http://www.cnblogs.com/xiaomalv2/archive/2012/01/06/2314872.html
基於像素的匹配
1、歸一化積相關灰度匹配:
模板圖像 以窗口滾動的方式 在源圖像中 掃一遍。
具體運算公式如下:
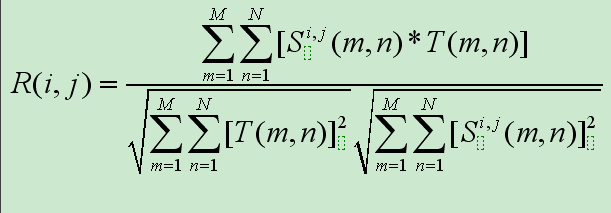
R(i,j) = dSigmaST / (dSigmaT * dSigmaS) 對應上公式; R(i,j)=[0,1]
M ,N 模板大小
對於公式的解釋:
dSimgmaST -- 在 在原圖(i ,j) 位置 模板圖像每個像素與對應原圖像素的積 的和)
dSigmaT -- 模板圖像每個像素的積 的和
dSigmaS -- 在原圖(i,j)位置,模板圖像對應的原圖的每個像素的積的和
R(i,j)最大的位置就是最匹配的位置。
/*
函數:NormalizeGrayMatch
功能:歸一化灰度值匹配
參數:src 原圖
template 模板
point 匹配的位置左上角
返回值: 0 --- 未找打匹配對象
其他 --- 返回匹配位置相似度
*/
double NormalizeGrayMatch(IplImage * src , IplImage * temp , CvPoint & point)
{
int nSrcWidth = src->width ;
int nSrcHeight = src->height;
int nTwidth = temp->width ;
int nTheight = temp->height ;
//計算模板像素灰度值 dSigmaT
double dSigmaT = 0 ;
unsigned char piexl = 0 ;
for (int i = 0 ; i < nTheight ; ++ i)
{
for(int j = 0 ; j< nTwidth ; ++j)
{
piexl = *(temp->imageData + i * temp->widthStep + j ) ;
dSigmaT += (double) piexl * piexl ;
}
}
double R = 0 ;
double dSigmaS ;
double dSigmaST ;
unsigned char piexlT,piexlS;
double dMaxR = -1 ;
int nMaxHeight = -1 ;
int nMaxWidht = -1 ;
for (int i = 0 ; i < nSrcHeight - nTheight +1 ; ++ i )
{
for (int j = 0 ; j < nSrcWidth - nTwidth + 1 ; ++ j)
{
dSigmaST = 0 ;
dSigmaS = 0 ;
// 計算dSigmaST dSigmaS
for (int k = 0 ; k < nTheight ; ++ k)
{
for (int l = 0 ; l < nTwidth ; ++ l)
{
//模板像素
piexlT =*( temp->imageData + k * temp->widthStep + l );
//源圖像像素
piexlS = *(src->imageData + (k + i ) * src->widthStep + (l + j) ) ;
dSigmaST += (double) piexlS * piexlT ;
dSigmaS += (double) piexlS * piexlS ;
}
}
R = dSigmaST / ( sqrt(dSigmaS) * sqrt(dSigmaT) ) ;
if (R > dMaxR )
{
nMaxWidht = j;
nMaxHeight = i ;
dMaxR=R ;
}
}
}
if (dMaxR != -1)
{
point .x = nMaxWidht ;
point .y = nMaxHeight;
return dMaxR ;
}
return 0 ;
}
2. 序貫相似性檢測法匹配 SSDA -- Similarity Sequential Dectection Algorithm
前面的歸一化積相關匹配算法計算量很大,原因在於搜索窗口在源圖像上進行滑動,每滑動一次就要做一次匹配運算,除了匹配的點外在其他匹配點做了『無用功』,導
致了匹配算法的計算量上升。所以,一旦發現所在的參考位置為非匹配點,就丟棄不再計算,立刻換到新的參考點計算,可以大大加速匹配過程。
SSDA算法過程:
1.定義絕對誤差:

其中:
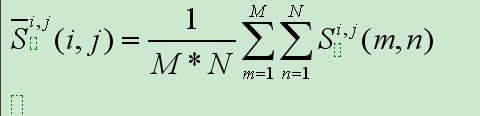
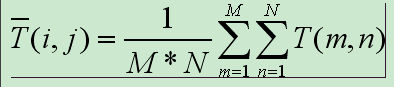
2.取一個不變閾值
3.掃面原圖每個像素點待匹配點對應的模板子圖,根據1中的公式據算絕對誤差,當累加值超過閾值時停止累加,停止此模板子圖的掃描,記錄帶匹配點的位置和累加次數
4,循環 3 直到掃描完全圖
5,累加次數最少的像素點就為最佳匹配點
對於序貫相似相算法有很多可以優化的地方, 比如 第三步 掃描子圖像素的時候 可以用 隔行 列 掃描 , 第二部 閾值可以改為自動閾值等等,不過這裡的優化有能
怎麼樣呢 還是很慢呀,還是期待後面的算法吧。
/*
函數:SSDAMatch
參數:src --- 源圖像 in
temp -- 匹配模板 in
point -- 匹配位置 out
threshold -- 閾值 in
返回值:累加次數
限制: 8位灰度圖
*/
int SSDAMatch(IplImage * src , IplImage * temp , CvPoint & point , int threshold)
{
int nSrcWidth = src->width ;
int nSrcHeight = src->height;
int nTwidth = temp->width ;
int nTheight = temp->height ;
int ntempsize = nTwidth * nTheight ; //模板大小
//計算模板像素灰度值 dSigmaT
double dSigmaT = 0 ;
unsigned char piexl = 0 ;
for (int i = 0 ; i < nTheight ; ++ i)
{
for(int j = 0 ; j< nTwidth ; ++j)
{
piexl = *(temp->imageData + i * temp->widthStep + j ) ;
dSigmaT += (double) piexl ;
}
}
dSigmaT /=ntempsize ;
double dSigmaS = 0 ;
double dbr =0; //誤差
long lr = 0; //誤差累積次數
long maxR =0; //最大累積次數
int nMaxHeight = 0 ; //最大累計次數 對應匹配位置 (左上角)
int nMaxWidht = 0 ;
for (int i = 0 ; i < nSrcHeight - nTheight +1 ; ++i)
{
for (int j = 0 ; j < nSrcWidth - nTwidth + 1 ; ++ j)
{
//計算dSigmaS
dSigmaS = 0 ;
dbr = 0 ;
lr = 0 ;
for (int k = 0 ; k < nTheight ; ++k)
{
for(int l = 0 ; l < nTwidth ; ++l)
{
dSigmaS +=(unsigned char ) *(src->imageData + (k +i) * src->widthStep + (l + j)) ;
}
}
dSigmaS /=ntempsize ;
//計算誤差 一旦超過閾值則拋棄不再計算
for (int k = 0 ; k < nTheight ; ++ k)
{
for (int l = 0 ; l < nTwidth ; ++l)
{
dbr += abs( (unsigned char )*(src->imageData + (k+i) * src->widthStep + (l+j) )-dSigmaS -
(unsigned char )*(temp->imageData + k * temp->widthStep + l) +dSigmaT );
lr ++ ;
if (dbr >= threshold)
break;
}
if (dbr>=threshold)
break;
}
//取達到threshold 累加最多的 位置
if ( lr >maxR )
{
maxR = lr ;
nMaxHeight = i;
nMaxWidht = j ;
}
}
}
point.x = nMaxWidht ;
point.y = nMaxHeight ;
return lr;
}
基於特征的匹配
利用灰度信息匹配的方法主要缺陷是計算量過大,對圖像的灰度變換很敏感,尤其是非線性的光照變化,此外,對目標的旋轉、變形以及遮擋也比較敏感,為
了克服這些缺點,可以利用圖像的特征進行匹配,由於圖像的特征點比像素點要少很多,大大減少了匹配過程的計算量,同時,特征點的匹配度量值對位置的
變化比較敏感,可以大大的提高匹配的精度。而且,特征點的提取過程可以減少噪聲的影響,對灰度變化、圖像形變以及遮擋等有較好的適應能力。
3. 不變矩匹配法 TM算法 具有平移、旋轉、尺寸不變性
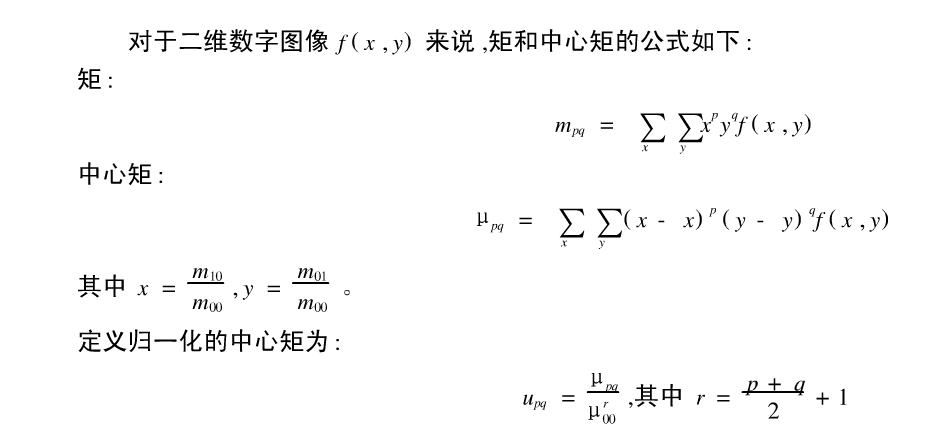
p+q>=2
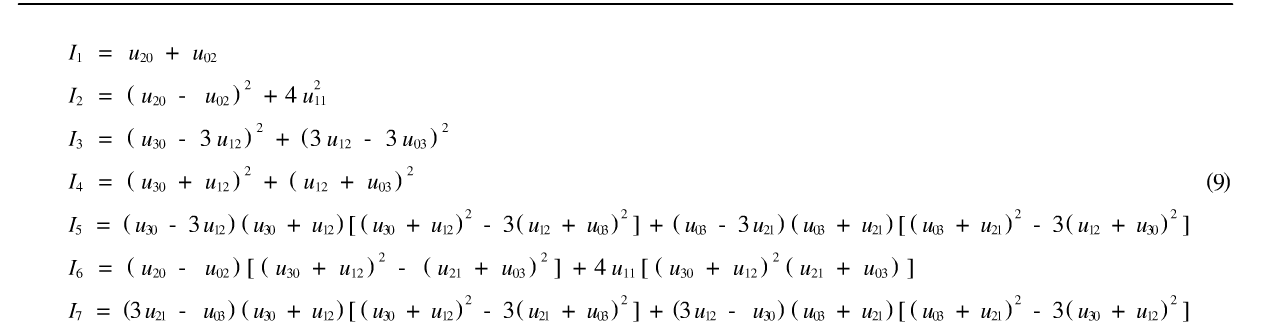
歸一化公式:
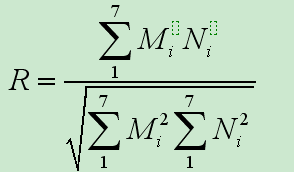
算法過程:計算 分別計算模板和原圖的7個不變矩 ,根據歸一化公式得出相似度。
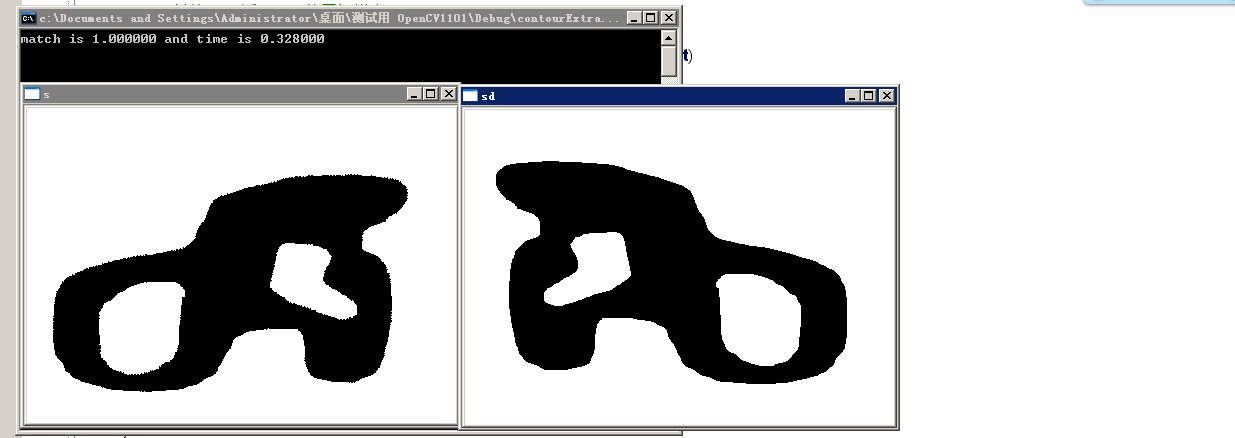
double momentMatch(unsigned char * src , unsigned char * temp ,int nwidth , int nheight ,int nwidthstep )
{
//原圖和模板重心矩
int nSBarycenterX , nSBarycenterY;
int nTBasrycenterX,nTBarycenterY;
CalBarycenter(src , nwidth , nheight , nwidthstep ,nSBarycenterX , nSBarycenterY ) ;
CalBarycenter(temp , nwidth , nheight ,nwidthstep , nTBasrycenterX , nTBarycenterY);
//原圖和模板二階三階規格化中心矩
double Su00 ,Su02 ,Su20 ,Su11 ,Su30 ,Su12 ,Su21 ,Su03;
double Tu00 ,Tu02 ,Tu20 ,Tu11 ,Tu30 ,Tu12 ,Tu21 ,Tu03;
Su00 = CalCenterMoment(src , nwidth ,nheight ,nwidthstep ,nSBarycenterX,nSBarycenterY,0,0) ;
Su02 = CalCenterMoment(src , nwidth ,nheight , nwidthstep , nSBarycenterX,nSBarycenterY,0,2)/pow(Su00 , 2);
Su20 = CalCenterMoment(src , nwidth , nheight , nwidthstep , nSBarycenterX,nSBarycenterY,2,0)/pow(Su00 , 2);
Su11 = CalCenterMoment(src , nwidth , nheight , nwidthstep , nSBarycenterX,nSBarycenterY,1,1)/pow(Su00,2);
Su30 = CalCenterMoment(src , nwidth , nheight ,nwidthstep , nSBarycenterX,nSBarycenterY ,3 ,0 )/pow(Su00 , 2.5);
Su12 = CalCenterMoment(src , nwidth , nheight , nwidthstep , nSBarycenterX,nSBarycenterY ,1 ,2 )/pow(Su00 , 2.5);
Su21 = CalCenterMoment(src , nwidth , nheight , nwidthstep , nSBarycenterX,nSBarycenterY , 2 ,1 )/pow(Su00 , 2.5);
Su03 = CalCenterMoment(src , nwidth , nheight , nwidthstep , nSBarycenterX,nSBarycenterY ,0,3)/pow(Su00 , 2.5);
Tu00 = CalCenterMoment(temp , nwidth ,nheight ,nwidthstep ,nTBasrycenterX , nTBarycenterY,0,0) ;
Tu02 = CalCenterMoment(temp , nwidth ,nheight , nwidthstep , nTBasrycenterX , nTBarycenterY,0,2)/pow(Su00 , 2);
Tu20 = CalCenterMoment(temp , nwidth , nheight , nwidthstep , nTBasrycenterX , nTBarycenterY,2,0)/pow(Su00 , 2);
Tu11 = CalCenterMoment(temp , nwidth , nheight , nwidthstep , nTBasrycenterX , nTBarycenterY,1,1)/pow(Su00,2);
Tu30 = CalCenterMoment(temp , nwidth , nheight ,nwidthstep , nTBasrycenterX , nTBarycenterY ,3 ,0 )/pow(Su00 , 2.5);
Tu12 = CalCenterMoment(temp , nwidth , nheight , nwidthstep , nTBasrycenterX , nTBarycenterY ,1 ,2 )/pow(Su00 , 2.5);
Tu21 = CalCenterMoment(temp , nwidth , nheight , nwidthstep , nTBasrycenterX , nTBarycenterY , 2 ,1 )/pow(Su00 , 2.5);
Tu03 = CalCenterMoment(temp , nwidth , nheight , nwidthstep , nTBasrycenterX , nTBarycenterY,0,3)/pow(Su00 , 2.5);
//原圖和模板不變矩
double Sa[7] , Ta[7];
Sa[0] = Su02 + Su20 ;
Sa[1] = pow(Su20 - Su02 , 2) + 4 * pow(Su11 , 2 );
Sa[2] = pow(Su30 - 3*Su12 , 2) + pow(3 * Su12 -Su03 ,2);
Sa[3] = pow(Su30+Su12 , 2) + pow(Su21 + Su03 ,2);
Sa[4] = (Su30 -3*Su12) * (Su30 + Su12 ) * (pow(Su30 + Su12 ,2) - 3*pow(Su21 + Su03 ,2)) +
(3 * Su21 -Su03) *(Su21 + Su03) *(3* pow(Su03 + Su12 , 2) - pow(Su21 + Su03 ,2));
Sa[5] = (Su20 - Su02)*(pow(Su30 +Su12 ,2) - pow(Su21 + Su03 ,2)) + 4*Su11 *(Su30 + Su12)*(Su21 +Su03);
Sa[6] = (3*Su21 - Su03) *(Su30 + Su12) *(pow(Su30 + Su12 ,2) - 3 *pow(Su21 + Su03 ,2)) + (Su30 -3*Su12) *(Su21 +Su03) *
(3 *pow(Su30 + Su12 ,2) - pow(Su21 + Su03 ,2));
Ta[0] = Tu02 + Tu20 ;
Ta[1] = pow(Tu20 - Tu02 , 2) + 4 * pow(Tu11 , 2 );
Ta[2] = pow(Tu30 - 3*Tu12 , 2) + pow(3 * Tu12 -Tu03 ,2);
Ta[3] = pow(Tu30+Tu12 , 2) + pow(Tu21 + Tu03 ,2);
Ta[4] = (Tu30 -3*Tu12) * (Tu30 + Tu12 ) * (pow(Tu30 + Su12 ,2) - 3*pow(Tu21 + Tu03 ,2)) +
(3 * Tu21 -Tu03) *(Tu21 + Tu03) *(3* pow(Tu03 + Tu12 , 2) - pow(Tu21 + Tu03 ,2));
Ta[5] = (Tu20 - Tu02)*(pow(Tu30 +Tu12 ,2) - pow(Tu21 + Tu03 ,2)) + 4*Tu11 *(Tu30 + Tu12)*(Tu21 +Tu03);
Ta[6] = (3*Tu21 - Tu03) *(Tu30 + Tu12) *(pow(Tu30 + Tu12 ,2) - 3 *pow(Tu21 + Tu03 ,2)) + (Tu30 -3*Tu12) *(Tu21 +Tu03) *
(3 *pow(Tu30 + Tu12 ,2) - pow(Tu21 + Tu03 ,2));
double r = 0;
double dSigmaST =0;
double dSigmaS = 0;
double dSigmaT = 0 ;
for (int i = 0 ; i < 7 ; ++i)
{
dSigmaST += Ta[i] * Sa[i] ;
dSigmaS +=pow(Sa[i] ,2);
dSigmaT +=pow(Ta[i] ,2);
}
return r = dSigmaST / sqrt( dSigmaS * dSigmaT) ;
}
/*
函數:CalBarycenter
功能:計算重心矩
參數:pdata -- 圖像數據 in
nwidth -- 寬 in
nheight -- 高 in
nwidthstep -- 步長 in
nBarycenterX -- 重心坐標 out
nBarycenterY
*/
void CalBarycenter(unsigned char * pdata , int nwidth , int nheight ,int nwidthstep , int &nBarycenterX , int &nBarycenterY)
{
double m00 , m01 ,m10;
m00 = 0 ;
m01 = 0 ;
m10 = 0 ;
for (int i = 0 ; i < nheight ; ++i)
{
for (int j = 0 ; j < nwidth ; ++ j)
{
m00 +=*(pdata + i * nwidthstep + j) ;
m01 +=*(pdata + i * nwidthstep + j) * j ;
m10 +=*(pdata + i * nwidthstep + j) * i ;
}
}
nBarycenterX =(int) (m10 / m00 +0.5);
nBarycenterY = (int)(m01/ m00 + 0.5);
}
/*
函數:CalCenterMoment
功能:計算中心矩
參數:pdata --- 圖像數據 in
nwidth -- 寬 in
nheight -- 高 in
nwidthstep -- 步長 in
nBarycenterX -- 重心矩 in
nBarycenterY
ip -- 階數 in
jq
返回值:中心距值
*/
double CalCenterMoment(unsigned char * pdata , int nwidth , int nheight ,int nwidthstep ,
double nBarycenterX , double nBarycenterY,int ip,int jq)
{
double Upq = 0 ;
for (int i = 0 ; i < nheight ; ++i)
{
for (int j=0; j <nwidth ; ++ j)
{
Upq +=*(pdata + i * nwidthstep + j) + pow(j -nBarycenterX , ip) * pow(i - nBarycenterY , jq) ;
}
}
return Upq ;
}
4.距離變換匹配法
距離變換是一種常見的二值圖像處理算法,用來計算圖像中任意位置到最近邊緣點的距離
歐幾米德空間距離:

其中a=(x1 , y1) b =(x2 ,y2)
設R 為二維圖像空間集合 S 為R中的邊緣點集合 R中任意一點 r,的距離變換為

如果該點是邊緣點則 距離就為0 ,如果不是邊緣點則找與之最近的邊緣點距離,就需要做最近鄰域搜索這樣計算量很大。
所以有一種近似方法作為替代 即查看點8鄰域內的情況如下表所示:
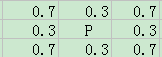
8鄰域內 P 到各個點的距離 , 如果8鄰域內找不到 邊緣點 則距離為1;
g(x) = 0 if=0
0.3 x=1
0.7 x=sqrt(2)
1 x>sqrt(2)
對於兩幅二值圖像A(模板圖),B(模板子圖) 的匹配誤差度量為:
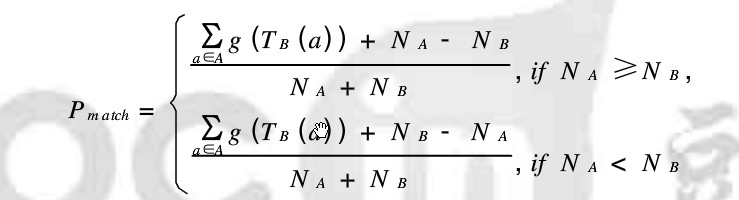
A,B 為圖像中的邊緣點集合。 a,b分別為A,B中的任意一點 Na Nb為A,B的點個數。
Pmatch = [0,1] ;這個公式表示:在模板對應圖中如果遍歷所有邊緣點,累加 邊緣點位置對應的模板圖的該點的距離變換。
可知如果兩個圖像完全一樣 則 Pmatch =0 ;
算法過程: 求模板圖的距離變換 , 模板圖邊緣點個數
在待匹配圖中 滑動窗口(大小為模板圖大小) ,在模板對應圖中如果遍歷所有邊緣點,累加 邊緣點位置對應的模板圖的該點的距離變換
dSigmaST , Pmatch = (dSigmaST + | Na - Nb | ) /(Na + Nb) ;
Pmatch最小時為最佳匹配點.
/*
函數:DisMatch
功能:距離變換匹配
參數:src -- 原圖 in
temp -- 模板圖 in
point -- 最佳匹配點 out
返回值:匹配誤差
*/
double DisMatch(IplImage * src,IplImage *temp,CvPoint &point )
{
int nSwidth = src->width ;
int nSheight =src->height;
int nSwidthstep = src->widthStep;
int nTwidth = temp->width;
int nTheight = temp->height;
int nTwidthstep = temp->widthStep;
unsigned char piexl;
//將模板圖像長寬加2 方便8鄰域計算
unsigned char * pTdata = (unsigned char *)malloc( (nTwidthstep+2 ) *(nTheight +2) );
memset(pTdata , 0, (nTwidthstep+2 ) *(nTheight +2) * sizeof(unsigned char));
for (int n = 1 ; n < nTheight +1; ++n)
memcpy( pTdata +n *(nTwidthstep +2)+1 , temp->imageData + n * nTwidthstep , nTwidthstep *sizeof(unsigned char) );
//計算模板距離變換
double * pTDist = (double *)malloc(nTwidth * nTheight * sizeof(double));
//模板T邊緣點個數
int Nb =0;
//8鄰域
unsigned char u11,u12,u13,u21,u23,u31,u32,u33;
for(int i = 1 ; i < nTheight +1 ; ++i)
{
for (int j = 1 ; j <nTwidth +1 ;++j)
{
piexl = *( pTdata + i * (nTwidthstep+2) +j);
//如果該點是邊緣點 dist=0;
if (piexl ==255)
{
pTDist[(i-1) *nTwidth + (j-1)] =0;
Nb++;
}
else
{
//否則看8鄰域 膨化加權
u11 = *(pTdata + (i-1)*(nTwidthstep+2)+j-1);
u12 = *(pTdata +(i-1)*(nTwidthstep+2)+j);
u13 =*(pTdata +(i-1)*(nTwidthstep+2)+j+1);
u21 =*(pTdata +i*(nTwidthstep+2)+j-1);
u23 =*(pTdata +i*(nTwidthstep+2)+j+1);
u31 =*(pTdata + (i+1)*(nTwidthstep+2)+j-1);
u32 =*(pTdata +(i+1)*(nTwidthstep+2)+j);
u33 =*(pTdata +(i+1)*(nTwidthstep+2)+j+1);
if (u12==255 ||u21==255 ||u23==255 ||u32==255)
pTDist[(i-1) *nTwidth + (j-1)] =0.3;
else if(u11==255 || u13==255 ||u31==255 ||u33==255)
pTDist[(i-1) *nTwidth + (j-1)] =0.7;
else
pTDist[(i-1) *nTwidth + (j-1)] =1;
}
}//for j
} //for i
//匹配
double dbMatch = 0 ; //匹配誤差
//最小匹配誤差
double dbMinMatch = 1;
double dSigmaST = 0 ;
//邊緣點個數
int Na = 0;
//最佳匹配點
int nMatchWidth , nMatchHeight;
for(int i = 0 ; i <nSheight -nTheight+1; ++i)
{
for (int j=0; j<nSwidth -nTwidth+1;++j)
{
dSigmaST = 0;
dbMatch = 0;
Na =0;
//模板滑動到(i,j)點對應的原圖
for (int k=0;k<nTheight ; ++k)
{
for (int l=0 ; l<nTwidth ;++l)
{
piexl = *(src->imageData + (i +k)*nSwidthstep +(j+l));
if (piexl==255)
{
dSigmaST +=pTDist[k *nTwidth +l];
Na++;
}
}//for l
}//for l
//計算匹配誤差
dbMatch = ( dSigmaST + abs(Na-Nb)) /(Na +Nb);
if (dbMatch < dbMinMatch)
{
dbMinMatch = dbMatch;
nMatchHeight = i ;
nMatchWidth = j;
}
} // for j
} //for i
point.x = nMatchWidth;
point.y = nMatchHeight;
free(pTDist);
free(pTdata);
return dbMinMatch;
}
5.最小均方誤差匹配法
最小均方誤差匹配方法是利用圖像中的對應
特征點,通過解特征點的變換防長來計算圖像間的變換參數。
基本原理:
最小均方誤差匹配方法是以模板中的特征點構造矩陣X ,圖像子圖中的特征點構造矩陣Y ,求解矩陣X 到矩陣Y 的變換矩陣, 其中誤差
最小的位置為最佳匹配位置
圖像間的仿變換方程:

原點為中心旋轉 平移
仿變換參數為
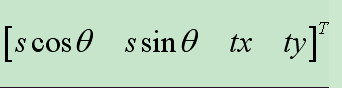
構建圖像矩陣 X
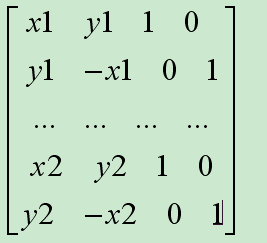
Y

最小均方誤差的原理是求解

其中
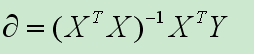