引言:
最近开始学习Person ReID(行人重识别)有关的内容,于是便读了几篇2018CVPR论文。来记录学习的经历。如果有讲得不到位或者您有什么想要补充的话,欢迎留言哦~
接下里将列举3篇论文:
- Pose Transferrable Person Re-Identification
- Deep Spatial Feature Reconstruction for Partial Person Re-identification: Alignment-free Approach
- Adversarially Occluded Samples for Person Re-identification
使用的数据集介绍
Market1501: It consists
of 12,936 images for training, and each person has
17.2 images on average in the train set.
DukeMTMC-reID: a subset of the DukeMTMC for image-based ReID.
Its train set contains 16522 images of 702 identities.
CUHK03: contains 14,096 images of 1,467 identities which are captured from two cameras in the CUHK campus.
MARS: a large video-based person ReID dataset. It consists of 20,478 tracklets and 1,191,003 bounding boxes of 1,261 identities and contains rich pose variations.
Partial-REID: a specially designed partial
person dataset that includes 600 images from 60 people,
with 5 full-body images and 5 partial images per person.
Partial-iLIDs: contains a total of 476 images of 119 people captured
by multiple non-overlapping cameras. Some images
in the dataset contain people occluded by other individuals
or luggages.
CVRP2018
1. Pose Transferrable Person Re-Identification
a) Methods strategy:
Data Augumention
b) 实施细节:
首先在目标数据集上训练一个Re-ID model.
然后使用上面pre-train model作为一个guider,来指导GAN对skeleton-to-image的训练,其目的是生成更多的姿态.
最后将上面生成的结果来产生更多的labeled pose-varied samples,通过这种数据增强的方法来增强Re-ID的训练model.
c) 效果展示:
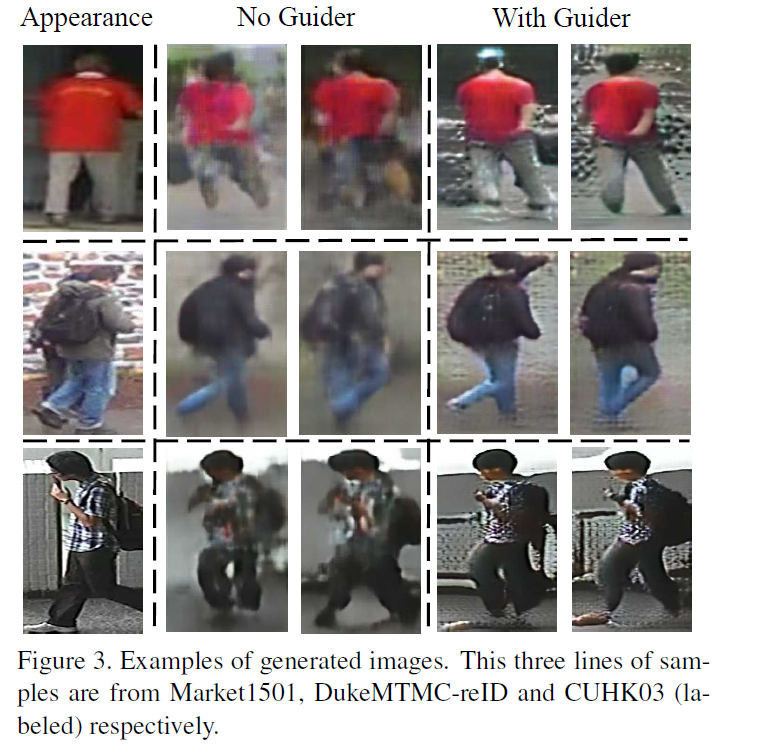

可以看出,加入guider指导后,GAN生成的图片具有更多的辨识信息,对于模型训练帮助会更好。
d) 实验结果:
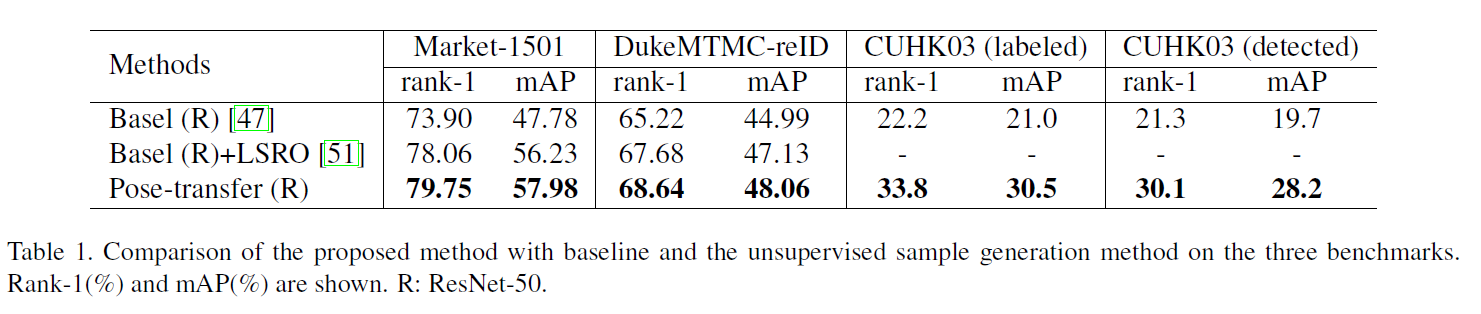
可以看到,在多个数据集上都有提升。
e) 优点:
该文的方法不仅可以增强基于feature learning,而且也能增强基于metric
learning的Re-ID model性能
2. Deep Spatial Feature Reconstruction for Partial Person Re-identification: Alignment-free Approach
a) Methods strategy:
deep feature learning method
b) 采用的方法:
Fully Convolutional Network
该方法可以解除输入尺寸必须限定的约束(因为没有全连接层)
且能保留spatial coordinate information
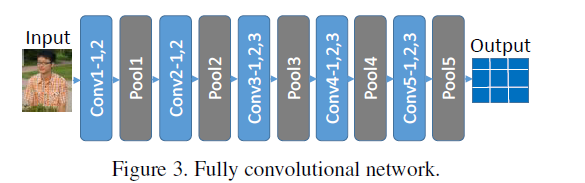
Deep Spatial Feature Reconstruction
主要用于衡量一个人不同尺寸图片之间的相似度
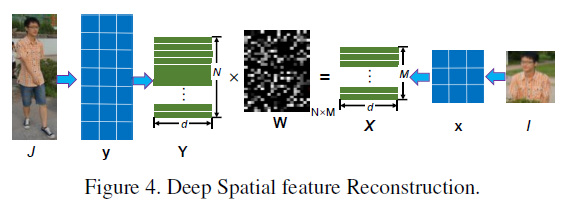
Multiscale Block Representation
Figure 4采用的singleScale-blocks 鲁棒性不够,因此采用MutiScale-blocks来提升性能
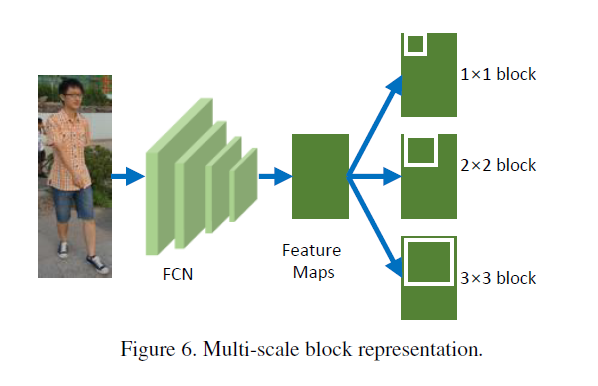
c) 结果分析:
可以看出,相比于直接暴力Resize图片而言,DSR采用的方法性能大大优于前者
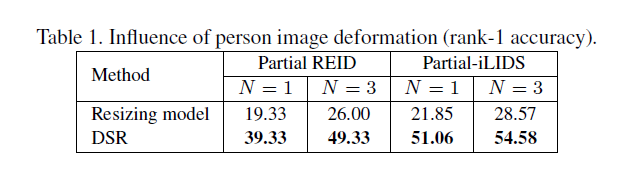
相比于其他算法
,提升效果明显
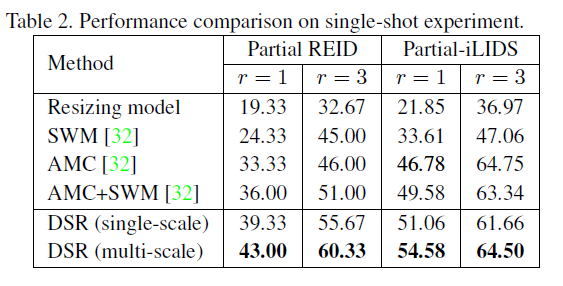
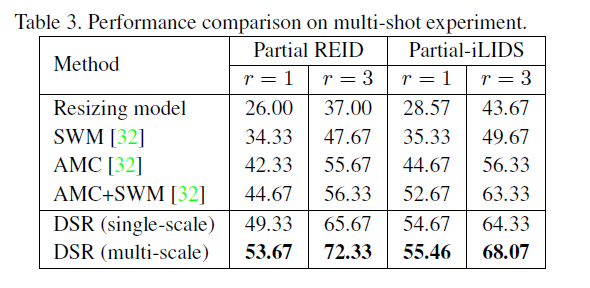
d) 优点:
为了摆脱固定的输入尺寸,本文提出的空间特征重构方法提供了一种可行的方案,该方法将探测空间特征图中的每个通道由空间图像映射的通道线性重构,同时避免了繁琐的无对齐匹配。此外,我们将DSR嵌入到FCN中,以学习更多的鉴别特征,从而使来自同一个人的图像对的重构误差最小
3. Adversarially Occluded Samples for Person Re-identification
a) Methods strategy:
Data Augumention
b) 采用的方法:
首先训练一个Re-ID model直到收敛。
然后利用网络可视化工具去探索哪些region是用来做最终决策的。
最后对这些region采用全黑Mask部分遮挡或者加入真实世界场景的noise重新作为训练集,re-train model。
c) 思路来源:
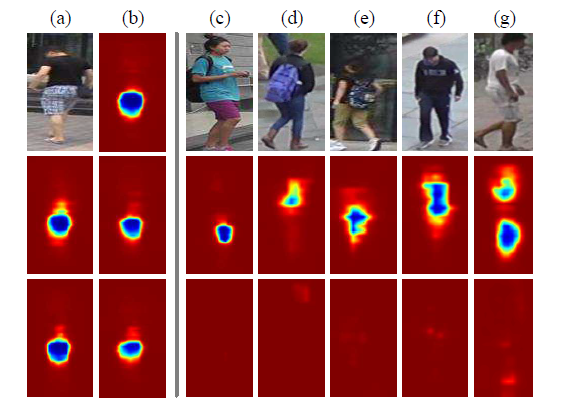
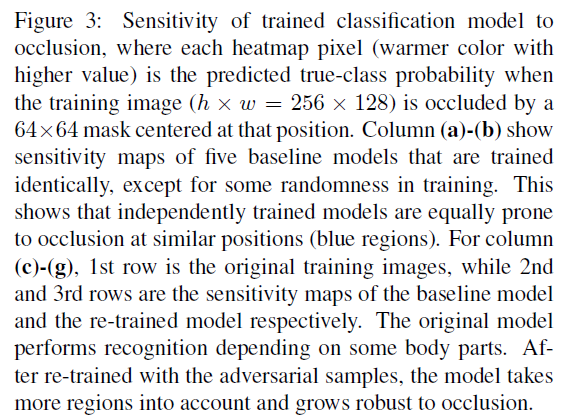
左列中(a)(b)中5张heatmap是采用不同的baseline,但对其可视化结果可以看出大致相同。因此网络在进行决策时,主要是依赖的图像特征是相近的。所以为了对数据进行有效地增强,可以考虑在这些起决策作用的region进行遮掩或者添加噪声,来获取更好的泛化能力。
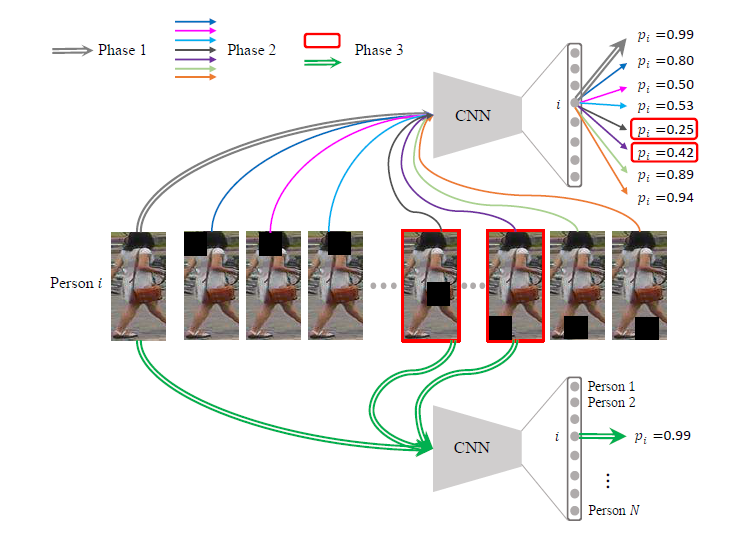
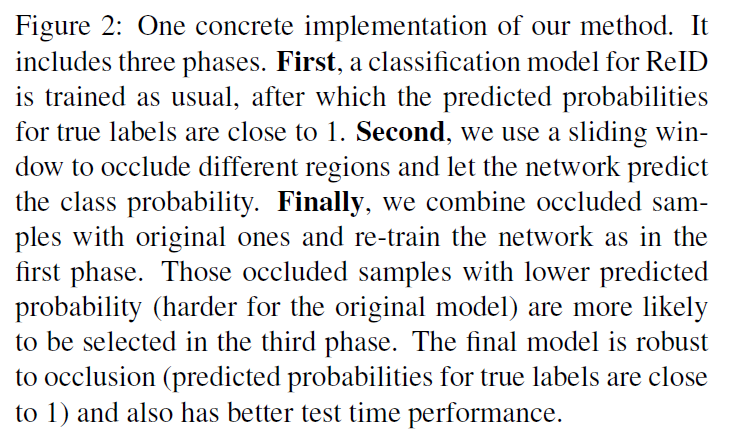
由于不同图片的决策region是不相同的,因此可以本文采用上图的方法去寻找对决策起比较大负面影响的Mask位置来做为对抗训练的样本。
d) 实验结果:
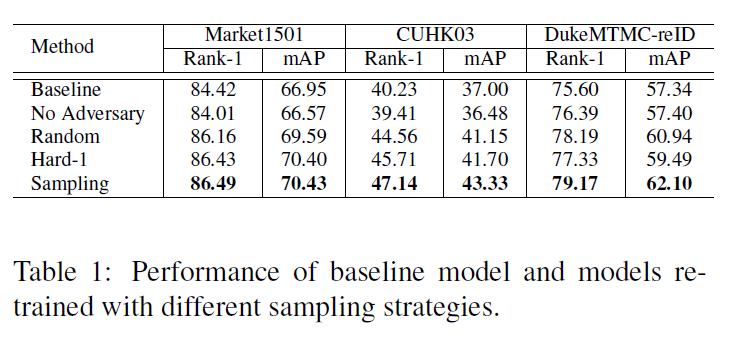
可以看出本文所采用的的方法,无论是Hard-1策略还是Sampling策略,在前两个数据集上都能产生积极效果,然后在第三个数据集上,Hard-1策略表现不如Random。
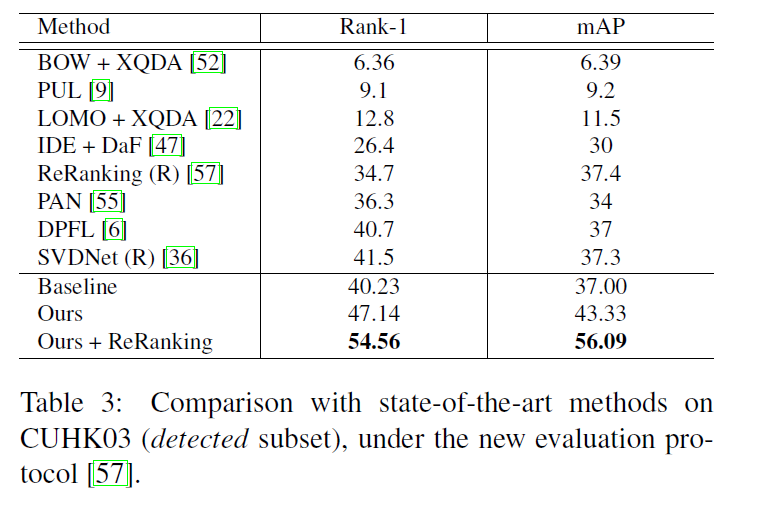
此外,作者还发现,本文提出的方法+ReRanking可以大大提高模型的性能。
e) 优点:
由于本文采用的是Data Augumention,因此可以与其他模型进行融合。
如果觉得我有地方讲的不好的或者有错误的欢迎给我留言,谢谢大家阅读(点个赞我可是会很开心的哦)~