^9的等比数列
np.logspace(0, 9, 10)
array([1.e+00, 1.e+01, 1.e+02, 1.e+03, 1.e+04, 1.e+05, 1.e+06, 1.e+07,
1.e+08, 1.e+09])
(8)创建一个3*3的,在0~1之间均匀分布的随机数构成的数组
np.random.random((3,3))
array([[0.24347952, 0.41715541, 0.41363866],
[0.44869706, 0.18128167, 0.18568051],
[0.05705023, 0.0689205 , 0.74837661]])
(9)创建一个3*3的,均值为0,标准差为1的正态分布随机数构成的数组
np.random.normal(0, 1, (3,3))
array([[-0.38530465, 0.17474932, 0.31129291],
[ 1.61626424, -2.18883854, 0.54043825],
[-0.9141666 , -0.03804043, -0.6645122 ]])
(10)创建一个3*3的,在[0,10)之间随机整数构成的数组
np.random.randint(0, 10, (3,3))
array([[9, 1, 9],
[0, 3, 9],
[8, 5, 4]])
(11)随机重排列
x = np.array([10, 20, 30, 40])
np.random.permutation(x) # 生产新列表
array([20, 40, 10, 30])
print(x)
np.random.shuffle(x) # 修改原列表
print(x)
[10 20 30 40]
[20 40 10 30]
(12)随机采样
- 按指定形状采样
x = np.arange(10, 25, dtype = float)
x
array([10., 11., 12., 13., 14., 15., 16., 17., 18., 19., 20., 21., 22.,
23., 24.])
np.random.choice(x, size=(4, 3))
array([[19., 23., 22.],
[22., 21., 13.],
[15., 21., 17.],
[14., 23., 19.]])
import numpy as np
np.random.choice(10, 10)
array([0, 0, 9, 5, 8, 5, 2, 4, 9, 8])
x = np.arange(5).reshape(1, 5)
print(x)
print(np.arange(5))
x.sum(axis=1, keepdims=True)
[[0 1 2 3 4]]
[0 1 2 3 4]
array([[10]])
- 按概率采样
np.random.choice(x, size=(4, 3), p=x/np.sum(x))
array([[15., 21., 20.],
[23., 17., 18.],
[23., 15., 17.],
[19., 24., 22.]])
11.3 Numpy数组的性质
11.3.1 数组的属性
- 数组的形状shape
- 数组的维度ndim
- 数组的大小size
- 数组的数据类型dtype
x = np.random.randint(10, size=(3, 4))
print(x)
print('type:', type(x))
print('x.ndim:', x.ndim)
print('x.shape:', x.shape)
print('x.size:', x.size)
print()
y = np.arange(10)
print(y)
print('type:', type(y))
print('y.dim:', y.ndim)
print('y.shape:', y.shape)
print('y.size:', y.size)
[[4 3 1 6]
[8 1 8 6]
[3 6 9 6]]
type: <class 'numpy.ndarray'>
x.ndim: 2
x.shape: (3, 4)
x.size: 12
x.dtype: int32
[0 1 2 3 4 5 6 7 8 9]
type: <class 'numpy.ndarray'>
y.dim: 1
y.shape: (10,)
y.size: 10
y.dtype: int32
11.3.2 数组索引
1、一维数组的索引
x1 = np.arange(10)
x1
array([0, 1, 2, 3, 4, 5, 6, 7, 8, 9])
x1[0]
0
x1[5]