AutoEncoder
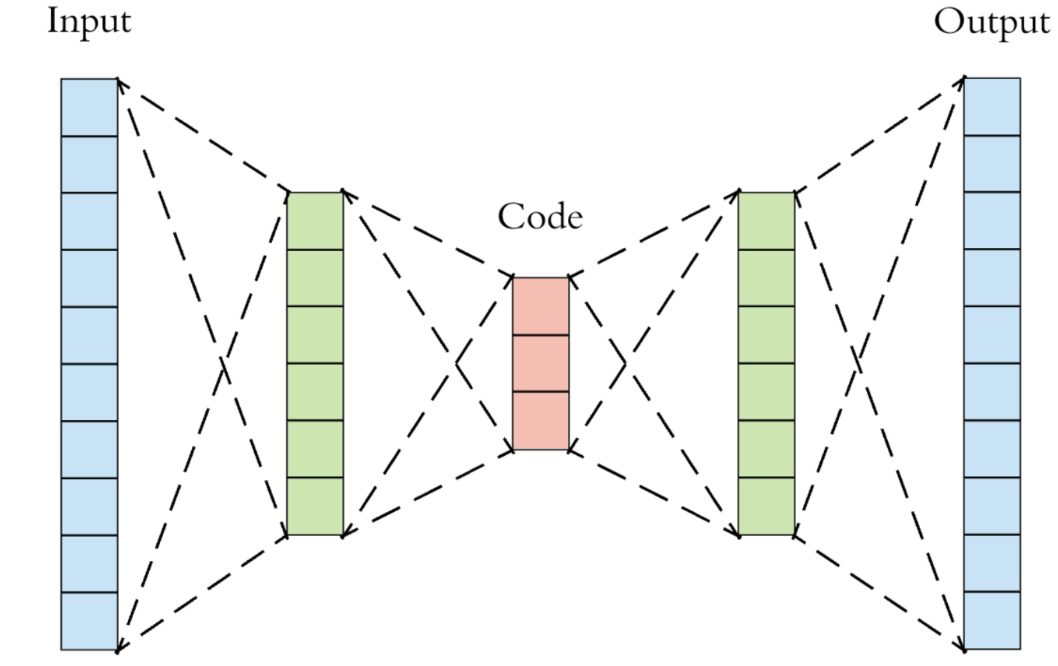
AutoEncoder(自编码器,AE)由两部分组成,左半部分称为编码器(Encoder),用函数h=f(x)h=f(x)h=f(x)表示,其中xxx代表输入,hhh代表中间隐藏层的编码输出;右半部分称为解码器(Decoder),用函数y=g(h)y=g(h)y=g(h)表示,其中yyy代表解码器的输出。
之所以叫做自编码器,是因为AE能够“自动地”对数据进行“编码”,或者说是“降维”,例如设损失函数:
L(x,g(f(x))),
L(x,g(f(x))),
L(x,g(f(x))),
LLL惩罚xxx与g(f(x))g(f(x))g(f(x))之间的误差(如均方误差),这样最小化LLL的训练过程就是希望AE的输入与输出相同。在这样的目的下,中间输出hhh就可以以更小的维度“表示”原始数据,也就实现了编码(降维)的过程。
当解码器是线性的,且LLL为均方误差,AE会学习出与PCA相同的生成子空间。
一个栗子
用AE对MNIST手写数字进行学习,AutoEncoder的结构如下:
AE(
(encoder): Sequential(
(0): Linear(in_features=784, out_features=128, bias=True)
(1): Tanh()
(2): Linear(in_features=128, out_features=64, bias=True)
(3): Tanh()
(4): Linear(in_features=64, out_features=12, bias=True)
(5): Tanh()
(6): Linear(in_features=12, out_features=3, bias=True)
)
(decoder): Sequential(
(0): Linear(in_features=3, out_features=12, bias=True)
(1): Tanh()
(2): Linear(in_features=12, out_features=64, bias=True)
(3): Tanh()
(4): Linear(in_features=64, out_features=128, bias=True)
(5): Tanh()
(6): Linear(in_features=128, out_features=784, bias=True)
(7): Sigmoid()
)
训练完后的输入和输出对比:
可以看到这个AE在隐藏层将输入数据(784维)压缩成了3维数据,损失了很多图像信息,甚至有些数字解码后变成了另一个数字(上图的“1”),这说明在实际应用时应当合理地选择编码尺度,不宜过大或者过小。
不过这里压缩成3维是为了方便可视化,这里取0,5,7三个数字的编码进行可视化: