AttGAN: Facial Attribute Editing by Only Changing What You Want(2017 CVPR)
文章简介:
本文研究面部属性编辑任务,其目的是通过操作单个或多个感兴趣的属性(如头发颜色、表情、胡须和年龄)来编辑面部图像。
- Dataset: CelebA
- 比较算法: FaderNetwork, VAE/GAM, IcGAN等
- 方法特点: 现有方法(如FaderNetwork)中有一些有attribute-independent约束,然而这个约束对于latent presentation的生成是一点"要求过度",最终可能会导致信息的丢失,导致生成效果不佳,本文是通过引入一个attribute classification constraint来使得更加精确地去修改属性。
- 效果初览:
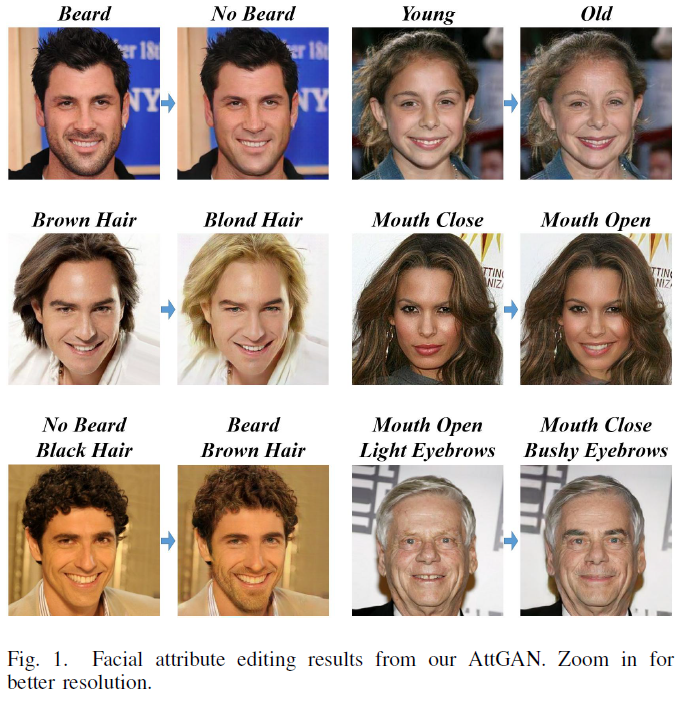
Contribution:
- 移除了严格的attribute-independent约束,仅需要通过attribute classification来保证正确地修改属性
- 整合了attribute classification constraint、reconstruction learning、adversarial learning,使得结果生成效果非常好
- 可以直接控制属性强度,从而可以自然地完成风格变换
Method Struct:
-
测试阶段
首先使用一个 G e n c G_{enc} Genc将 x a x^a xa转换为latent representations,其中 a a a是一个二值属性列表
z = G e n c ( x a ) z = G_{enc}(x^a) z=Genc(xa)
然后将目标属性 b b b加入进来,利用 G d e c G_dec Gdec进行decode
x b ^ = G d e c ( z , b ) x^{\hat{b}}=G_{dec}(z,b) xb^=Gdec(z,b)
上述结果的输出 x b ^ x^{\hat{b}} xb^就是我们想要生成的结果 -
训练阶段
训练的目标为:仅修改想要进行改变的attribute而对其他细节应该要保持不变- attribute classifier的目标就是在对 x b ^ x^{\hat{b}} xb^进行预测时,其预测结果标签应为 b b b。其中 b b b是一个二值列表。
- adversarial learning用于 x b ^ x^{\hat{b}} xb^是为了让生成结果更加逼真
- reconstruction learning的目标是令latent representations
z
z
z包含足够的信息,同时能令
G
d
e
c
G_{dec}
Gdec能够通过
z
z
z进行比较好的重建
x a ^ = G d e c ( z , a ) 并 且 x a ^ ≈ x a x^{\hat{a}}=G_{dec}(z, a) 并且x^{\hat{a}}\approx x^a xa^=Gdec(z,a)并且xa^≈xa
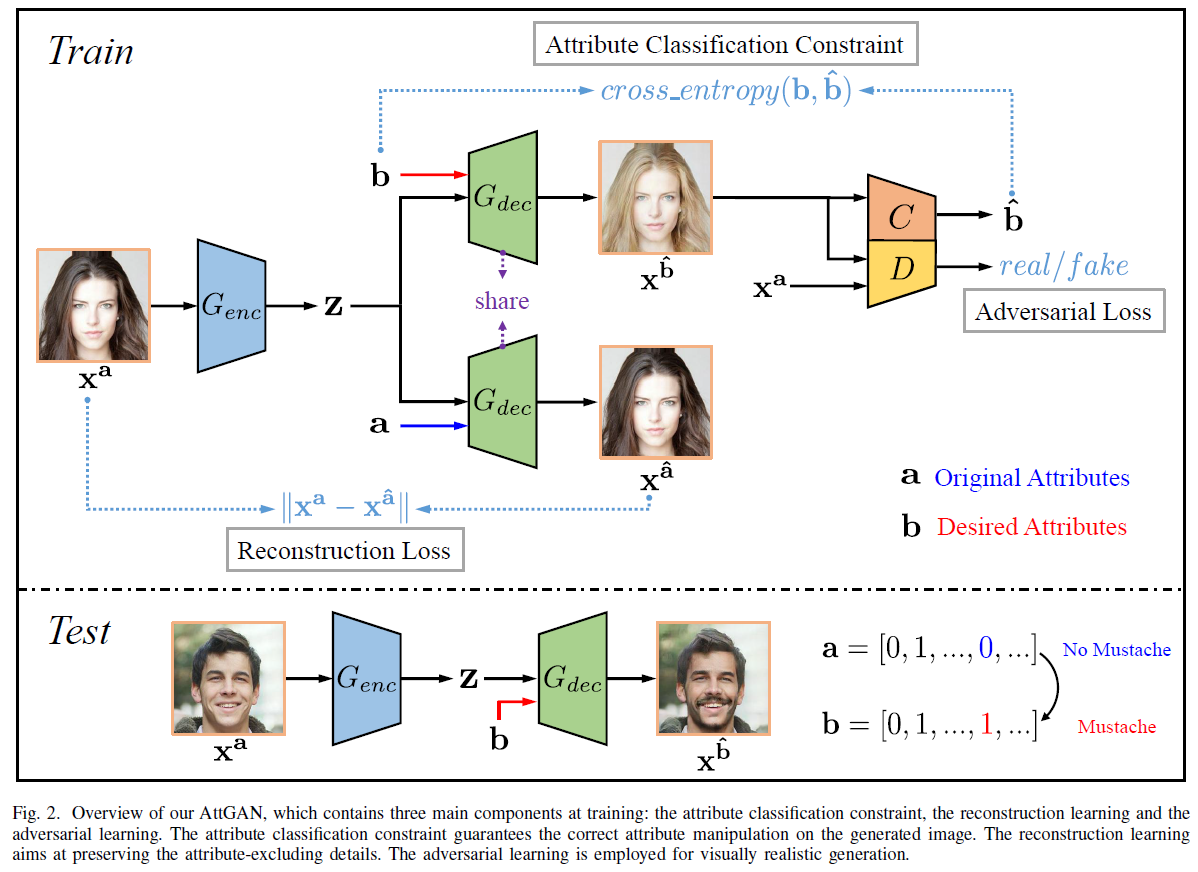
Method extension(style): 其生成模型可以表示为
x
θ
^
b
^
=
G
d
e
c
(
G
e
n
c
(
x
a
)
,
θ
,
b
)
x^{\hat{\theta}\hat{b}}= G_{dec}(G_{enc}(x^a), \theta, b)
xθ^b^=Gdec(Genc(xa),θ,b)
下图就是对Attribute Classification Constraint进行修改后的结构图,其中
Q
Q
Q是style predictor
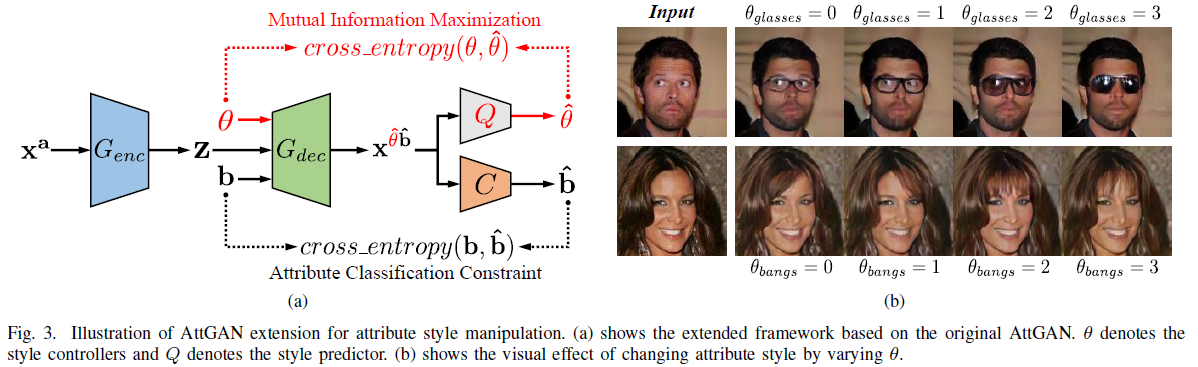
如果觉得我有地方讲的不好的或者有错误的欢迎给我留言,谢谢大家阅读(点个赞我可是会很开心的哦)~